Isolated Sign Language Recognition With Grassmann Covariance Matrices
ACM Transactions on Accessible Computing(2016)
摘要
In this article, to utilize long-term dynamics over an isolated sign sequence, we propose a covariance matrix-based representation to naturally fuse information from multimodal sources. To tackle the drawback induced by the commonly used Riemannian metric, the proximity of covariance matrices is measured on the Grassmann manifold. However, the inherent Grassmann metric cannot be directly applied to the covariance matrix. We solve this problem by evaluating and selecting the most significant singular vectors of covariance matrices of sign sequences. The resulting compact representation is called the Grassmann covariance matrix. Finally, the Grassmann metric is used to be a kernel for the support vector machine, which enables learning of the signs in a discriminative manner. To validate the proposed method, we collect three challenging sign language datasets, on which comprehensive evaluations show that the proposed method outperforms the state-of-the-art methods both in accuracy and computational cost.
更多查看译文
关键词
Hearing loss,sign language,covariance matrix,Grassmann manifold
AI 理解论文
溯源树
样例
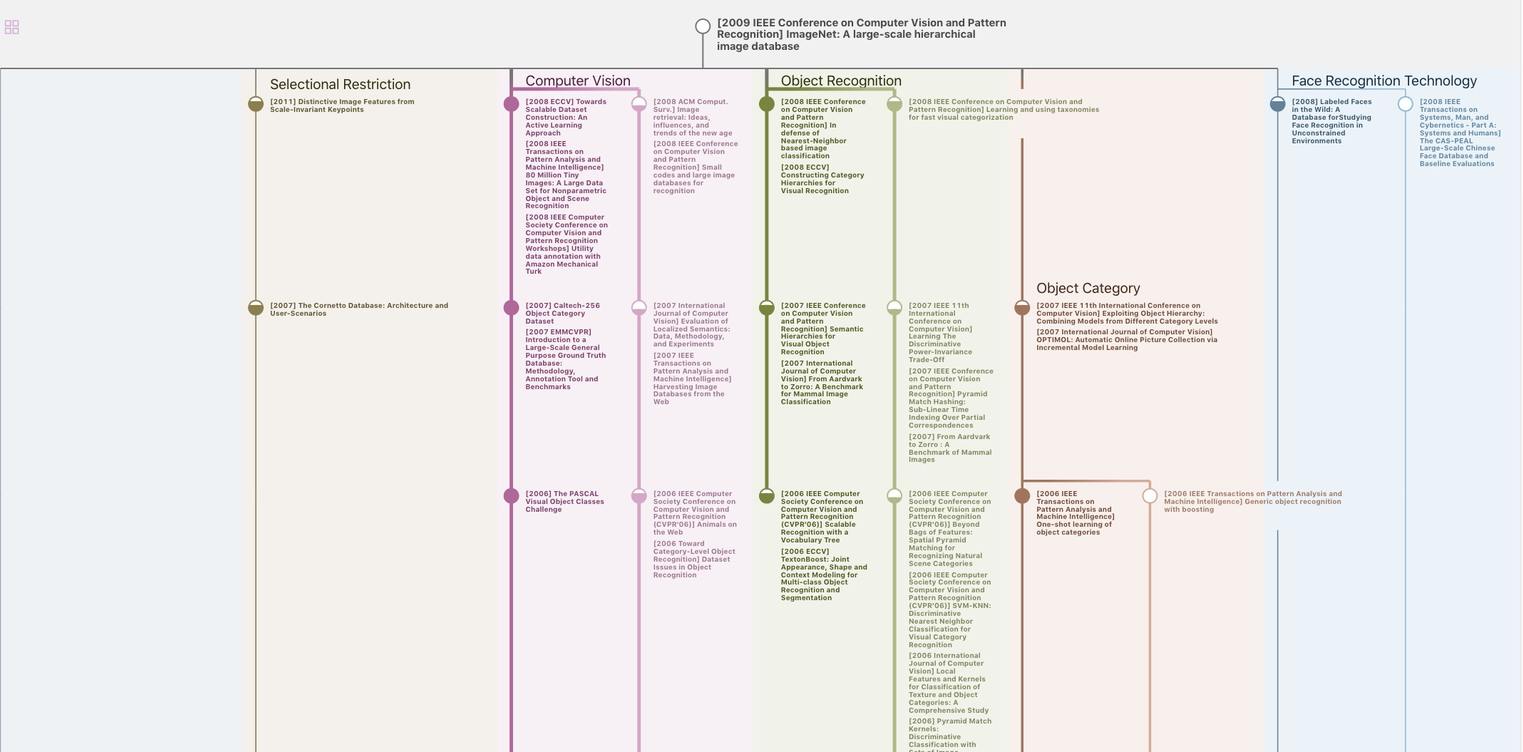
生成溯源树,研究论文发展脉络
Chat Paper
正在生成论文摘要