Improving surface normals based action recognition in depth images
2016 13th IEEE International Conference on Advanced Video and Signal Based Surveillance (AVSS)(2016)
摘要
In this paper, we propose a new local descriptor for action recognition in depth images. Our proposed descriptor jointly encodes the shape and motion cues using surface normals in 4D space of depth, time, spatial coordinates and higher-order partial derivatives of depth values along spatial coordinates. In a traditional Bag-of-words (BoW) approach, local descriptors extracted from a depth sequence are encoded to form a global representation of the sequence. In our approach, local descriptors are encoded using Sparse Coding (SC) and Fisher Vector (FV), which have been recently proven effective for action recognition. Action recognition is then simply performed using a linear SVM classifier. Our proposed action descriptor is evaluated on two public benchmark datasets, MSRAction3D and MSRGesture3D. The experimental result shows the effectiveness of the proposed method on both the datasets.
更多查看译文
关键词
surface normals,action recognition,depth images,local descriptor,4D space,spatial coordinates,higher-order partial derivatives,bag-of-words,BoW approach,depth sequence,sparse coding,Fisher vector,support vector machines,linear SVM classifier,MSRAction3D,MSRGesture3D
AI 理解论文
溯源树
样例
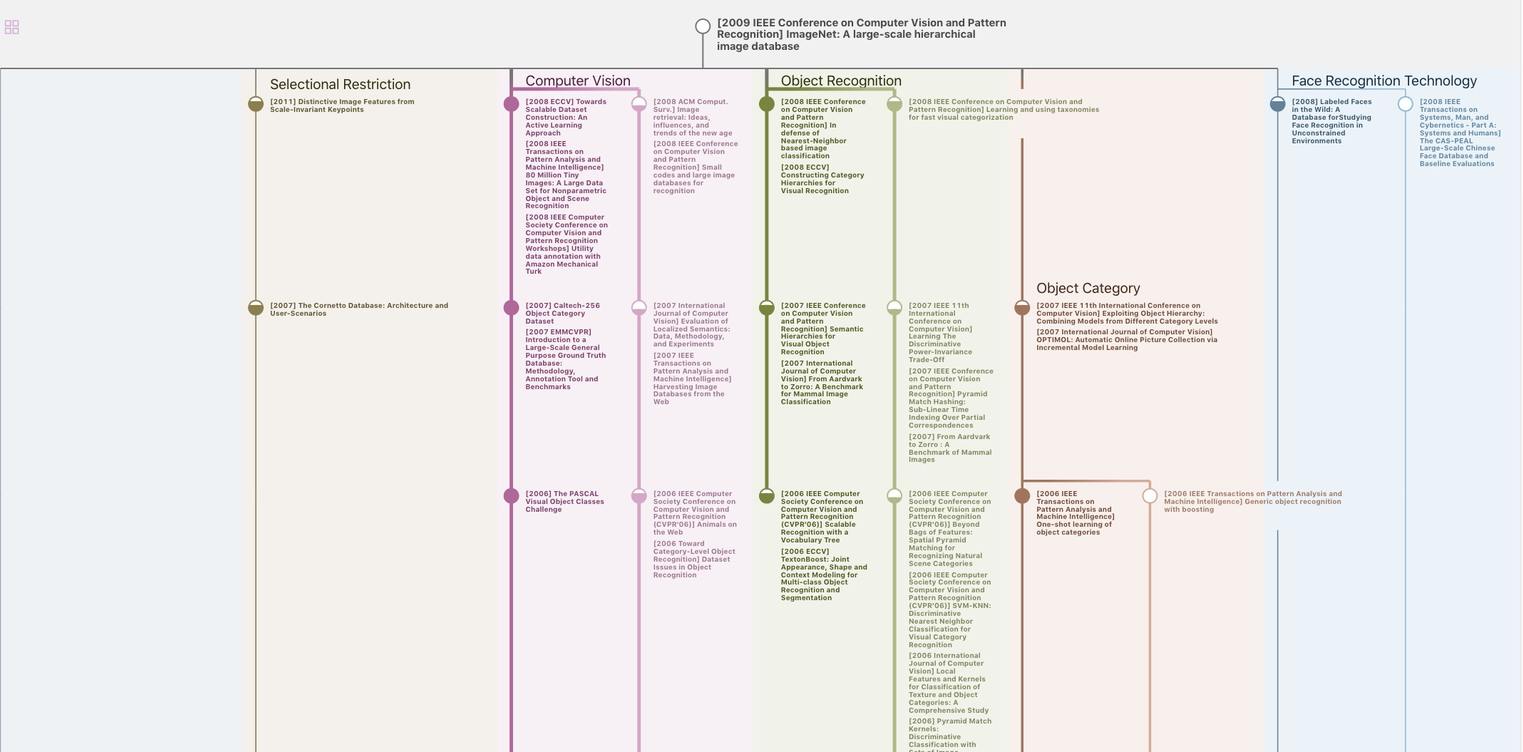
生成溯源树,研究论文发展脉络
Chat Paper
正在生成论文摘要