Mean Response-Time Minimization of a Soft-Cascade Detector.
ICORES(2016)
摘要
In this paper, the problem of minimizing the mean response-time of a soft-cascade detector is addressed. A soft-cascade detector is a machine learning tool used in applications that need to recognize the presence of certain types of object instances in images. Classical soft-cascade learning methods select the weak classifiers that compose the cascade, as well as the classification thresholds applied at each cascade level, so that a desired detection performance is reached. They usually do not take into account its mean response-time, which is also of importance in time-constrained applications. To overcome that, we consider the threshold selection problem aiming to minimize the computation time needed to detect a target object in an image (i.e., by classifying a set of samples). We prove the NP-hardness of the problem and propose a mathematical model that takes benefit from several dominance properties, which are put into evidence. On the basis of computational experiments, we show that we can provide a faster cascade detector, while maintaining the same detection performances.
更多查看译文
AI 理解论文
溯源树
样例
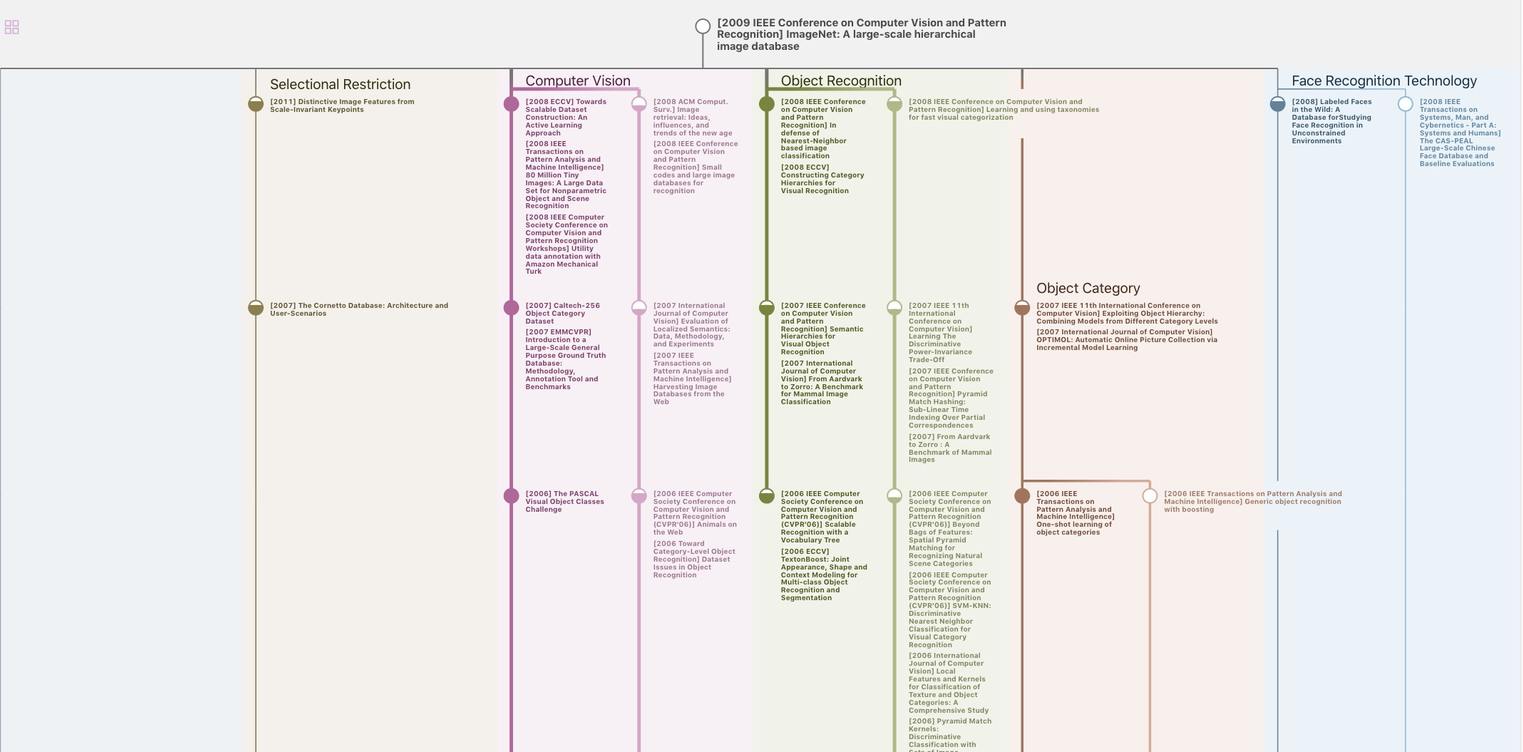
生成溯源树,研究论文发展脉络
Chat Paper
正在生成论文摘要