Two feature weighting approaches for naive Bayes text classifiers.
Knowledge-Based Systems(2016)
摘要
This paper works on feature weighting approaches for naive Bayes text classifiers. Almost all existing feature weighting approaches for naive Bayes text classifiers have some defects: limited improvement to classification performance of naive Bayes text classifiers or sacrificing the simplicity and execution time of the final models. In fact, feature weighting is not new for machine learning community, and many researchers have made fruitful efforts in the field of feature weighting. This paper reviews some simple and efficient feature weighting approaches designed for standard naive Bayes classifiers, and adapts them for naive Bayes text classifiers. As a result, this paper proposes two adaptive feature weighting approaches for naive Bayes text classifiers. Experimental results based on benchmark and real-world data show that, compared to their competitors, our feature weighting approaches show higher classification accuracy, yet at the same time maintain the simplicity and lower execution time of the final models.
更多查看译文
关键词
Naive Bayes text classifiers,Feature weighting,Gain ratio,Decision tree
AI 理解论文
溯源树
样例
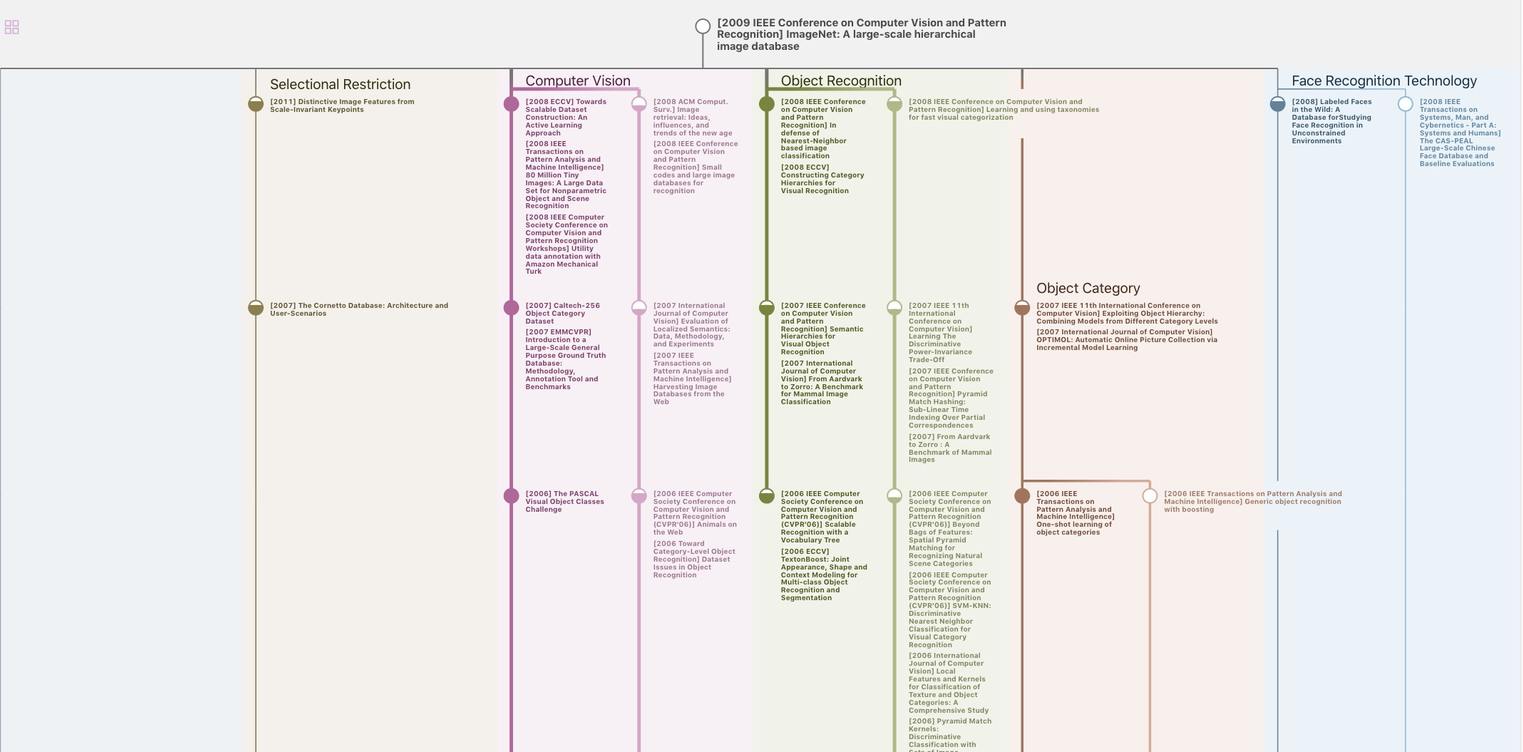
生成溯源树,研究论文发展脉络
Chat Paper
正在生成论文摘要