Linear-time online action detection from 3D skeletal data using bags of gesturelets
2016 IEEE Winter Conference on Applications of Computer Vision (WACV)(2016)
摘要
Sliding window is one direct way to extend a successful recognition system to handle the more challenging detection problem. While action recognition decides only whether or not an action is present in a pre-segmented video sequence, action detection identifies the time interval where the action occurred in an unsegmented video stream. Sliding window approaches can however be slow as they maximize a classifier score over all possible sub-intervals. Even though new schemes utilize dynamic programming to speed up the search for the optimal sub-interval, they require offline processing on the whole video sequence. In this paper, we propose a novel approach for online action detection based on 3D skeleton sequences extracted from depth data. It identifies the sub-interval with the maximum classifier score in linear time. Furthermore, it is suitable for real-time applications with low latency.
更多查看译文
关键词
linear-time online action detection,3D skeletal data,bags of gesturelets,sliding window,action recognition,presegmented video sequence,classifier,real-time applications
AI 理解论文
溯源树
样例
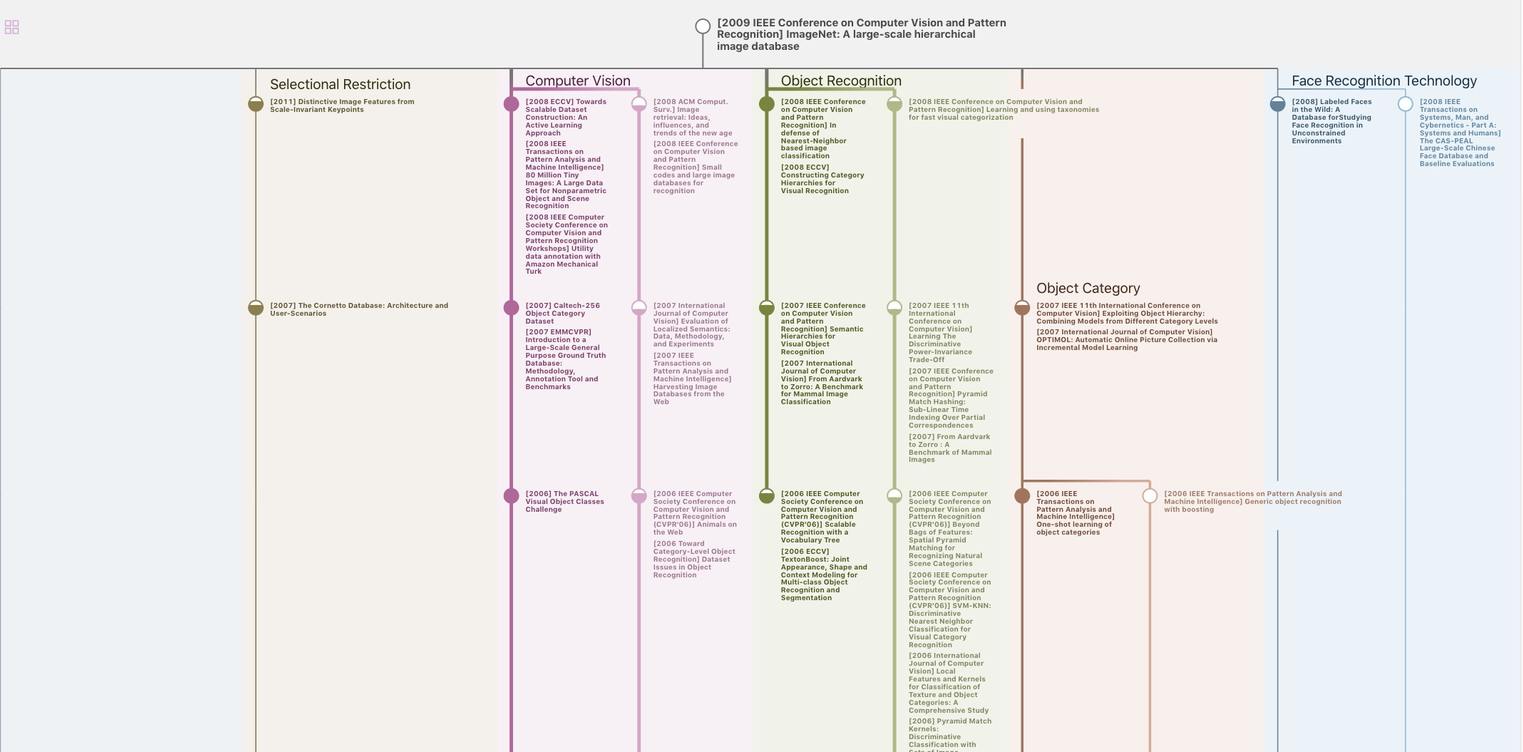
生成溯源树,研究论文发展脉络
Chat Paper
正在生成论文摘要