Estimating Individual Differences For Student Modeling In Intelligent Tutors From Reading And Pretest Data
ITS 2016: Proceedings of the 13th International Conference on Intelligent Tutoring Systems - Volume 9684(2016)
摘要
Past studies have shown that Bayesian Knowledge Tracing (BKT) can predict student performance and implement Cognitive Mastery successfully. Standard BKT individualizes parameter estimates for skills, also referred to as knowledge components (KCs), but not for students. Studies deriving individual student parameters from the data logs of student tutor performance have shown improvements to the standard BKT model fits, and result in different practice recommendations for students. This study investigates whether individual student parameters, specifically individual difference weights (IDWs) [1], can be derived from student activities prior to tutor use. We find that student performance measures in reading instructional text and in a conceptual knowledge pretest can be employed to predict IDWs. Further, we find that a model incorporating these predicted IDWs performs well, in terms of model fit and learning efficiency, when compared to a standard BKT model and a model with best-fitting IDWs derived from tutor performance.
更多查看译文
关键词
BKT,Genetics,Machine learning,Student modeling
AI 理解论文
溯源树
样例
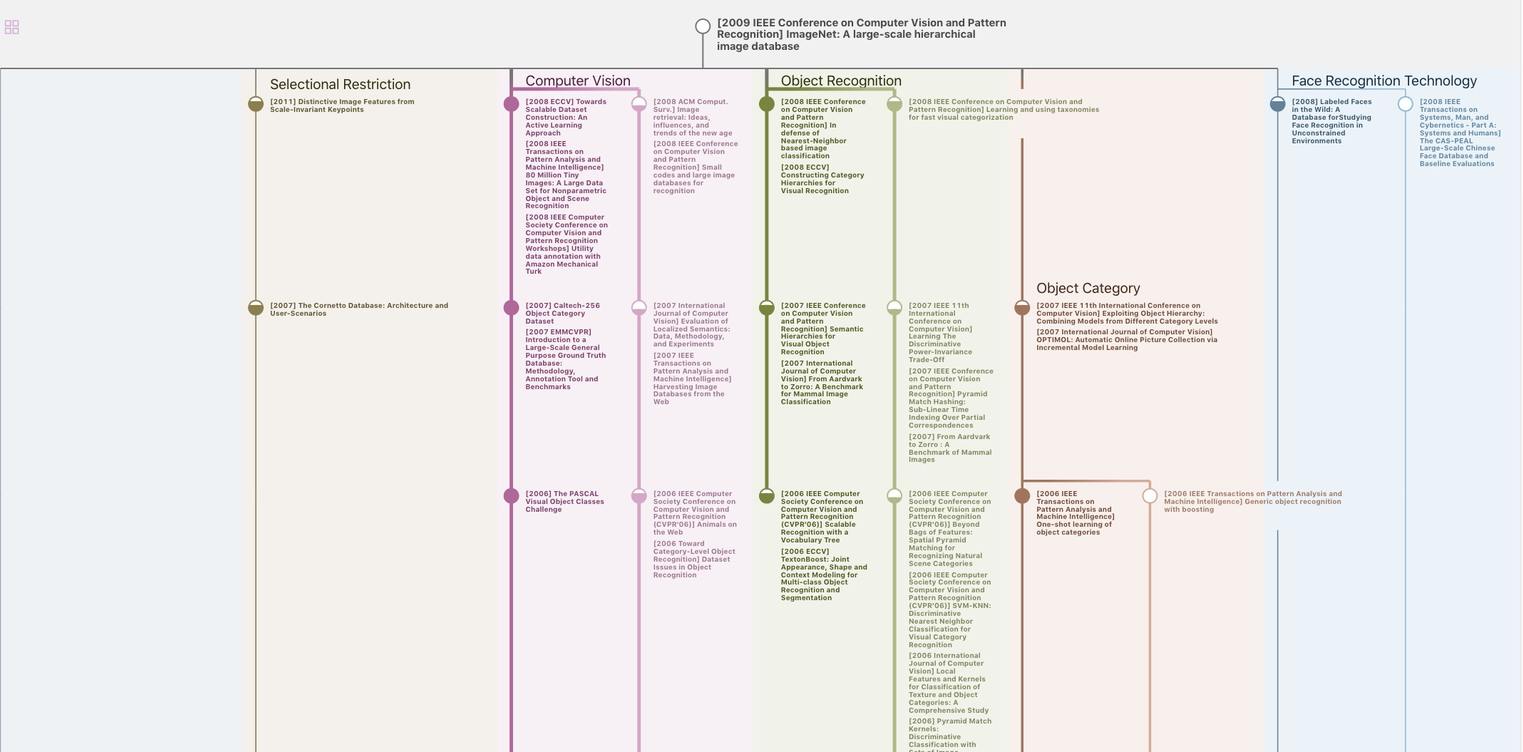
生成溯源树,研究论文发展脉络
Chat Paper
正在生成论文摘要