Modeling Users Preference Dynamics and Side Information in Recommender Systems.
IEEE Trans. Systems, Man, and Cybernetics: Systems(2016)
摘要
In recommender systems user preferences can be fairly dynamic, as users tend to exploit a wide range of items and modify their tastes accordingly over time. In this paper, we model user-item interactions over time using a tensor that has time as a dimension (mode). To account for the fact that user preferences change individually, we propose a new measure of user-preference dynamics (UPD) that captures the rate with which the current preferences of each user have been shifted. UPD shows the variability in how users interact with items in recommender systems. We generate recommendations based on a tensor factorization technique, where the importance of past user preferences are weighted according to their UPD values, that is, higher UPD values downweigh more past user preferences. Additionally, we exploit users’ side data, such as demographics, which improve the accuracy of recommendations based on a coupled tensor-matrix factorization scheme. Our empirical evaluation uses two real benchmark datasets from the social media platforms Last.fm and MovieLens, containing users’ history records pertaining to listening to songs and viewing movies, respectively. We demonstrate that in both datasets, there are users with a varying level of dynamics, expressed by the UPD metric. Our experimental results show that the proposed method outperforms several baselines, by taking into account both dynamics and side data of users.
更多查看译文
关键词
Tensile stress,Recommender systems,Motion pictures,Optimization,Approximation methods,Collaboration,Music
AI 理解论文
溯源树
样例
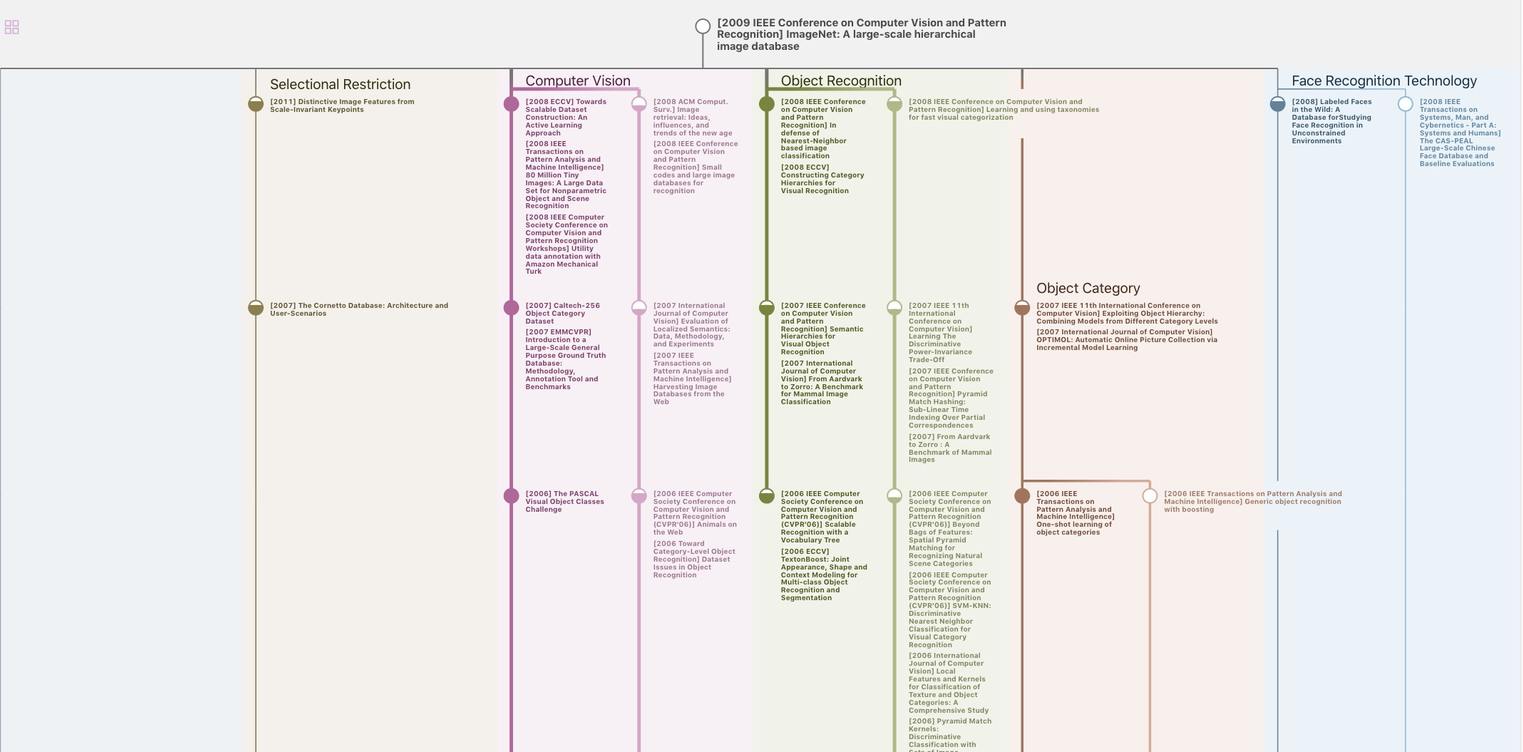
生成溯源树,研究论文发展脉络
Chat Paper
正在生成论文摘要