Learning gene regulatory networks from gene expression data using weighted consensus.
Neurocomputing(2017)
摘要
An accurate determination of the network structure of gene regulatory systems from high-throughput gene expression data is an essential yet challenging step in studying how the expression of endogenous genes is controlled through a complex interaction of gene products and DNA. While numerous methods have been proposed to infer the structure of gene regulatory networks, none of them seem to work consistently over different data sets with high accuracy. A recent study to compare gene network inference methods showed that an average-ranking-based consensus method consistently performs well under various settings. Here, we propose a linear programming-based consensus method for the inference of gene regulatory networks. Unlike the average-ranking-based one, which treats the contribution of each individual method equally, our new consensus method assigns a weight to each method based on its credibility. As a case study, we applied the proposed consensus method on synthetic and real microarray data sets, and compared its performance to that of the average-ranking-based consensus and individual inference methods. Our results show that our weighted consensus method achieves superior performance over the unweighted one, suggesting that assigning weights to different individual methods rather than giving them equal weights improves the accuracy.
更多查看译文
关键词
Gene regulatory networks,Gene expression,Consensus,Structure learning
AI 理解论文
溯源树
样例
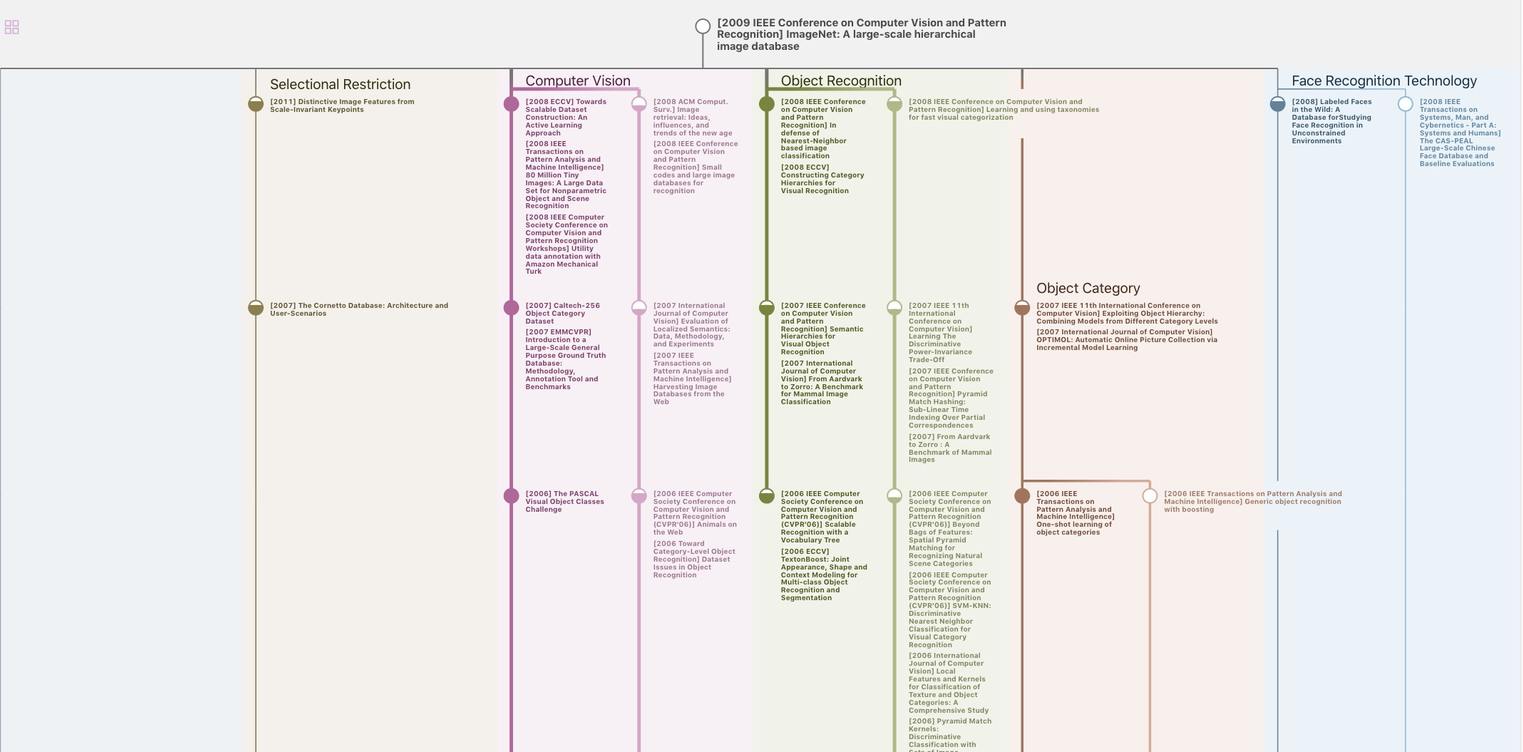
生成溯源树,研究论文发展脉络
Chat Paper
正在生成论文摘要