Sub-Linear Time Compressed Sensing For Support Recovery Using Left And Right Regular Sparse-Graph Codes
2016 IEEE INFORMATION THEORY WORKSHOP (ITW)(2016)
摘要
In [1], [2], two schemes have been proposed to recover the support of a K-sparse N-dimensional signal from noisy linear measurements. Both schemes use left-regular sparse-graph code based sensing matrices and a simple peeling-based decoding algorithm. Both the schemes require O (K log N) measurements and the first scheme require O (N log N) computations whereas the second scheme requires O (K log N) computations (sub-linear time complexity when K is sub-linear in N). We show that by replacing the left-regular ensemble with left and right regular ensemble, we can reduce the number of measurements required of these schemes to the optimal order of O (K log N/K) with decoding complexities of O (K log N/K) and O (N log N/K), respectively.
更多查看译文
关键词
sublinear time compressed sensing,support recovery,right regular sparse-graph code,K-sparse N-dimensional signal,noisy linear measurement,left regular sparse-graph code,sensing matrices,simple peeling-based decoding algorithm,sublinear time complexity
AI 理解论文
溯源树
样例
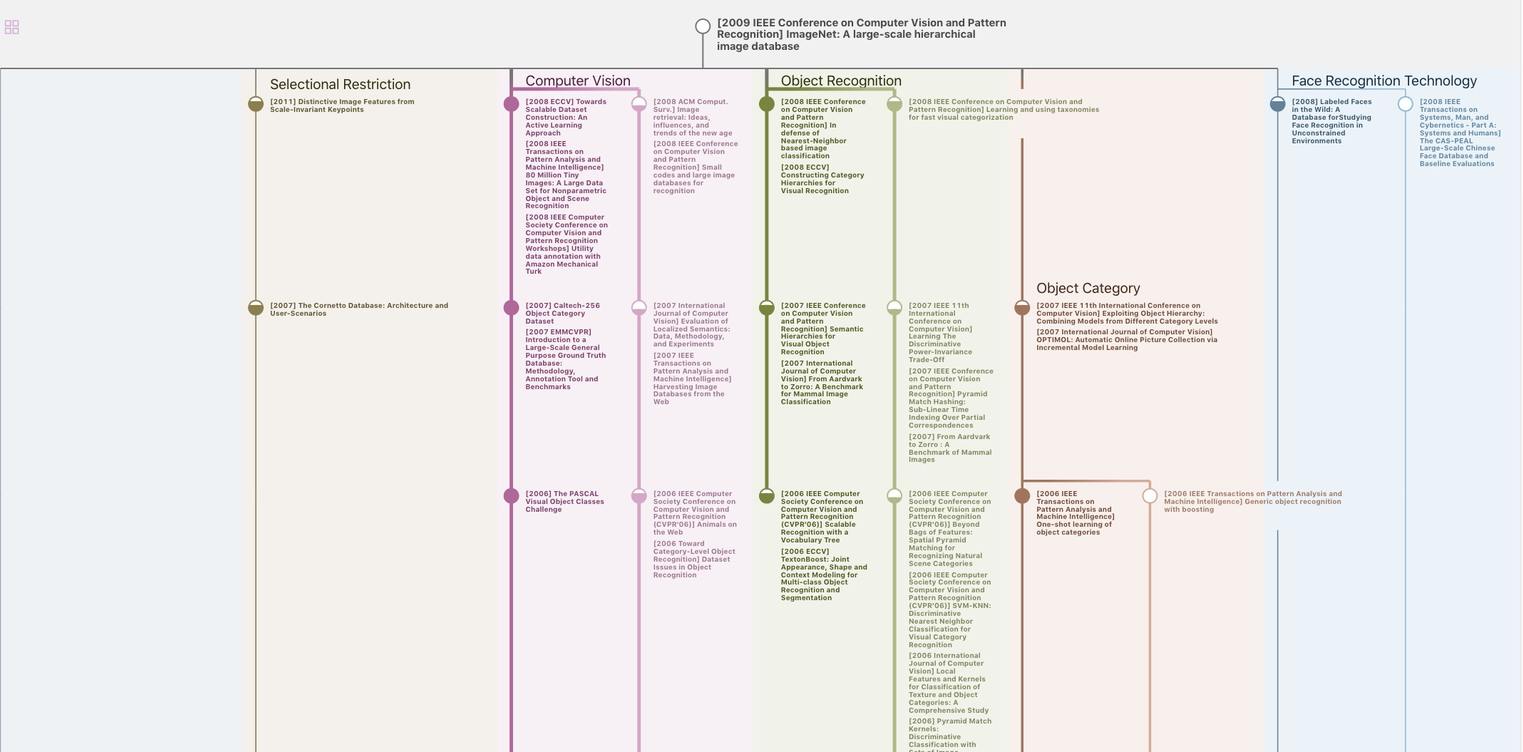
生成溯源树,研究论文发展脉络
Chat Paper
正在生成论文摘要