Brad: Background Regression Based Hyperspectral Anomaly Detection, A K-Nn Score Estimation Aspect
2016 IEEE International Geoscience and Remote Sensing Symposium (IGARSS)(2016)
摘要
Robust background representation is a key issue for detecting anomaly targets in hyperspectral imagery. Meanwhile, the inherent nonlinearity of hyperspectral images may cover up the intrinsic data structure in the anomaly detection process. This paper for the first time aims to implement robust background representation, as well as to explore the intrinsic data structure of the hyperspectral imagery simultaneously. Robust background dataset and potential anomaly dataset are firstly assigned by a robust background regression (RBR) procedure. In the RBR, a minimum squared loss relationship is constructed between the nonlinear data density feature and the corresponding assigned labels, to formulate the foundation of the regression. Furthermore, a manifold regularization term and a robust background average margin maximization term are jointly appended in the RBR procedure. After this, a paired-dataset based k-nn score estimation is undertaken to implement the detection output. Experimental results prove that the proposed method outperforms some other state-of-the-art anomaly detectors.
更多查看译文
关键词
Hyperspectral,anomaly detection,robust background regression,score estimation
AI 理解论文
溯源树
样例
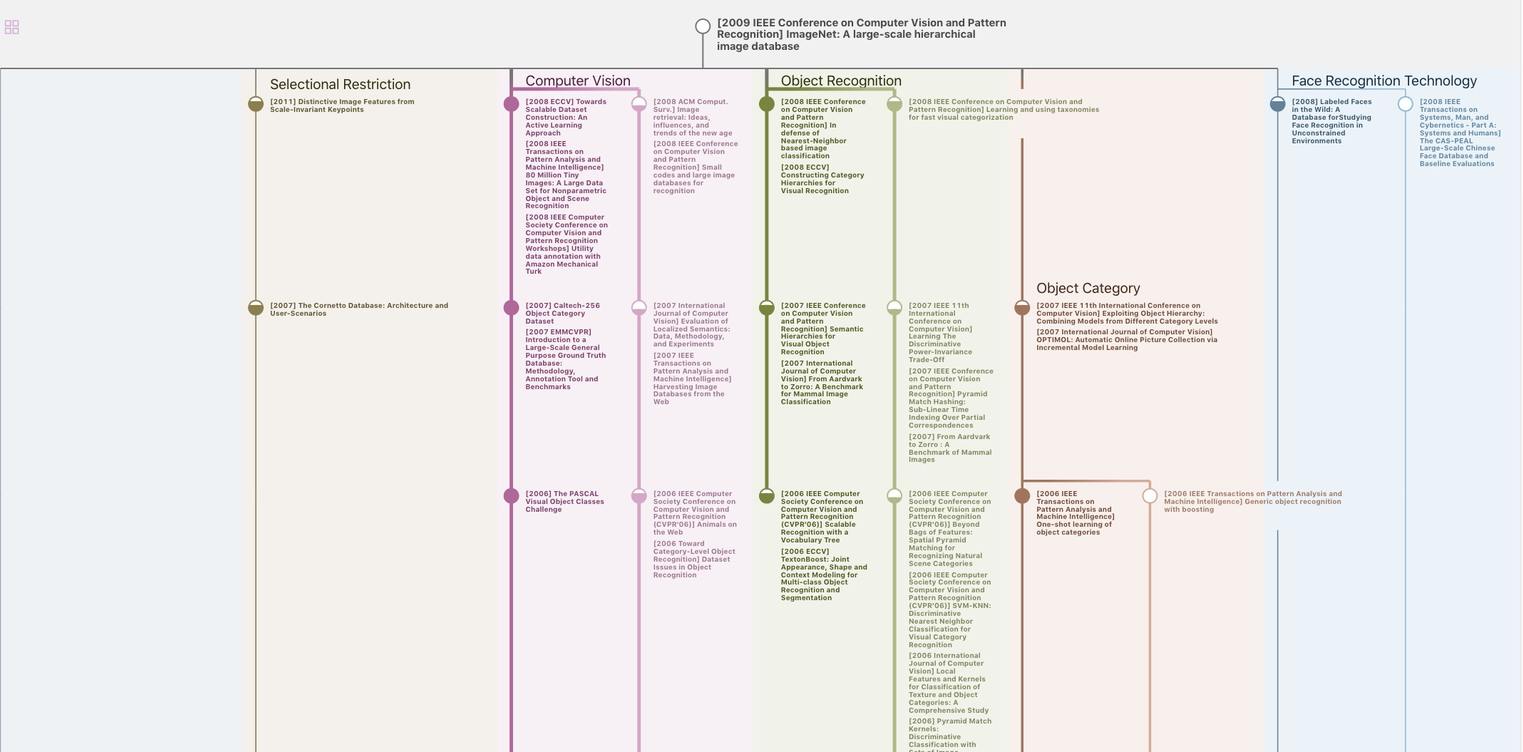
生成溯源树,研究论文发展脉络
Chat Paper
正在生成论文摘要