Multi-Source Multi-View Clustering via Discrepancy Penalty
2016 International Joint Conference on Neural Networks (IJCNN)(2016)
摘要
With the advance of technology, entities can be observed in multiple views. Multiple views containing different types of features can be used for clustering. Although multi-view clustering has been successfully applied in many applications, the previous methods usually assume the complete instance mapping between different views. In many real-world applications, information can be gathered from multiple sources, while each source can contain multiple views, which are more cohesive for learning. The views under the same source are usually fully mapped, but they can be very heterogeneous. Moreover, the mappings between different sources are usually incomplete and partially observed, which makes it more difficult to integrate all the views across different sources. In this paper, we propose MMC (Multi-source Multi-view Clustering), which is a framework based on collective spectral clustering with a discrepancy penalty across sources, to tackle these challenges. MMC has several advantages compared with other existing methods. First, MMC can deal with incomplete mapping between sources. Second, it considers the disagreements between sources while treating views in the same source as a cohesive set. Third, MMC also tries to infer the instance similarities across sources to enhance the clustering performance. Extensive experiments conducted on real-world data demonstrate the effectiveness of the proposed approach.
更多查看译文
关键词
multisource multiview clustering,discrepancy penalty,instance mapping,learning,MMC,collective spectral clustering
AI 理解论文
溯源树
样例
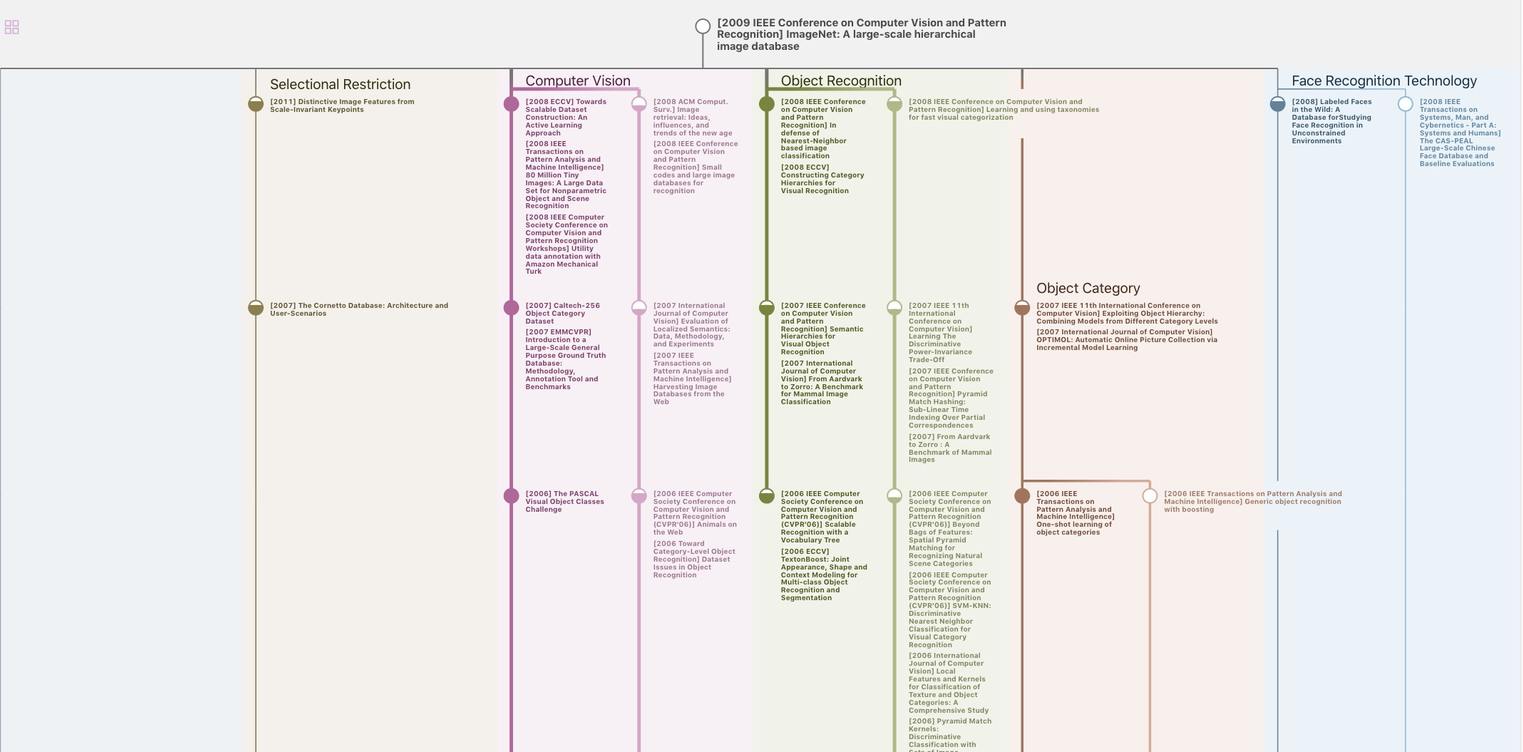
生成溯源树,研究论文发展脉络
Chat Paper
正在生成论文摘要