A Probabilistic Optimum-Path Forest Classifier for Binary Classification Problems.
arXiv: Computer Vision and Pattern Recognition(2016)
摘要
Probabilistic-driven classification techniques extend the role of traditional approaches that output labels (usually integer numbers) only. Such techniques are more fruitful when dealing with problems where one is not interested in recognition/identification only, but also into monitoring the behavior of consumers and/or machines, for instance. Therefore, by means of probability estimates, one can take decisions to work better in a number of scenarios. In this paper, we propose a probabilistic-based Optimum Path Forest (OPF) classifier to handle with binary classification problems, and we show it can be more accurate than naive OPF in a number of datasets. In addition to being just more accurate or not, probabilistic OPF turns to be another useful tool to the scientific community.
更多查看译文
AI 理解论文
溯源树
样例
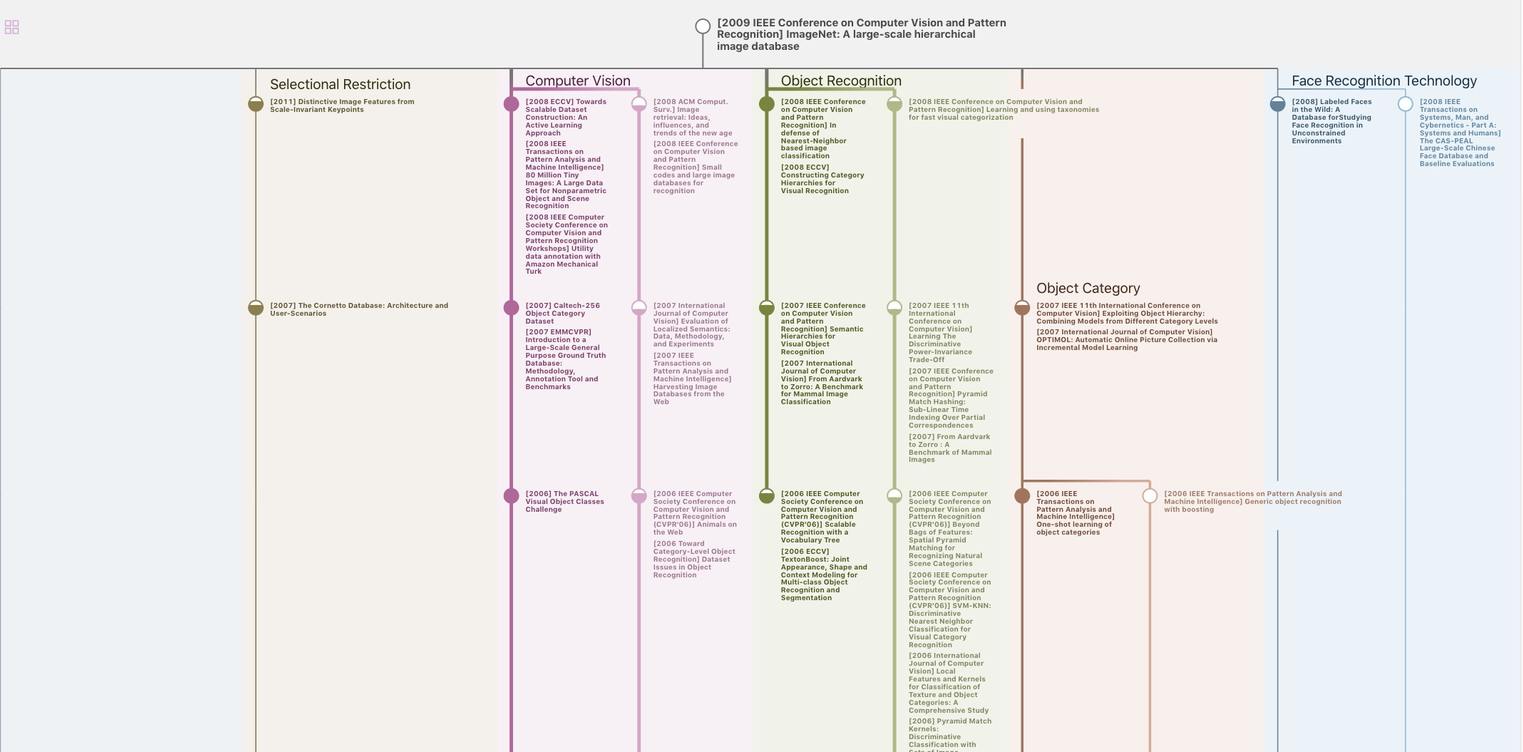
生成溯源树,研究论文发展脉络
Chat Paper
正在生成论文摘要