Approximate Near Neighbors for General Symmetric Norms
STOC '17: Symposium on Theory of Computing Montreal Canada June, 2017(2017)
摘要
We show that every symmetric normed space admits an efficient nearest neighbor search data structure with doubly-logarithmic approximation. Specifically, for every $n$, $d = n^{o(1)}$, and every $d$-dimensional symmetric norm $\|\cdot\|$, there exists a data structure for $\mathrm{poly}(\log \log n)$-approximate nearest neighbor search over $\|\cdot\|$ for $n$-point datasets achieving $n^{o(1)}$ query time and $n^{1+o(1)}$ space. The main technical ingredient of the algorithm is a low-distortion embedding of a symmetric norm into a low-dimensional iterated product of top-$k$ norms. We also show that our techniques cannot be extended to general norms.
更多查看译文
关键词
approximate near-neighbor,symmetric norms
AI 理解论文
溯源树
样例
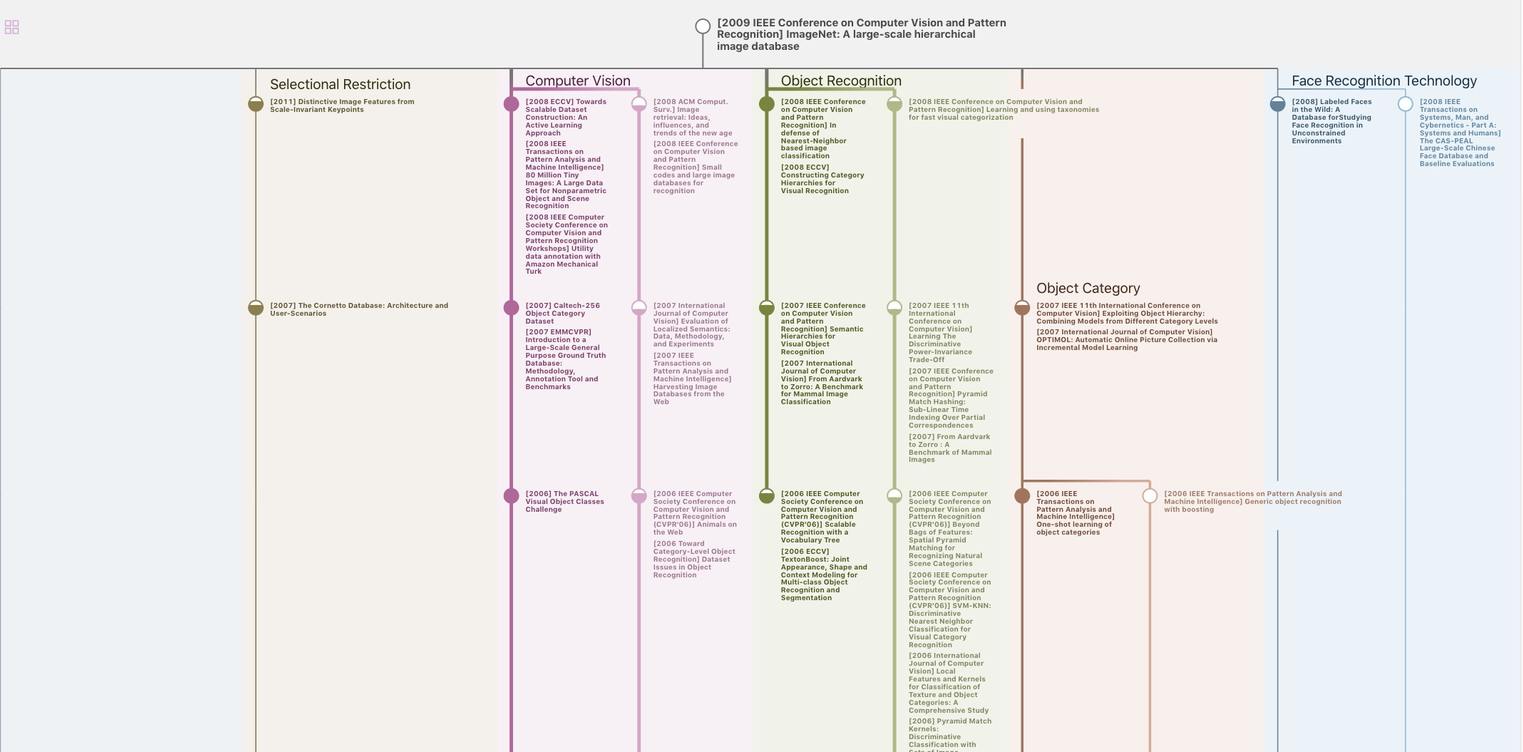
生成溯源树,研究论文发展脉络
Chat Paper
正在生成论文摘要