Speech Enhancement Using Non-negative Low-Rank Modeling with Temporal Continuity and Sparseness Constraints.
PCM(2016)
摘要
Conventional sparse and low-rank decomposition based speech enhancement algorithms seldom simultaneously consider the non-negativity and continuity of the enhanced speech spectrum. In this paper, an unsupervised algorithm for enhancing the noisy speech in a single channel recording is presented. The algorithm can be viewed as an extension of non-negative matrix factorization (NMF) which approximates the magnitude spectrum of noisy speech using the superposition of a low-rank non-negative matrix and a sparse non-negative matrix. The temporal continuity of speech is also considered by incorporating the sum of squared differences between the adjacent frames to the cost function. We prove that by iteratively updating parameters using the derived multiplicative update rules, the cost function finally converges to a local minimum. Simulation experiments on NOIZEUS database with various noise types demonstrate that the proposed algorithm outperforms recently proposed state-of-the-art methods under low signal-to-noise ratio (SNR) conditions.
更多查看译文
关键词
Sparse and low-rank decomposition, Speech enhancement, Non-negative matrix factorization, Temporal continuity
AI 理解论文
溯源树
样例
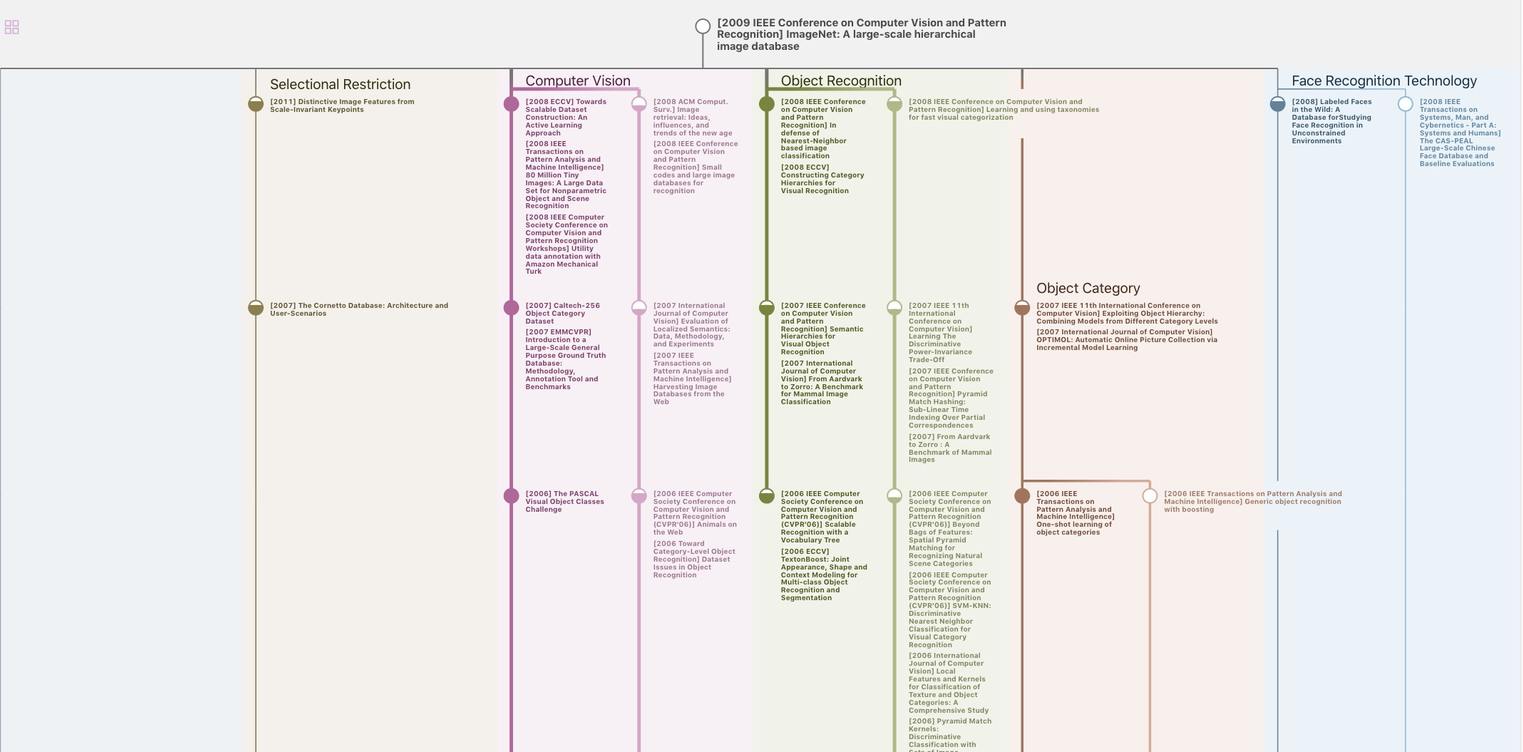
生成溯源树,研究论文发展脉络
Chat Paper
正在生成论文摘要