Meta-cognitive online sequential extreme learning machine for imbalanced and concept-drifting data classification.
Neural Networks(2016)
摘要
In this paper, a meta-cognitive online sequential extreme learning machine (MOS-ELM) is proposed for class imbalance and concept drift learning. In MOS-ELM, meta-cognition is used to self-regulate the learning by selecting suitable learning strategies for class imbalance and concept drift problems. MOS-ELM is the first sequential learning method to alleviate the imbalance problem for both binary class and multi-class data streams with concept drift. In MOS-ELM, a new adaptive window approach is proposed for concept drift learning. A single output update equation is also proposed which unifies various application specific OS-ELM methods. The performance of MOS-ELM is evaluated under different conditions and compared with methods each specific to some of the conditions. On most of the datasets in comparison, MOS-ELM outperforms the competing methods.
更多查看译文
关键词
Concept drift,Extreme learning machine,Meta-cognition,Multi-class imbalance,Sequential learning
AI 理解论文
溯源树
样例
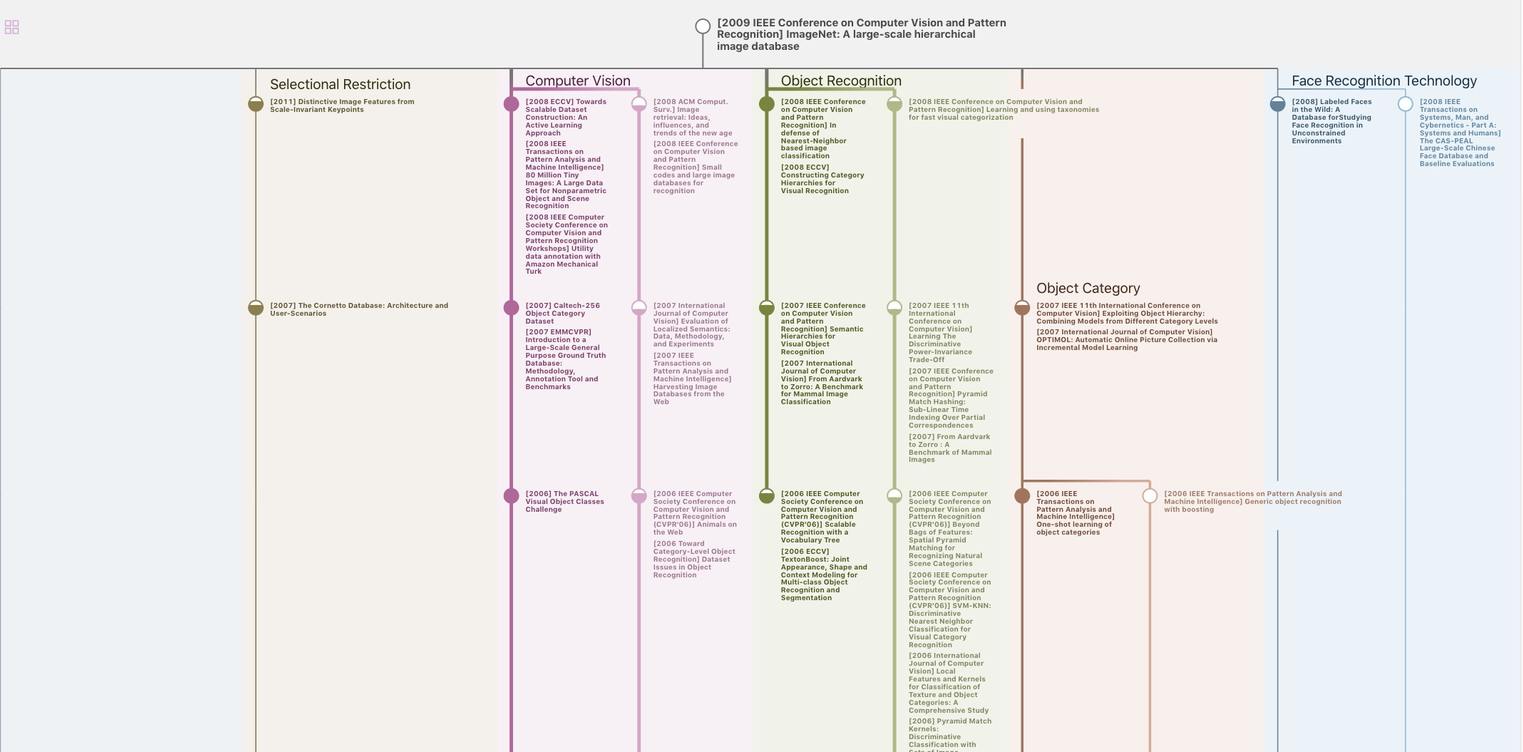
生成溯源树,研究论文发展脉络
Chat Paper
正在生成论文摘要