Effects Of Creativity And Cluster Tightness On Short Text Clustering Performance
PROCEEDINGS OF THE 54TH ANNUAL MEETING OF THE ASSOCIATION FOR COMPUTATIONAL LINGUISTICS, VOL 1(2016)
摘要
Properties of corpora, such as the diversity of vocabulary and how tightly related texts cluster together, impact the best way to cluster short texts. We examine several such properties in a variety of corpora and track their effects on various combinations of similarity metrics and clustering algorithms. We show that semantic similarity metrics outperform traditional n-gram and dependency similarity metrics for k-means clustering of a linguistically creative dataset, but do not help with less creative texts. Yet the choice of similarity metric interacts with the choice of clustering method. We find that graph-based clustering methods perform well on tightly clustered data but poorly on loosely clustered data. Semantic similarity metrics generate loosely clustered output even when applied to a tightly clustered dataset. Thus, the best performing clustering systems could not use semantic metrics.
更多查看译文
AI 理解论文
溯源树
样例
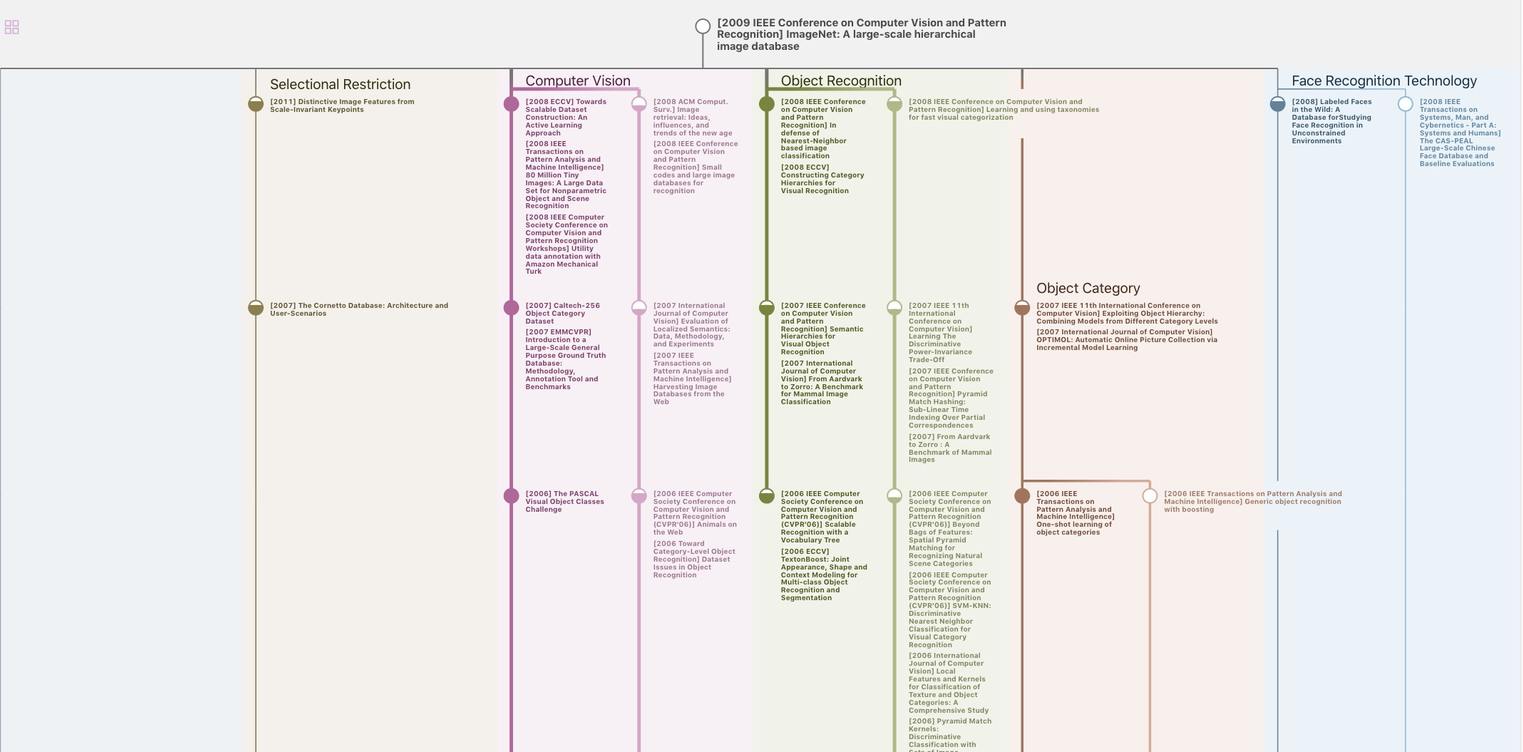
生成溯源树,研究论文发展脉络
Chat Paper
正在生成论文摘要