Faster Kernels for Graphs with Continuous Attributes via Hashing
2016 IEEE 16th International Conference on Data Mining (ICDM)(2016)
摘要
While state-of-the-art kernels for graphs with discrete labels scale well to graphs with thousands of nodes, the few existing kernels for graphs with continuous attributes, unfortunately, do not scale well. To overcome this limitation, we present hash graph kernels, a general framework to derive kernels for graphs with continuous attributes from discrete ones. The idea is to iteratively turn continuous attributes into discrete labels using randomized hash functions. We illustrate hash graph kernels for the Weisfeiler-Lehman subtree kernel and for the shortest-path kernel. The resulting novel graph kernels are shown to be, both, able to handle graphs with continuous attributes and scalable to large graphs and data sets. This is supported by our theoretical analysis and demonstrated by an extensive experimental evaluation.
更多查看译文
关键词
Data Mining,Big Data,Machine Learning,Graph Kernel,Classification,ICDM
AI 理解论文
溯源树
样例
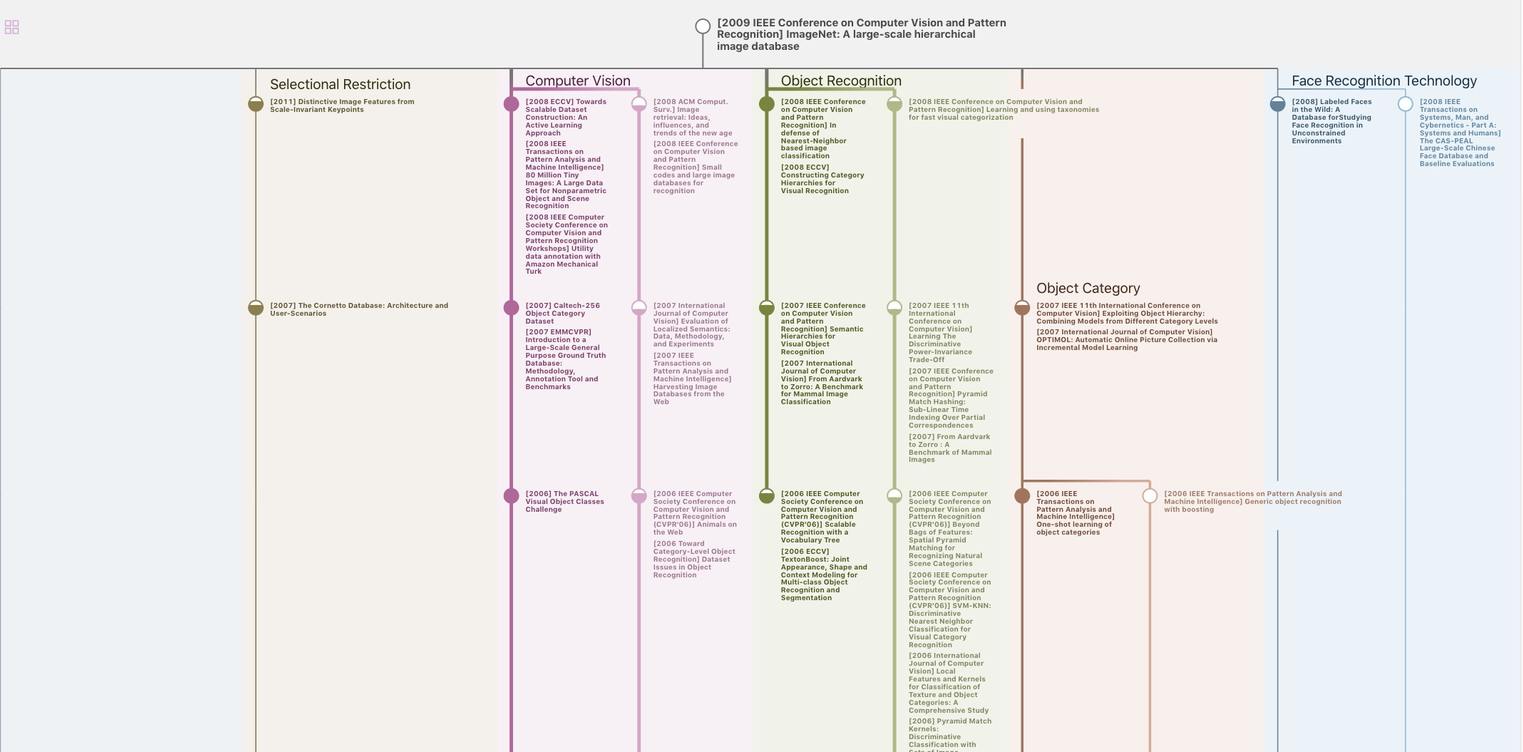
生成溯源树,研究论文发展脉络
Chat Paper
正在生成论文摘要