Improved Manifold Learning With Competitive Hebbian Rule
2015 International Joint Conference on Neural Networks (IJCNN)(2015)
摘要
Manifold Learning methods aim to find meaningful low-dimensional structures hidden in their high-dimensional observations. Recently, they are faced with critical problems of how to reduce computational and space complexity in big data applications, how to determine neighborhood size adaptive to different data sets and how to deal with new observations in an out-of-sample mode. This paper presents a new method called TLOE (Topology Learning and Out-of-sample Embedding) to deal with the above three problems. TLOE uses the competitive Hebbian rule to construct the topology preserving network on a given manifold. It is capable of: 1) automatical selection of the right number and position of landmarks, 2) adaptive determination of neighborhood sizes for landmarks and 3) online embedding of new observations. Experiments on both synthetic and real-world data sets show its promising results.
更多查看译文
关键词
manifold learning,competitive Hebbian rule,low-dimensional structures,high-dimensional observations,computational complexity,space complexity,Big Data applications,out-of-sample mode,TLOE,topology learning and out-of-sample embedding,topology preserving network,landmarks position,adaptive determination,neighborhood sizes,online embedding,synthetic data sets,real-world data sets
AI 理解论文
溯源树
样例
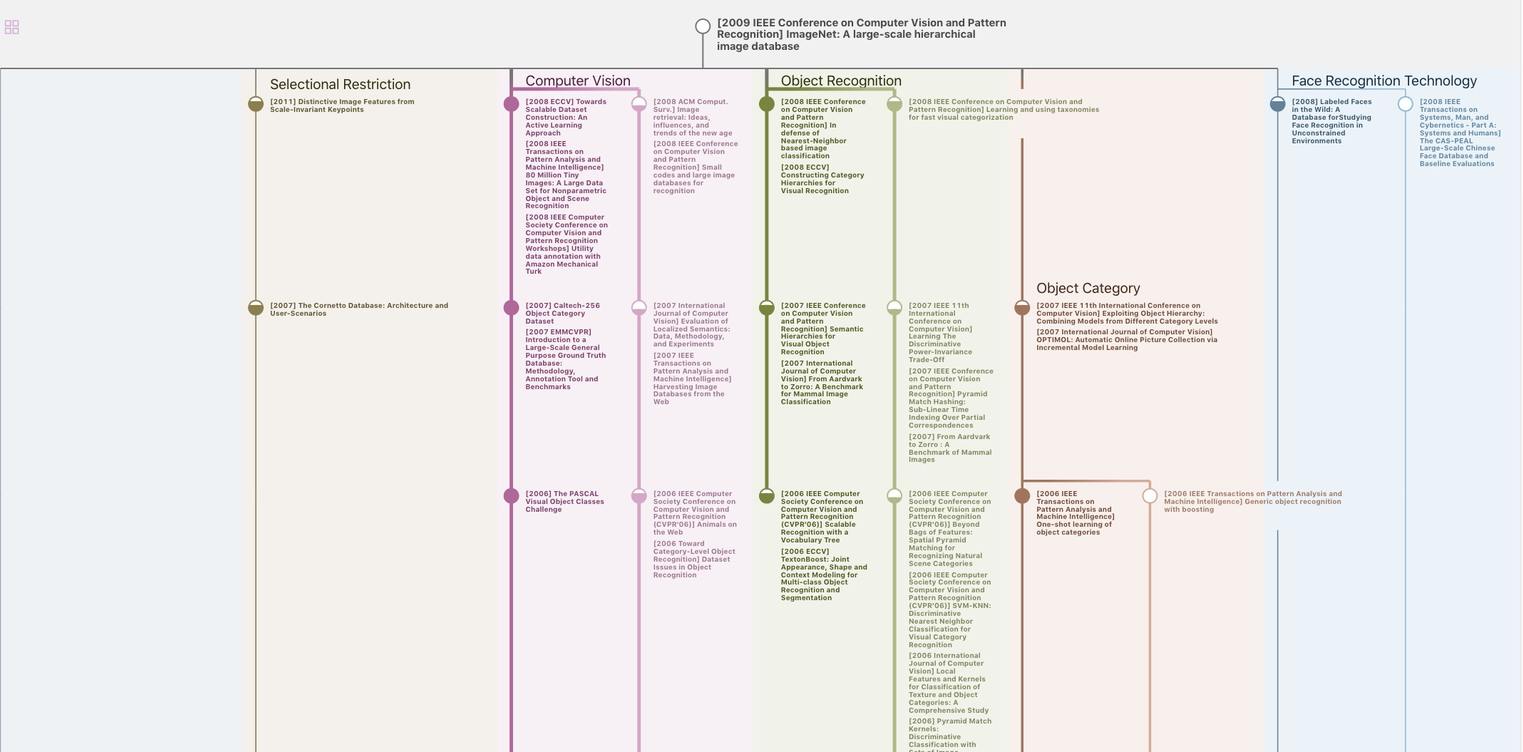
生成溯源树,研究论文发展脉络
Chat Paper
正在生成论文摘要