Reinforcement Learning with Multiple Shared Rewards.
Procedia Computer Science(2016)
摘要
A major concern in multi-agent coordination is how to select algorithms that can lead agents to learn together to achieve certain goals. Much of the research on multi-agent learning relates to reinforcement learning (RL) techniques. One element of RL is the interaction model, which describes how agents should interact with each other and with the environment. Discrete, continuous and objective-oriented interaction models can improve convergence among agents. This paper proposes an approach based on the integration of multi-agent coordination models designed for reward-sharing policies. By taking the best features from each model, better agent coordination is achieved. Our experimental results show that this approach improves convergence among agents even in large state-spaces and yields better results than classical RL approaches.
更多查看译文
关键词
Adaptive Agents,Shared Rewards,Interaction,Learning,Coordination.
AI 理解论文
溯源树
样例
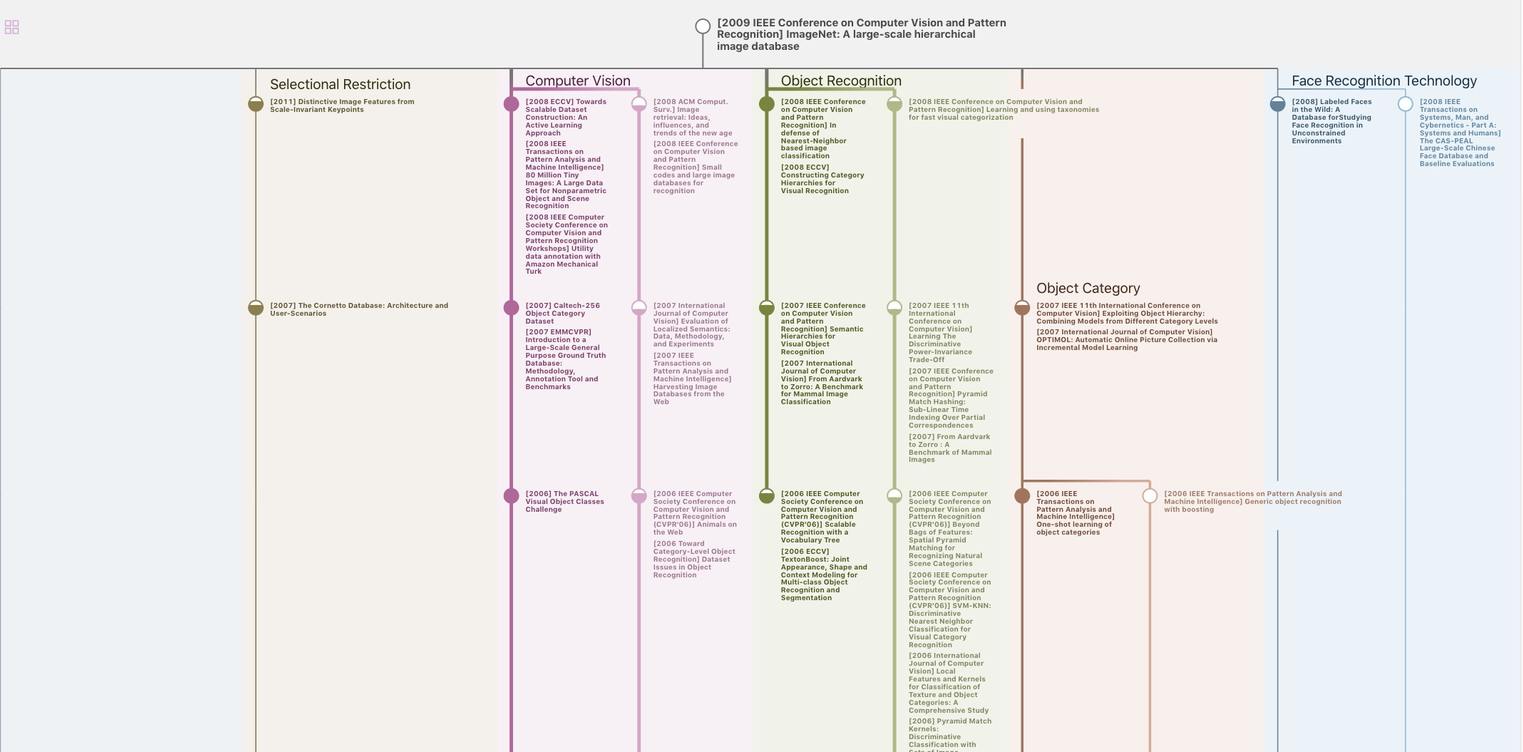
生成溯源树,研究论文发展脉络
Chat Paper
正在生成论文摘要