Sparse representation-based correlation analysis of non-stationary spatiotemporal big data.
INTERNATIONAL JOURNAL OF DIGITAL EARTH(2016)
摘要
As the basic data of digital city and smart city research, Spatiotemporal series data contain rich geographic information. Alongside the accumulation of spatial time-series data, we are also encountering new challenges related to analyzing and mining the correlations among the data. Because the traditional methods of analysis also have their own suitable condition restrictions for the new features, we propose a new analytical framework based on sparse representation to describe the time, space, and spatial-time correlation. First, before analyzing the correlation, we discuss sparse representation based on the K-singular value decomposition (K-SVD) algorithm to ensure that the sparse coefficients are in the same sparse domain. We then present new computing methods to calculate the time, spatial, and spatial-time correlation coefficients in the sparse domain; we then discuss the functions' properties. Finally, we discuss change regulations for the gross domestic product (GDP), population, and Normalized Difference Vegetation Index (NDVI) spatial time-series data in China's Jing-Jin-Ji region to confirm the effectiveness and adaptability of the new methods.
更多查看译文
关键词
Sparse representation,correlation analysis,Spatiotemporal data,spatial data analysis
AI 理解论文
溯源树
样例
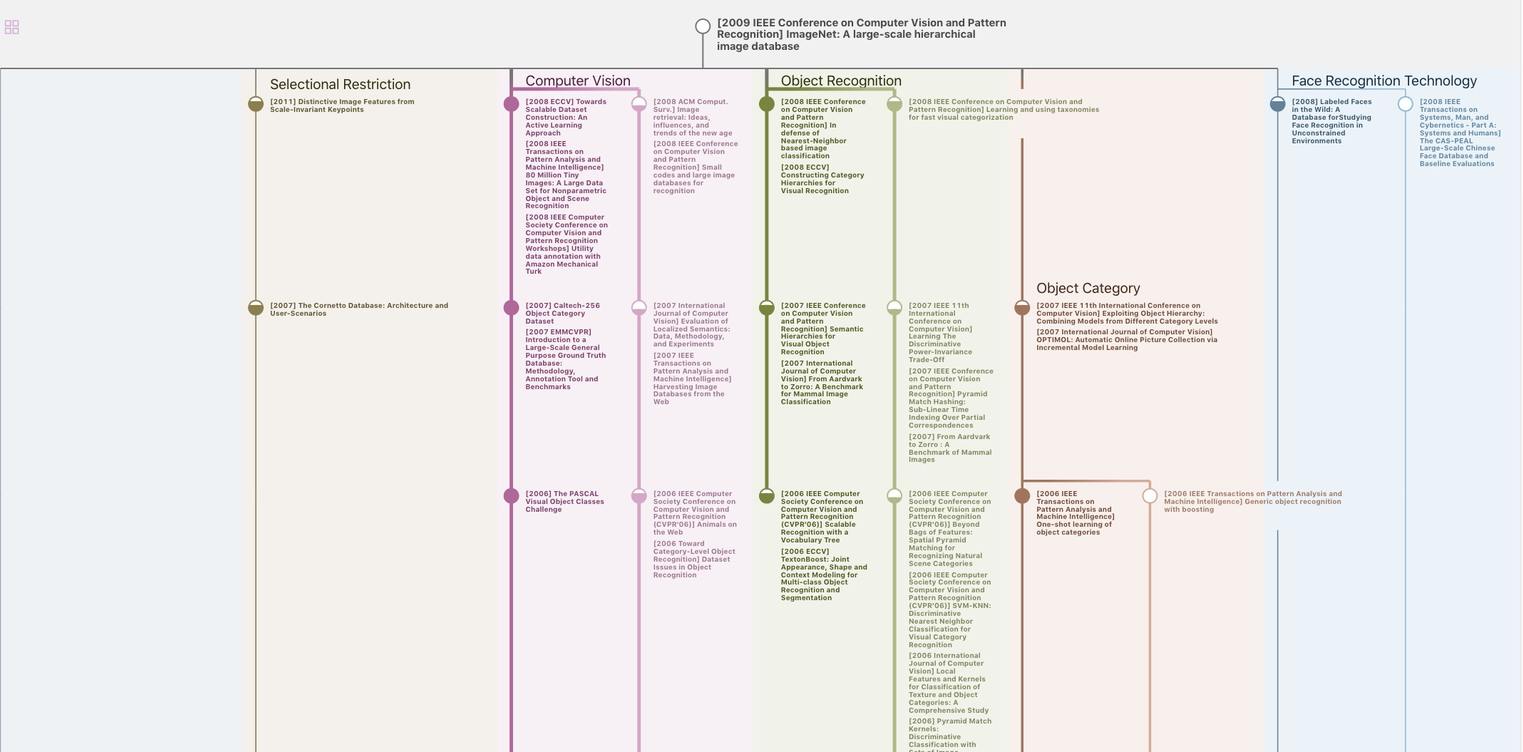
生成溯源树,研究论文发展脉络
Chat Paper
正在生成论文摘要