Multi-scale Convolutional Neural Networks for Crowd Counting
2017 IEEE International Conference on Image Processing (ICIP)(2017)
摘要
Crowd counting on static images is a challenging problem due to scale variations. Recently deep neural networks have been shown to be effective in this task. However, existing neural-networks-based methods often use the multi-column or multi-network model to extract the scale-relevant features, which is more complicated for optimization and computation wasting. To this end, we propose a novel multi-scale convolutional neural network (MSCNN) for single image crowd counting. Based on the multi-scale blobs, the network is able to generate scale-relevant features for higher crowd counting performances in a single-column architecture, which is both accuracy and cost effective for practical applications. Complemental results show that our method outperforms the state-of-the-art methods on both accuracy and robustness with far less number of parameters.
更多查看译文
关键词
Multi-scale CNN,scale-relevant architectures,crowd counting
AI 理解论文
溯源树
样例
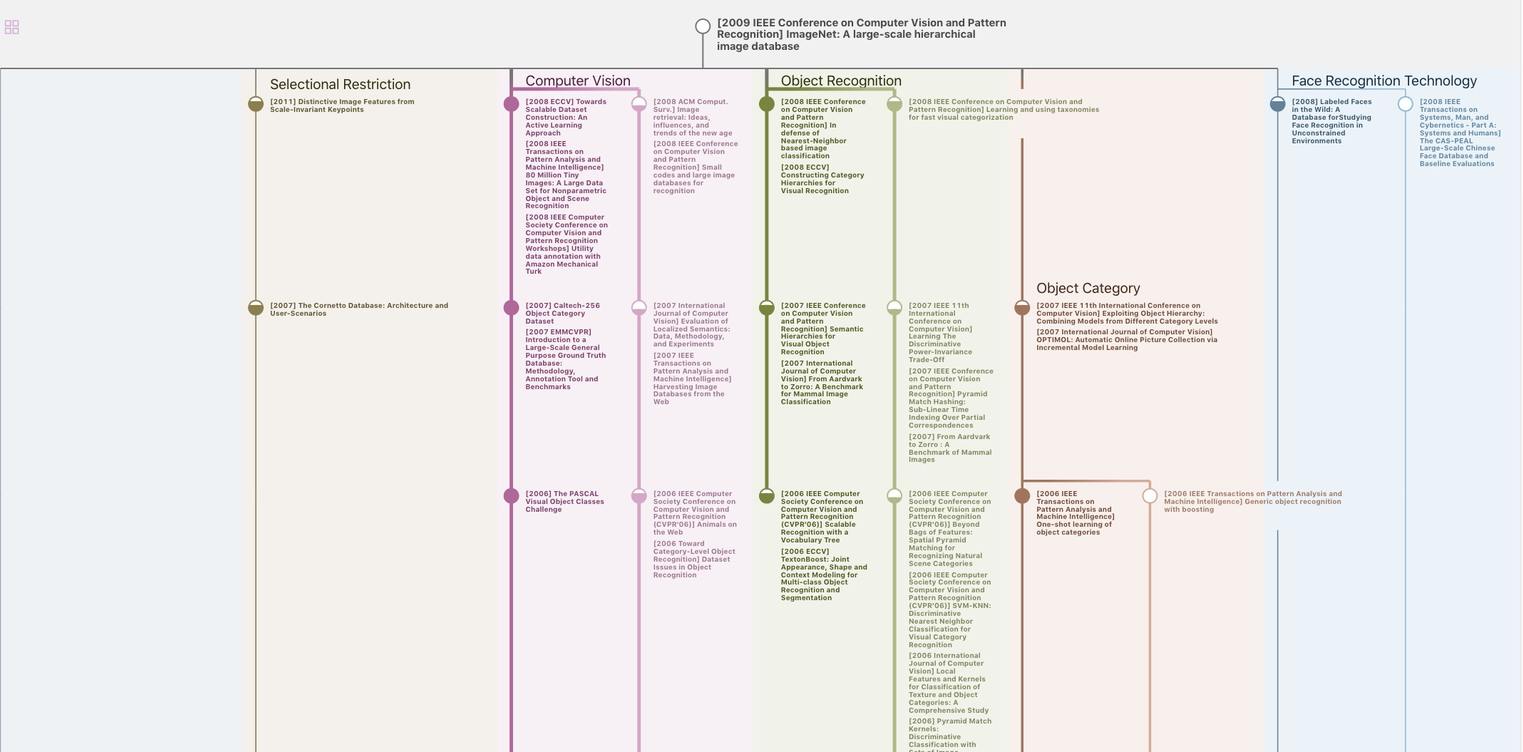
生成溯源树,研究论文发展脉络
Chat Paper
正在生成论文摘要