Online Non-Negative Dictionary Learning Via Moment Information For Sparse Poisson Coding
2016 International Joint Conference on Neural Networks (IJCNN)(2016)
摘要
Online dictionary learning for sparse coding is an effective tool for data analysis. It incrementally learns a set of basis vectors with sparse linear combinations of these vectors when new samples appear. Previous work assumes that the samples embed Gaussian noises, which weaken the power of these methods in handling real applications with non-negative data (e.g., frequency data in word counts). Differently, in this paper, we concentrate on online learning for non-negative dictionary by using moment information for sparse Poisson coding. We exploit the non-negativity of Poisson models to learn a set of non-negative basis vectors and a non-negative sparse linear combination for the moment information of samples. Specifically, we first formulate the online learning problem via the maximum-a-posteriori (MAP) framework. We then propose a novel online algorithm which alternatively updates the sparse-coefficient vector and the basis vectors with non-negativity constraints when a new sample arrives. More importantly, we present sufficient convergence analyses to guarantee the performance of the proposed algorithm, which leads to convergence of a stable dictionary for characterizing the moment information of samples. We finally conduct a series of experiments on word-counts data and image data to show merits of the proposed online algorithm.
更多查看译文
关键词
online nonnegative dictionary learning,moment information,sparse Poisson coding,Gaussian noises,Poisson models nonnegativity,nonnegative basis vectors,nonnegative sparse linear combination,maximum-a-posteriori framework,MAP framework,sparse-coefficient vector,convergence analyses
AI 理解论文
溯源树
样例
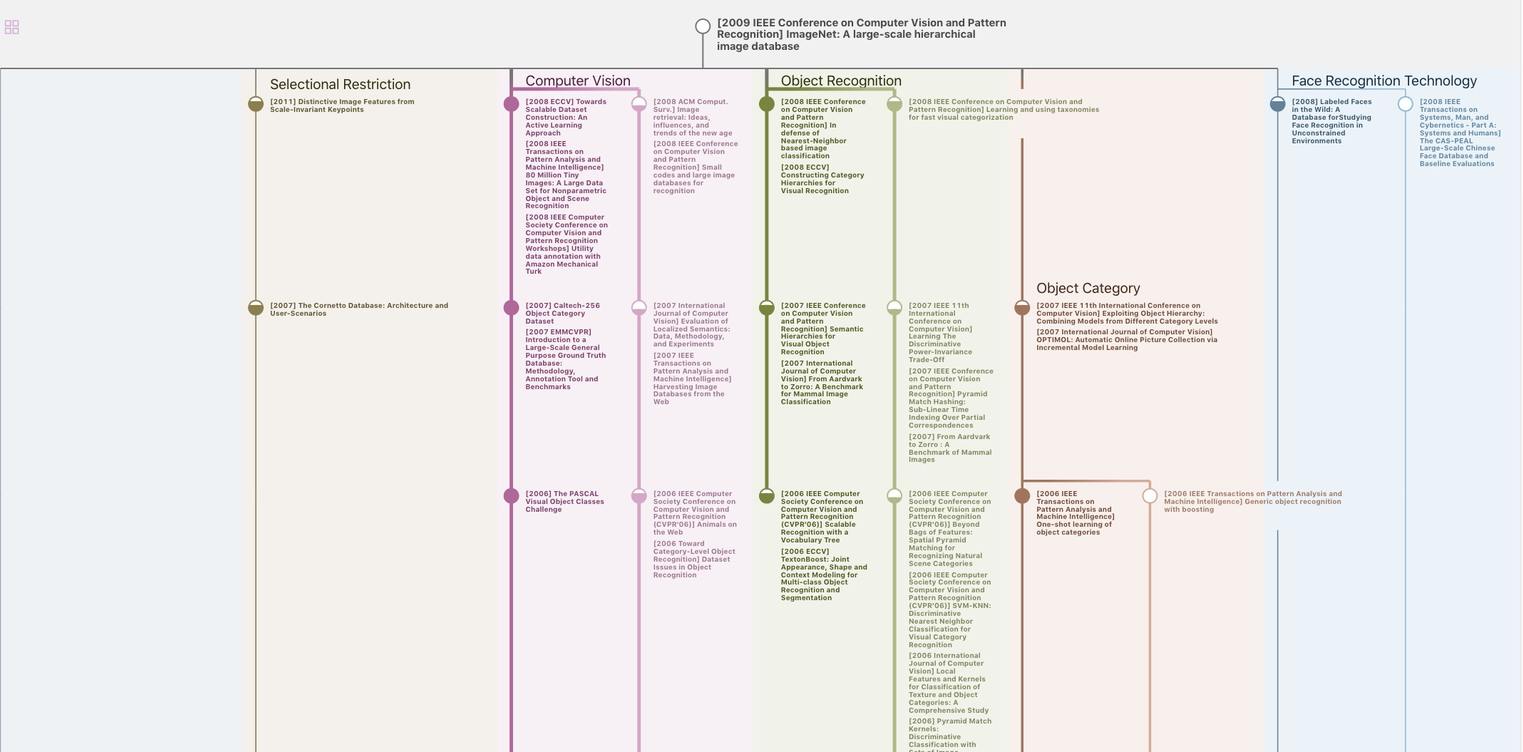
生成溯源树,研究论文发展脉络
Chat Paper
正在生成论文摘要