Dynamic optimization of fuzzy cognitive maps for time series forecasting.
Knowl.-Based Syst.(2016)
摘要
In this paper we propose a new approach to learning fuzzy cognitive maps (FCMs) as a predictive model for time series forecasting. The first contribution of this paper is the dynamic optimization of the FCM structure, i.e., we propose to select concepts involved in the FCM model before every prediction is made. In addition, the FCM transformation function together with the corresponding parameters are proposed to be optimized dynamically. Finally, the FCM weights are learned. In this way, the entire FCM model is learned in a completely new manner, i.e., it is continuously adapted to the current local characteristics of the forecasted time series. To optimize all of the aforementioned elements, we apply and compare 5 different population-based algorithms: genetic, particle swarm optimization, simulated annealing, artificial bee colony and differential evolution. For the evaluation of the proposed approach we use 11 publicly available data sets. The results of comparative experiments provide evidence that our approach offers a competitive forecasting method that outperforms many state-of-the-art forecasting models. We recommend to use our FCM-based approach for the forecasting of time series that are linear and tend to be trend stationary.
更多查看译文
关键词
Fuzzy cognitive maps,Forecasting time series,Machine learning
AI 理解论文
溯源树
样例
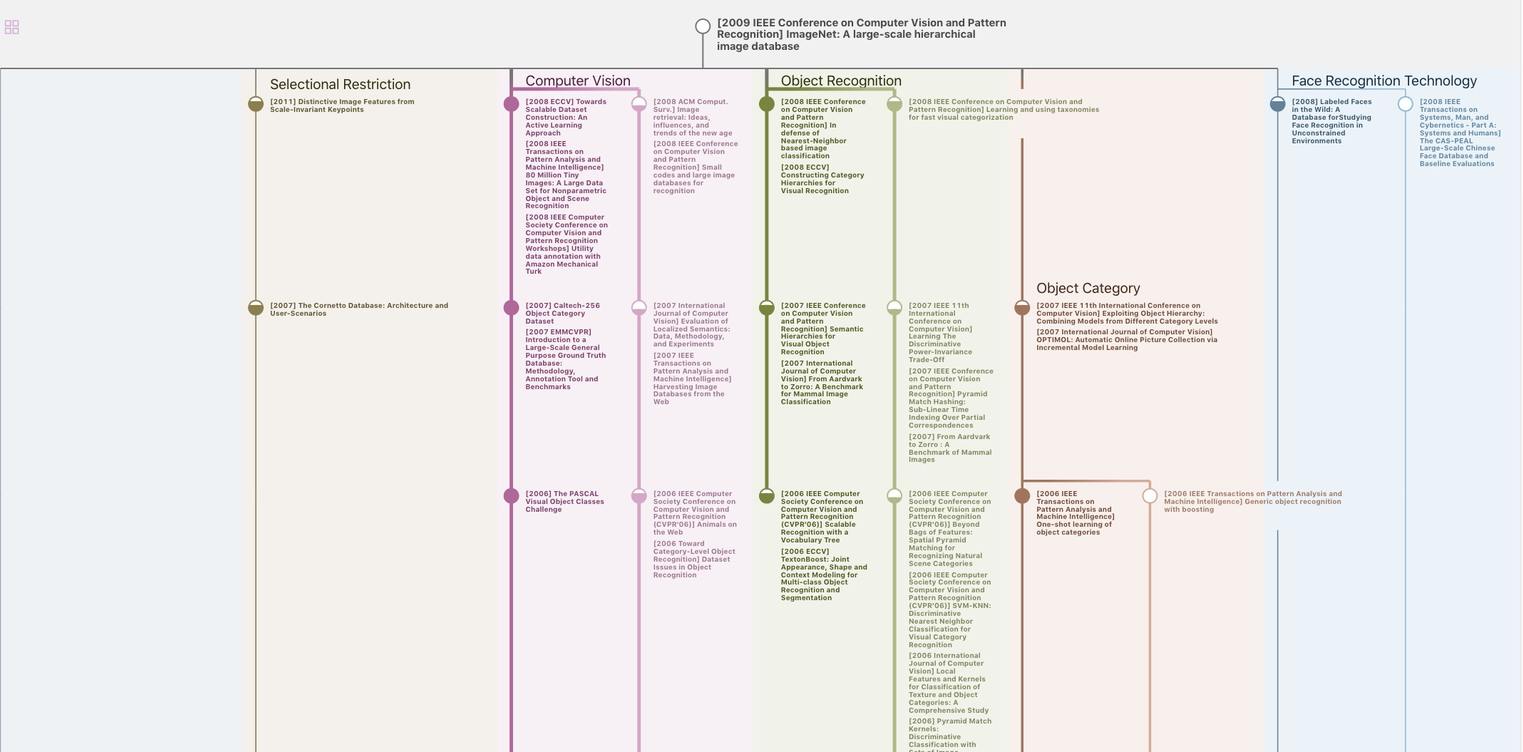
生成溯源树,研究论文发展脉络
Chat Paper
正在生成论文摘要