Supervised Feature Selection by Robust Sparse Reduced-Rank Regression.
ADMA(2016)
摘要
Feature selection keeping discriminative features (i.e., removing noisy and irrelevant features) from high-dimensional data has been becoming a vital important technique in machine learning since noisy/irrelevant features could deteriorate the performance of classification and regression. Moreover, feature selection has also been applied in all kinds of real applications due to its interpretable ability. Motivated by the successful use of sparse learning in machine learning and reduced-rank regression in statics, we put forward a novel feature selection pattern with supervised learning by using a reduced-rank regression model and a sparsity inducing regularizer during this article. Distinguished from those state-of-the-art attribute selection methods, the present method have described below: (1) built upon an (ell _{2,p})-norm loss function and an (ell _{2,p})-norm regularizer by simultaneously considering subspace learning and attribute selection structure into a unite framework; (2) select the more discriminating features in flexible, furthermore, in respect that it may be capable of dominate the degree of sparseness and robust to outlier samples; and (3) also interpretable and stable because it embeds subspace learning (i.e., enabling to output stable models) into the feature selection framework (i.e., enabling to output interpretable results). The relevant results of experiment on eight multi-output data sets indicated the effectiveness of our model compared to the state-of-the-art methods act on regression tasks.
更多查看译文
关键词
Subspace learning, Reduced-rank regression, sparse learning, Supervised feature selection
AI 理解论文
溯源树
样例
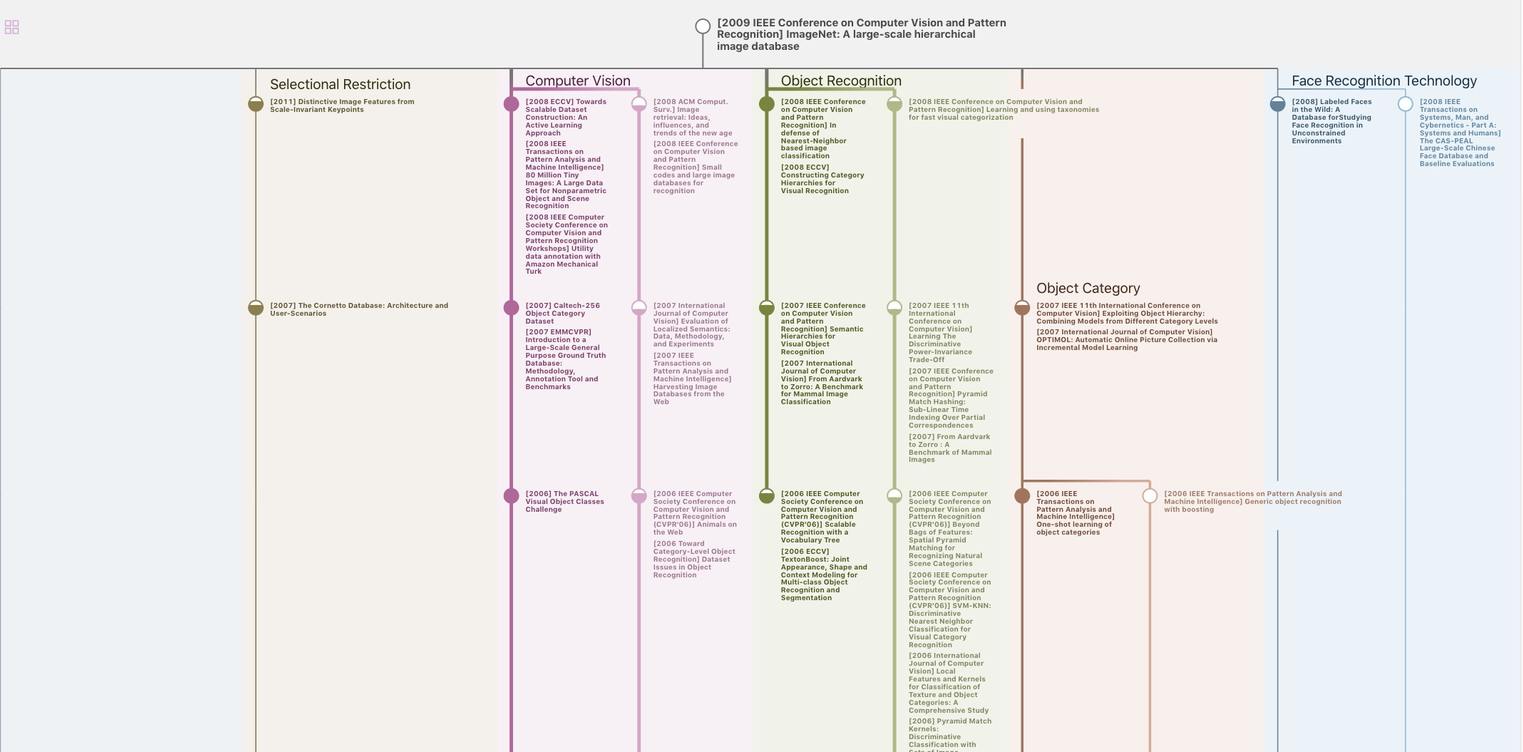
生成溯源树,研究论文发展脉络
Chat Paper
正在生成论文摘要