MiMSeg - an algorithm for automated detection of tumor tissue on NMR apparent diffusion coefficient maps.
Information Sciences(2017)
摘要
Although there are several data analysis frameworks, both commercial and open source, supporting the detection of tumours on nuclear magnetic resonance (NMR) sequences, none of them gives satisfactory results in the case of low volume tumors. The majority of the frameworks require the detailed analysis of at least two sequences of the examined sample, or give sample specific thresholds distinguishing between the tumor and subtypes of healthy tissue. In this paper, we present a novel algorithm for the automated estimation of tumor specific cut-off values in the domain of the apparent diffusion coefficient (ADC). Once the cut-off characteristics for a particular type of tumor is estimated, their further usage on other independent samples does not require any calculations except for an easy thresholding. The proposed methodology is a combination of classical decomposition of ADC distribution into a Gaussian mixture model (GMM) with k-means clustering subsequently performed on the parameters of mixture model components, leading to the identification of ADC distributions for every tissue type. The maximum conditional probability criterion gives the final threshold estimate. The developed signal analysis pipeline was applied to the problem of Glioblastoma Multiforme grade IV brain tumor segmentation, with a dataset of 119 randomly chosen ADC maps and Leave-One-Out cross-validation procedure for population error estimate. Additionally, a comparison to standard GMM based tumor segmentation algorithms as well as to three other automated segmentation methods was performed and the obtained tumor regions were referenced to the segmentation done by a human expert. The results demonstrate the average MiMSeg similarity to the expert-curated decision measured by the Dice coefficient as equal to 89.2% (with 95% confidence interval 87.7 ÷ 90.6). The MiMSeg algorithm significantly outperforms other techniques in the case of small tumors (of volume less than 10%), obtaining similarity to the expert-curated decision at the level 86.7%, with 44.9% obtained by standard GMM, 79.0% by Self-Organising-Maps algorithm, 68.7% by Murakami’s algorithm, and 78.2% by Kang’s method.
更多查看译文
关键词
Brain tumor segmentation,Diffusion weighted imaging,Gaussian mixture model,k-means clustering
AI 理解论文
溯源树
样例
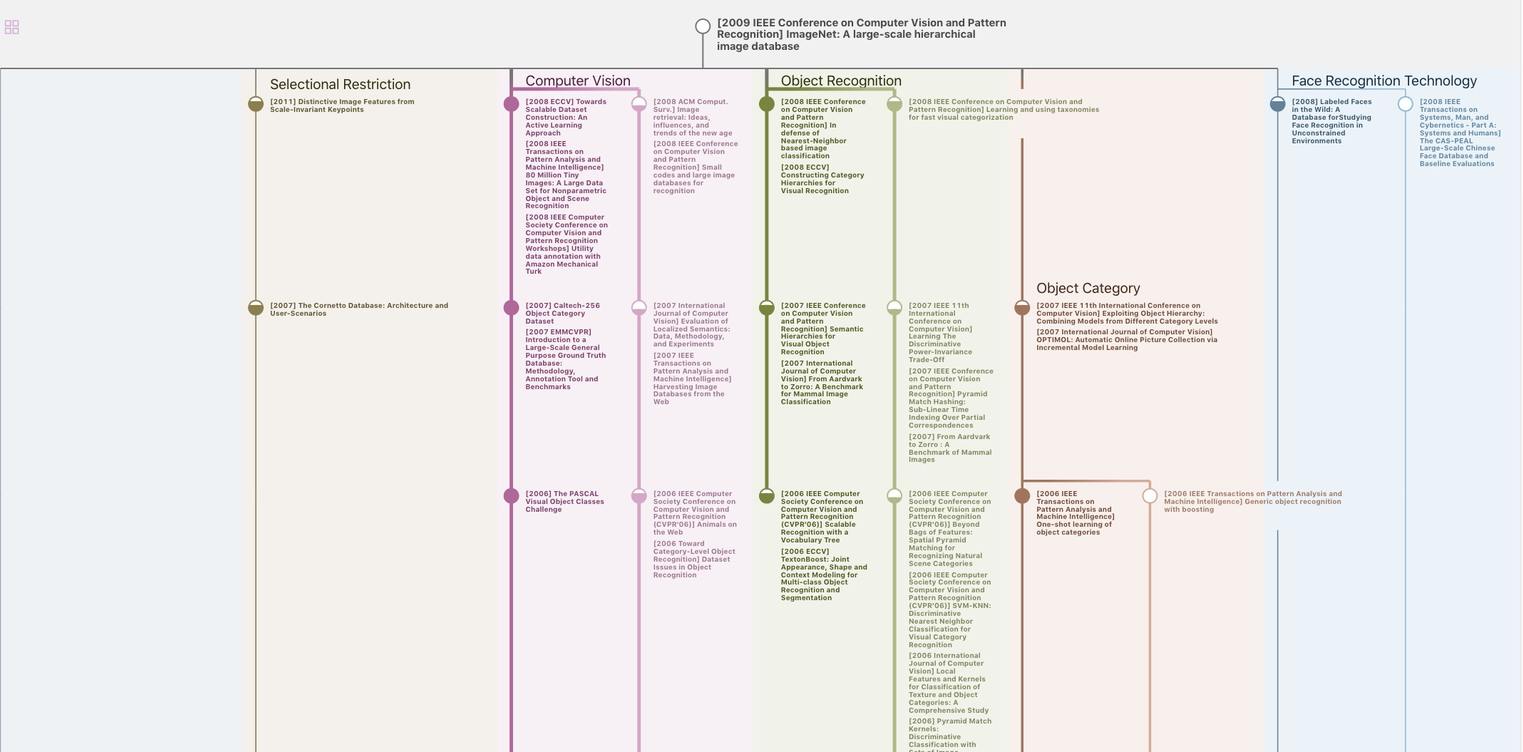
生成溯源树,研究论文发展脉络
Chat Paper
正在生成论文摘要