Student Skill Models in Adaptive Testing.
Probabilistic Graphical Models(2016)
摘要
This paper provides a common framework, a generic model, for Computerized Adaptive Testing (CAT) for different model types. We present question selection methods for CAT for this generic model. We use three different types of models, Item Response Theory, Bayesian Networks, and Neural Networks, that instantiate the generic model. We illustrate the usefulness of a special model condition ‐ the monotonicity ‐ and discuss its inclusion in these model types. With Bayesian networks we use specific type of learning using generalized linear models to ensure the monotonicity. We conducted simulated CAT tests on empirical data. Behavior of individual models was assessed based on these tests. The best performing model was the BN model constructed by a domain expert; its parameters were learned from data under the monotonicity condition.
更多查看译文
AI 理解论文
溯源树
样例
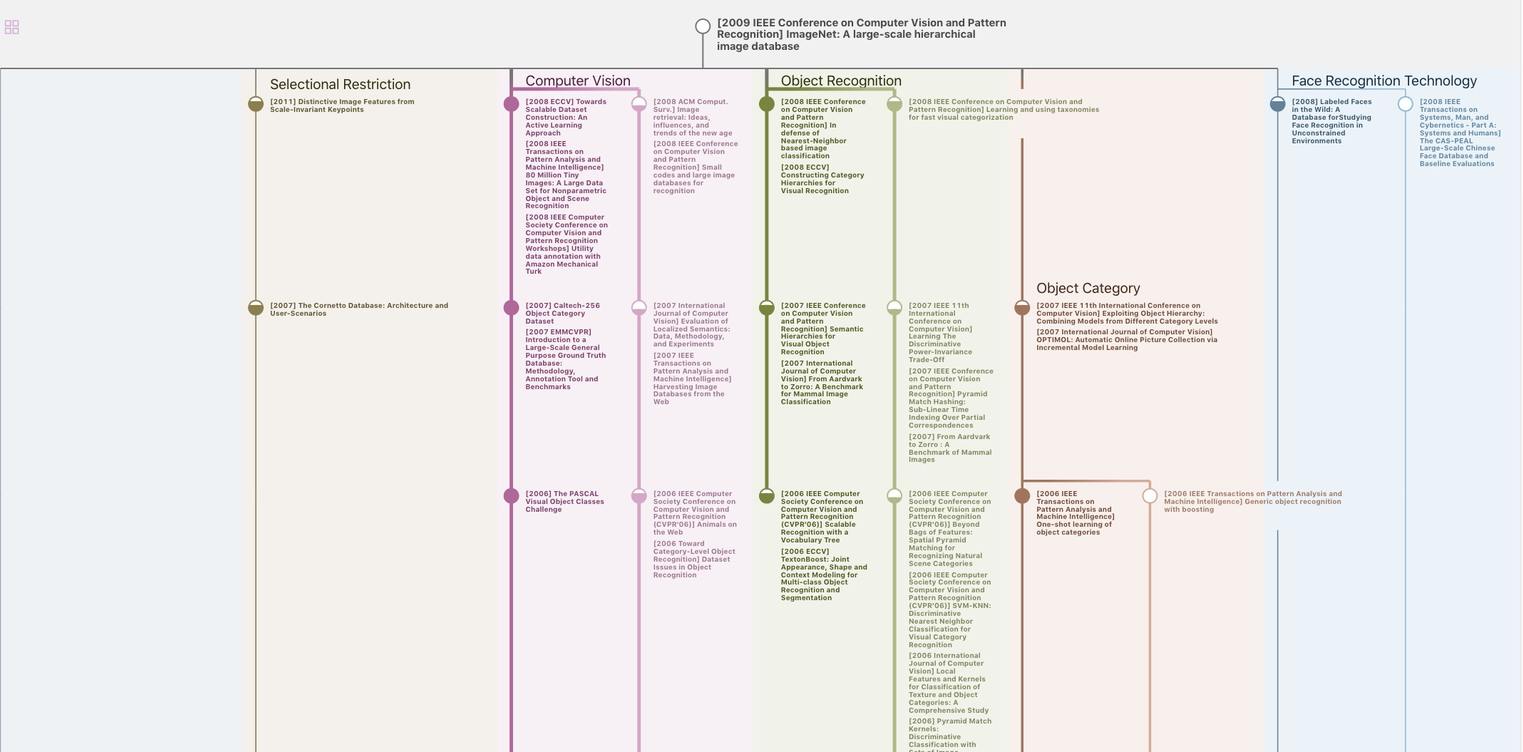
生成溯源树,研究论文发展脉络
Chat Paper
正在生成论文摘要