The Neural-Sift Feature Descriptor For Visual Vocabulary Object Recognition
2015 International Joint Conference on Neural Networks (IJCNN)(2015)
摘要
Recognizing the semantic content of an image is a challenging problem in computer vision. Many researchers attempt to apply local image descriptors to extract features from an image, but choosing the best type of feature to use is still an open problem. Some of these systems are only trained once using a fixed descriptor, like the Scale Invariant Feature Transform (SIFT). In most cases these algorithms show good performance, but they do not learn from their mistakes once training is completed. In this paper a continuous deep neural network feedback system is proposed which consists of an adaptive neural network feature descriptor, the bag of visual words approach and a neural classifier. Two initialization methods for the neural network feature descriptor were compared, one where it was trained on SIFT descriptor output and one where it was randomly initialized. After initial training, the system propagates the classification error from the neural network classifier through the entire pipeline, updating not only the classifier itself, but also the type of features to extract. Results show that for both initialization methods the feedback system increased accuracy substantially when regular training was not able to increase it any further. The proposed neural-SIFT feature descriptor performs better than the SIFT descriptor itself even with a limited number of training instances. Initializing on an existing feature descriptor is beneficial when not a lot of training samples are available. However, when there are a lot of training samples the system is able to construct a well-performing descriptor, solely based on classifier feedback.
更多查看译文
关键词
neural-SIFT feature descriptor,visual vocabulary object recognition,image semantic content,computer vision,feature extraction,scale invariant feature transform,adaptive neural network feature descriptor,visual words approach,initialization methods,classification error,neural network classifier,training samples,well-performing descriptor,classifier feedback
AI 理解论文
溯源树
样例
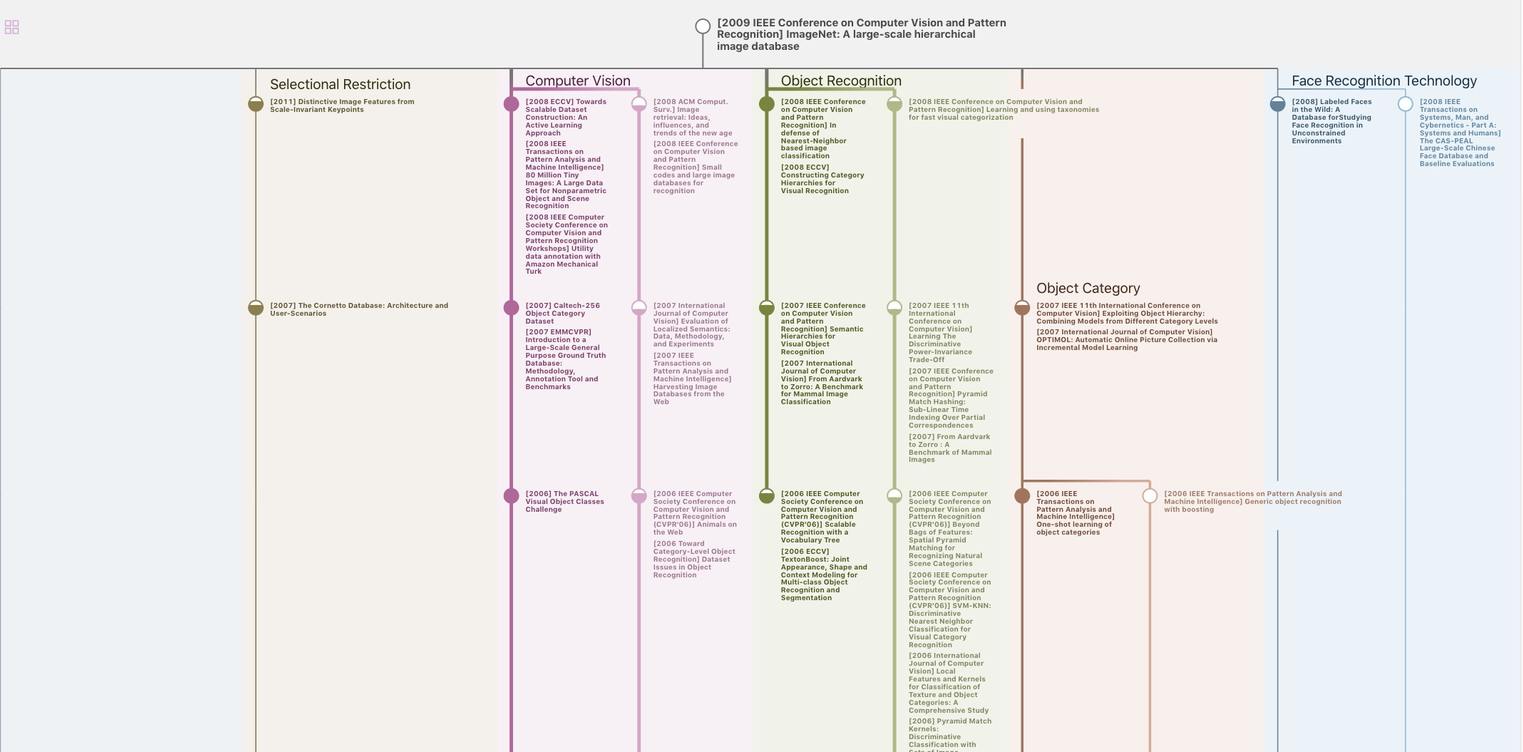
生成溯源树,研究论文发展脉络
Chat Paper
正在生成论文摘要