HyperNetworks
ICLR(2016)
摘要
This work explores hypernetworks: an approach of using a one network, also known as a hypernetwork, to generate the weights for another network. Hypernetworks provide an abstraction that is similar to what is found in nature: the relationship between a genotype - the hypernetwork - and a phenotype - the main network. Though they are also reminiscent of HyperNEAT in evolution, our hypernetworks are trained end-to-end with backpropagation and thus are usually faster. The focus of this work is to make hypernetworks useful for deep convolutional networks and long recurrent networks, where hypernetworks can be viewed as relaxed form of weight-sharing across layers. Our main result is that hypernetworks can generate non-shared weights for LSTM and achieve near state-of-the-art results on a variety of sequence modelling tasks including character-level language modelling, handwriting generation and neural machine translation, challenging the weight-sharing paradigm for recurrent networks. Our results also show that hypernetworks applied to convolutional networks still achieve respectable results for image recognition tasks compared to state-of-the-art baseline models while requiring fewer learnable parameters.
更多查看译文
AI 理解论文
溯源树
样例
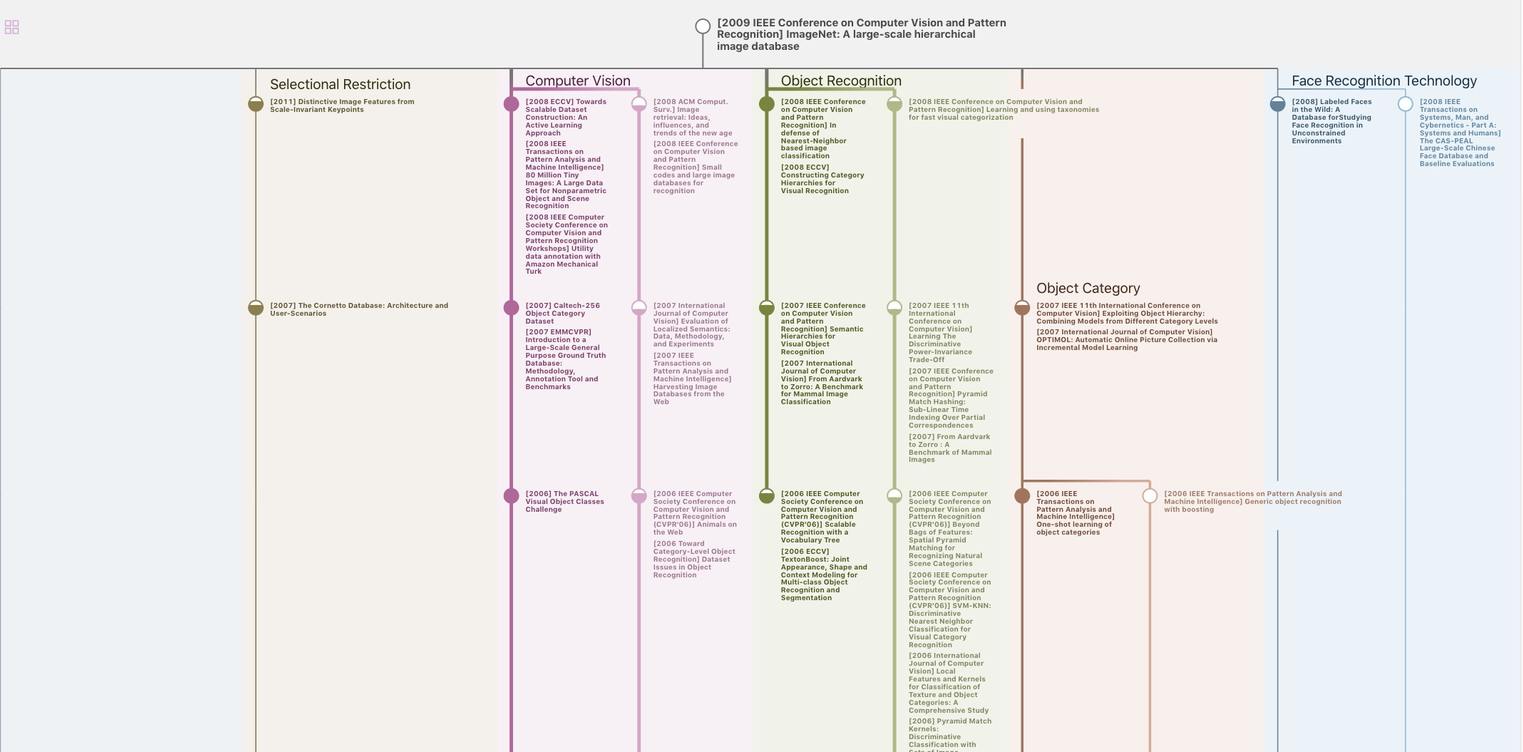
生成溯源树,研究论文发展脉络
Chat Paper
正在生成论文摘要