Patch forest: a hybrid framework of random forest and patch-based segmentation.
Proceedings of SPIE(2016)
摘要
The development of an accurate, robust and fast segmentation algorithm has long been a research focus in medical computer vision. State-of-the-art practices often involve non-rigidly registering a target image with a set of training atlases for label propagation over the target space to perform segmentation, a.k.a. multi-atlas label propagation (MALP). In recent years, the patch-based segmentation (PBS) framework has gained wide attention due to its advantage of relaxing the strict voxel-to-voxel correspondence to a series of pair-wise patch comparisons for contextual pattern matching. Despite a high accuracy reported in many scenarios, computational efficiency has consistently been a major obstacle for both approaches. Inspired by recent work on random forest, in this paper we propose a patch forest approach, which by equipping the conventional PBS with a fast patch search engine, is able to boost segmentation speed significantly while retaining an equal level of accuracy. In addition, a fast forest training mechanism is also proposed, with the use of a dynamic grid framework to efficiently approximate data compactness computation and a 3D integral image technique for fast box feature retrieval.
更多查看译文
关键词
Patch-based segmentation,random forest,hippocampus segmentation
AI 理解论文
溯源树
样例
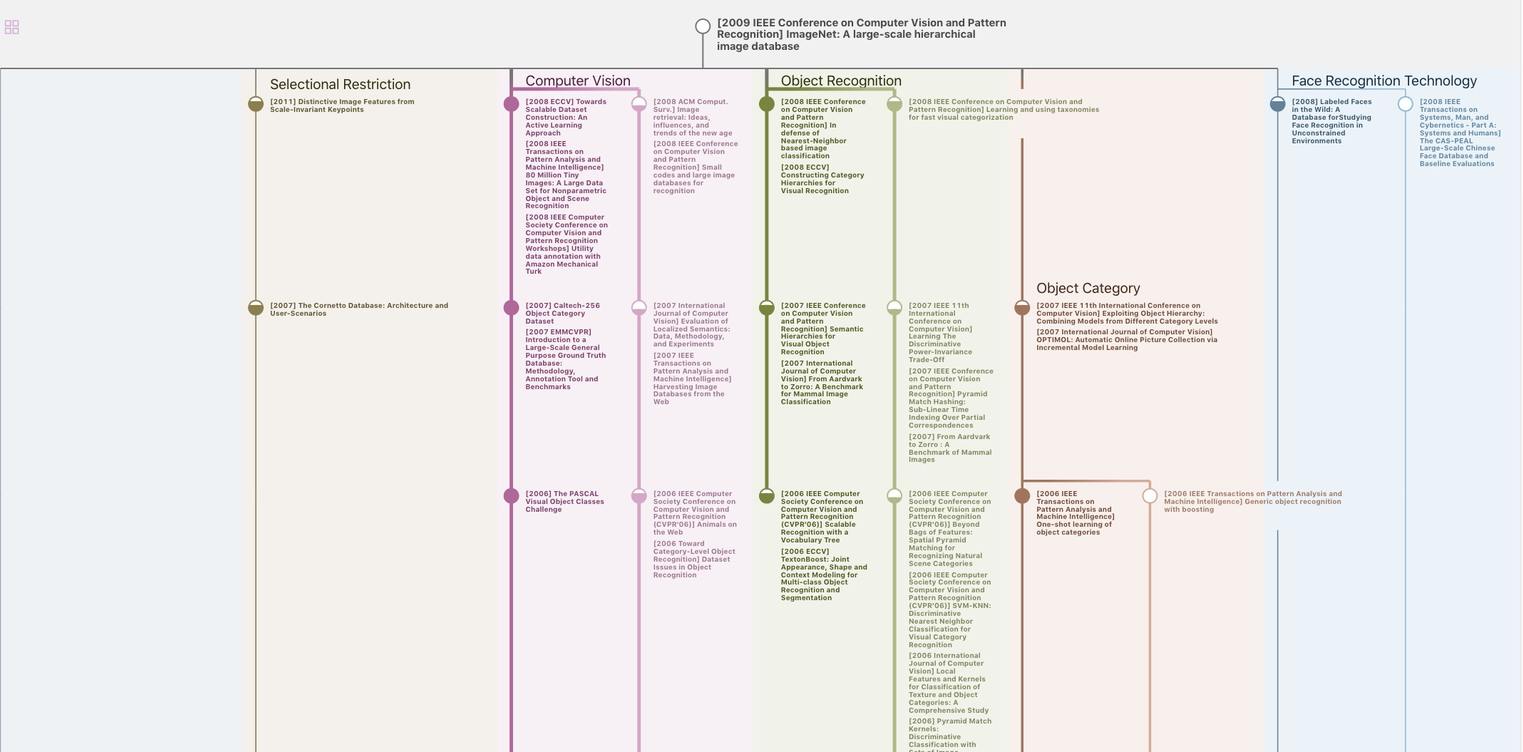
生成溯源树,研究论文发展脉络
Chat Paper
正在生成论文摘要