Approximating Probability Distributions With Short Vectors, Via Information Theoretic Distance Measures
2016 IEEE International Symposium on Information Theory (ISIT)(2016)
摘要
Given a probability distribution p = (p(1),...,p(n)) and an integer m < n, what is the probability distribution q = (q(1),...,q(m)) that is "the closest" to p, that is, that best approximates p ? It is clear that the answer depends on the function one chooses to evaluate the goodness of the approximation. In this paper we provide a general criterion to approximate p with a shorter vector q by using ideas from majorization theory. We evaluate the goodness of our approximation by means of a variety of information theoretic distance measures.
更多查看译文
关键词
probability distribution approximation,short-vectors,information theoretic distance measures,majorization theory
AI 理解论文
溯源树
样例
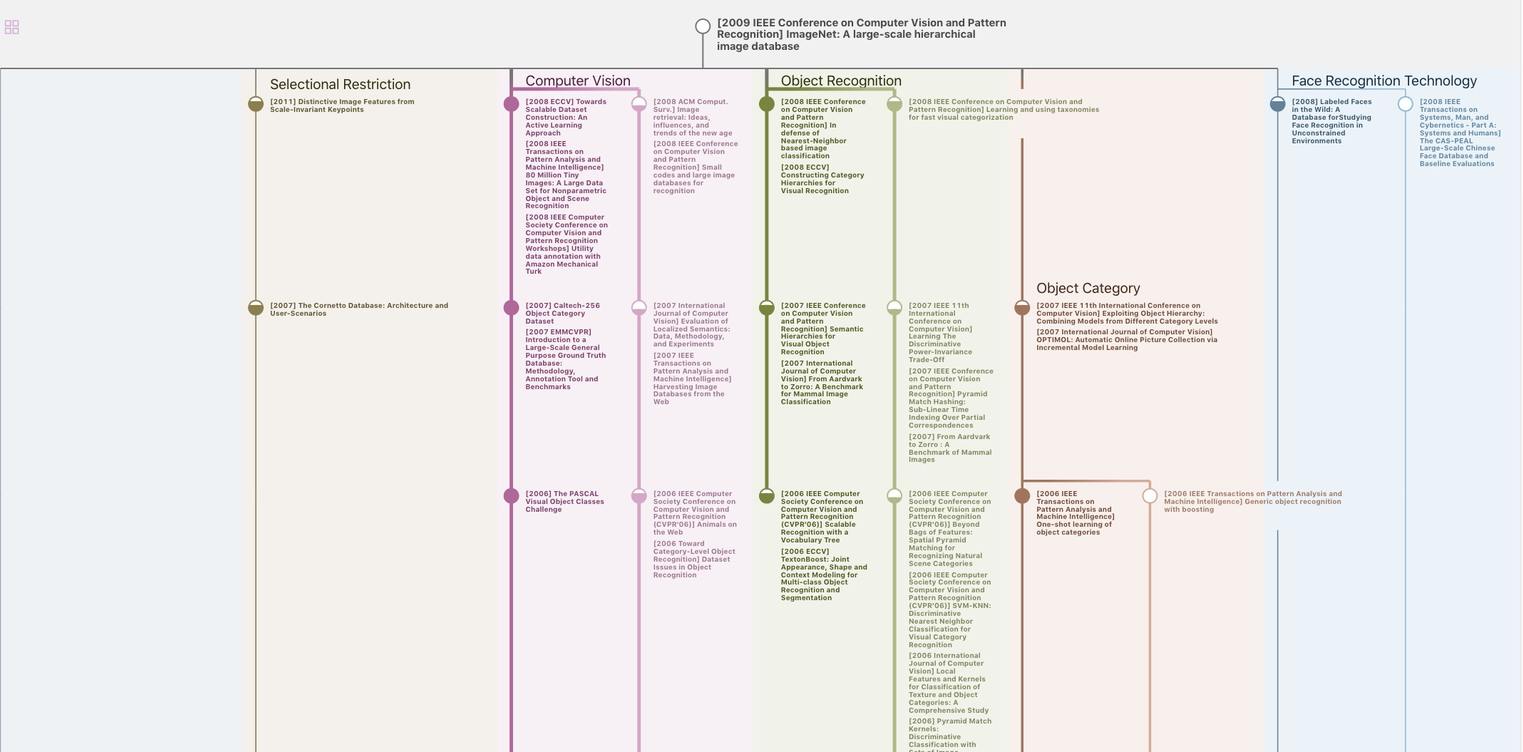
生成溯源树,研究论文发展脉络
Chat Paper
正在生成论文摘要