Sequential Appearance-Based Global Localization Using An Ensemble Of Knn-Dtw Classifiers
2016 International Joint Conference on Neural Networks (IJCNN)(2016)
摘要
The human Episodic Memory system stores sequences of events (episodes encoded in time and in space) experienced or imagined by an individual for later access to the episodes in whole or in part. Such ability provided by our Episodic Memory system is important, among other functions, for our localization in space throughout time. Inspired by that, in this paper, it is presented a new Sequential Appearance-Based approach to the Global Localization problem, dubbed SABGL. SABGL is based on an ensemble of kNN classifiers, where each classifier uses the Dynamic Time Warping (DTW) and the Hamming distance to compare binary features extracted from sequences of images. SABGL is designed to solve the global localization problem in two phases: mapping and localization. During mapping, it is trained with a sequence of images and associated locations that represents episodes experienced by an autonomous robot. During localization, it receives subsequences of images of the same environment and compares them to its previous experienced episodes, trying to recollect the most similar "experience" in time and space at once. Then, the system outputs the positions where it "believes" these images were captured. SABGL is compared with a previous single-image approach, named VibGL. Experimental results show that SABGL consistently outperforms VibGL in classification accuracy with higher precision in all maximum distance tolerance analyzed. For instance, given a maximum tolerance of 10m, SABGL is able to correctly localize an autonomous car 98% of the time.
更多查看译文
关键词
sequential appearance-based global localization,kNN-DTW classifiers,SABGL,Hamming distance,feature extraction,image sequences,classification accuracy,maximum distance tolerance
AI 理解论文
溯源树
样例
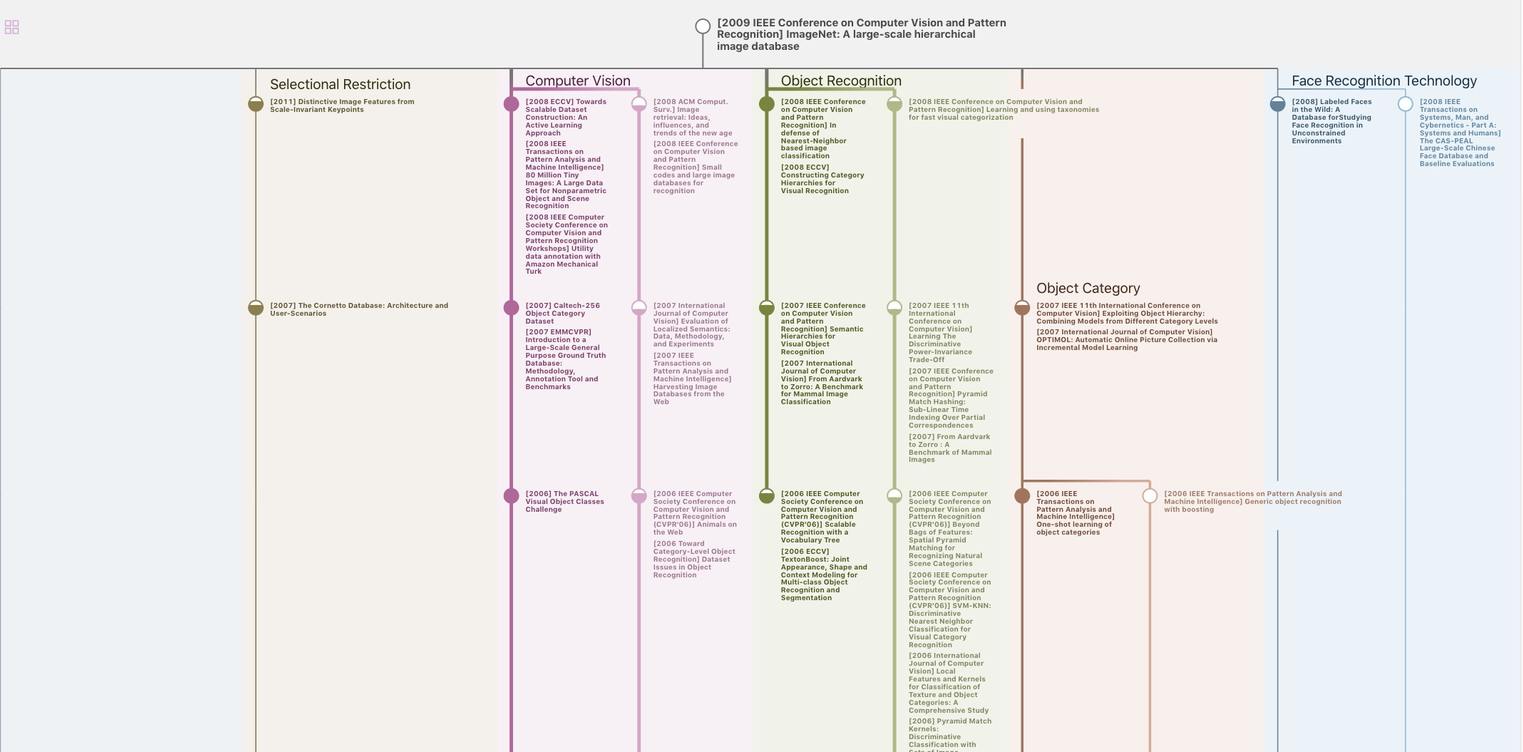
生成溯源树,研究论文发展脉络
Chat Paper
正在生成论文摘要