Optimality of the Johnson-Lindenstrauss Lemma
2017 IEEE 58th Annual Symposium on Foundations of Computer Science (FOCS)(2017)
摘要
For any d, n ≥ 2 and 1/(min{n, d})
0.4999
<; ε <; 1, we show the existence of a set of n vectors X ⊂ ℝ
d
such that any embedding f : X → ℝ
m
satisfying ∀x, y ∈ X, (1-ε)∥x-y∥
2
2
≤ ∥f(x)-f(y)∥
2
2
≤ (1+ε)∥x-y∥
2
2
must have m = Ω(ε
-2
lg n). This lower bound matches the upper bound given by the Johnson-Lindenstrauss lemma [JL84]. Furthermore, our lower bound holds for nearly the full range of ε of interest, since there is always an isometric embedding into dimension min{d, n} (either the identity map, or projection onto span(X)). Previously such a lower bound was only known to hold against linear maps f, and not for such a wide range of parameters ε, n, d [LN16]. The best previously known lower bound for general f was m = Ω(ε
-2
lg n/ lg(1/ε)) [Wel74], [Alo03], which is suboptimal for any ε = o(1).
更多查看译文
关键词
dimensionality reduction,Johnson-Lindenstrauss,random projections
AI 理解论文
溯源树
样例
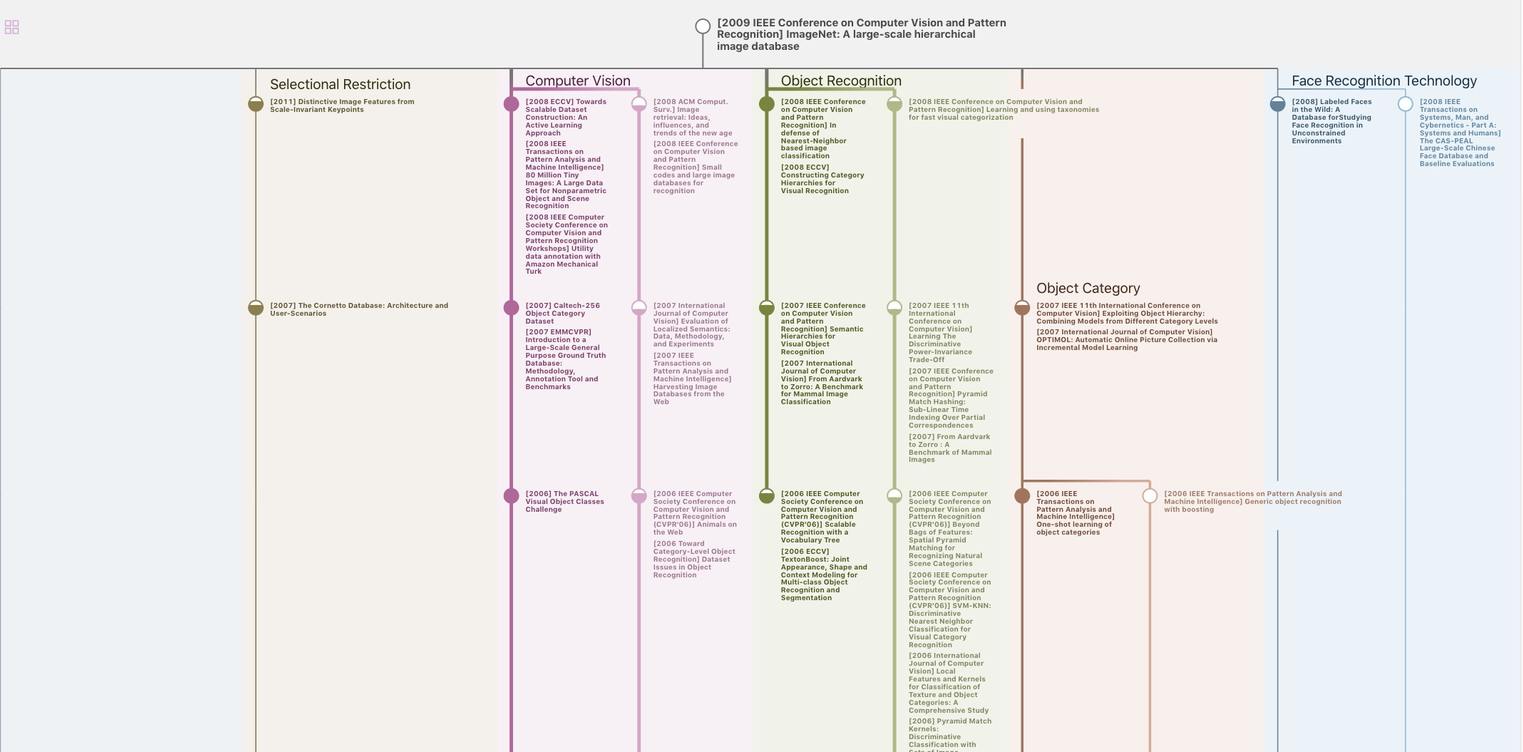
生成溯源树,研究论文发展脉络
Chat Paper
正在生成论文摘要