Enabling Factor Analysis On Thousand-Subject Neuroimaging Datasets
2016 IEEE INTERNATIONAL CONFERENCE ON BIG DATA (BIG DATA)(2016)
摘要
The scale of functional magnetic resonance image data is rapidly increasing as large multi-subject datasets are becoming widely available and high-resolution scanners are adopted. The inherent low-dimensionality of the information in this data has led neuroscientists to consider factor analysis methods to extract and analyze the underlying brain activity. In this work, we consider two recent multi-subject factor analysis methods: the Shared Response Model and the Hierarchical Topographic Factor Analysis. We perform analytical, algorithmic, and code optimization to enable multi-node parallel implementations to scale. Single-node improvements result in 99x and 2062x speedups on the two methods, and enables the processing of larger datasets. Our distributed implementations show strong scaling of 3.3x and 5.5x respectively with 20 nodes on real datasets. We demonstrate weak scaling on a synthetic dataset with 1024 subjects, equivalent in size to the biggest fMRI dataset collected until now, on up to 1024 nodes and 32,768 cores.
更多查看译文
关键词
functional Magnetic Resonance Imaging, Multi-subject Analysis, Scaling, Factor Analysis
AI 理解论文
溯源树
样例
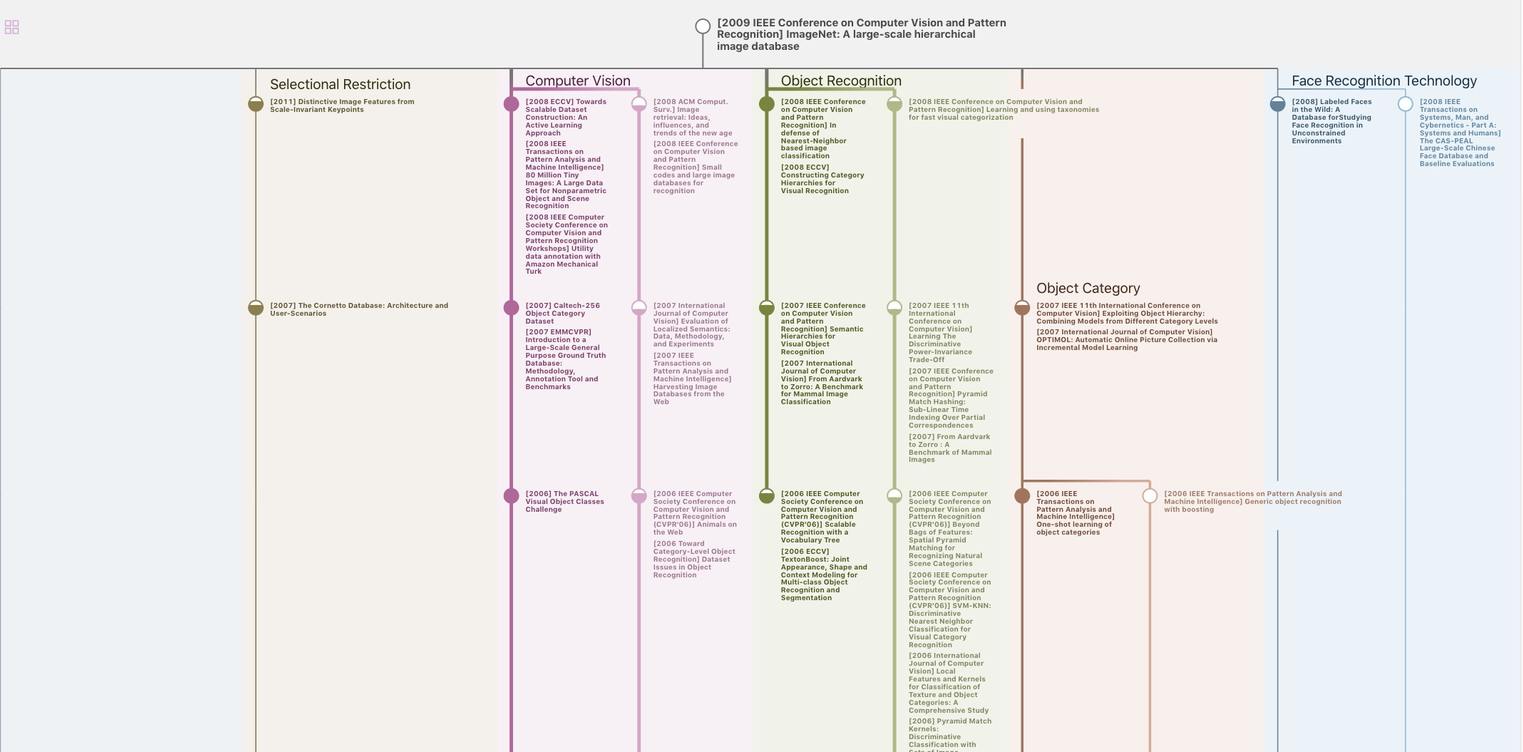
生成溯源树,研究论文发展脉络
Chat Paper
正在生成论文摘要