Position-Aware Listmle: A Sequential Learning Process For Ranking
UAI'14: Proceedings of the Thirtieth Conference on Uncertainty in Artificial Intelligence(2014)
摘要
ListMLE is a state-of-the-art listwise learning-to-rank algorithm, which has been shown to work very well in application. It defines the probability distribution based on Plackett-Luce Model in a top-down style to take into account the position information. However, both empirical contradiction and theoretical results indicate that ListMLE cannot well capture the position importance, which is a key factor in ranking. To amend the problem, this paper proposes a new listwise ranking method, called position-aware ListMLE (p-ListMLE for short). It views the ranking problem as a sequential learning process, with each step learning a subset of parameters which maximize the corresponding stepwise probability distribution. To solve this sequential multi-objective optimization problem, we propose to use linear scalarization strategy to transform it into a single-objective optimization problem, which is efficient for computation. Our theoretical study shows that p-ListMLE is better than ListMLE in statistical consistency with respect to typical ranking evaluation measure NDCG. Furthermore, our experiments on benchmark datasets demonstrate that the proposed method can significantly improve the performance of ListMLE and outperform state-of-the-art listwise learning-to-rank algorithms as well.
更多查看译文
AI 理解论文
溯源树
样例
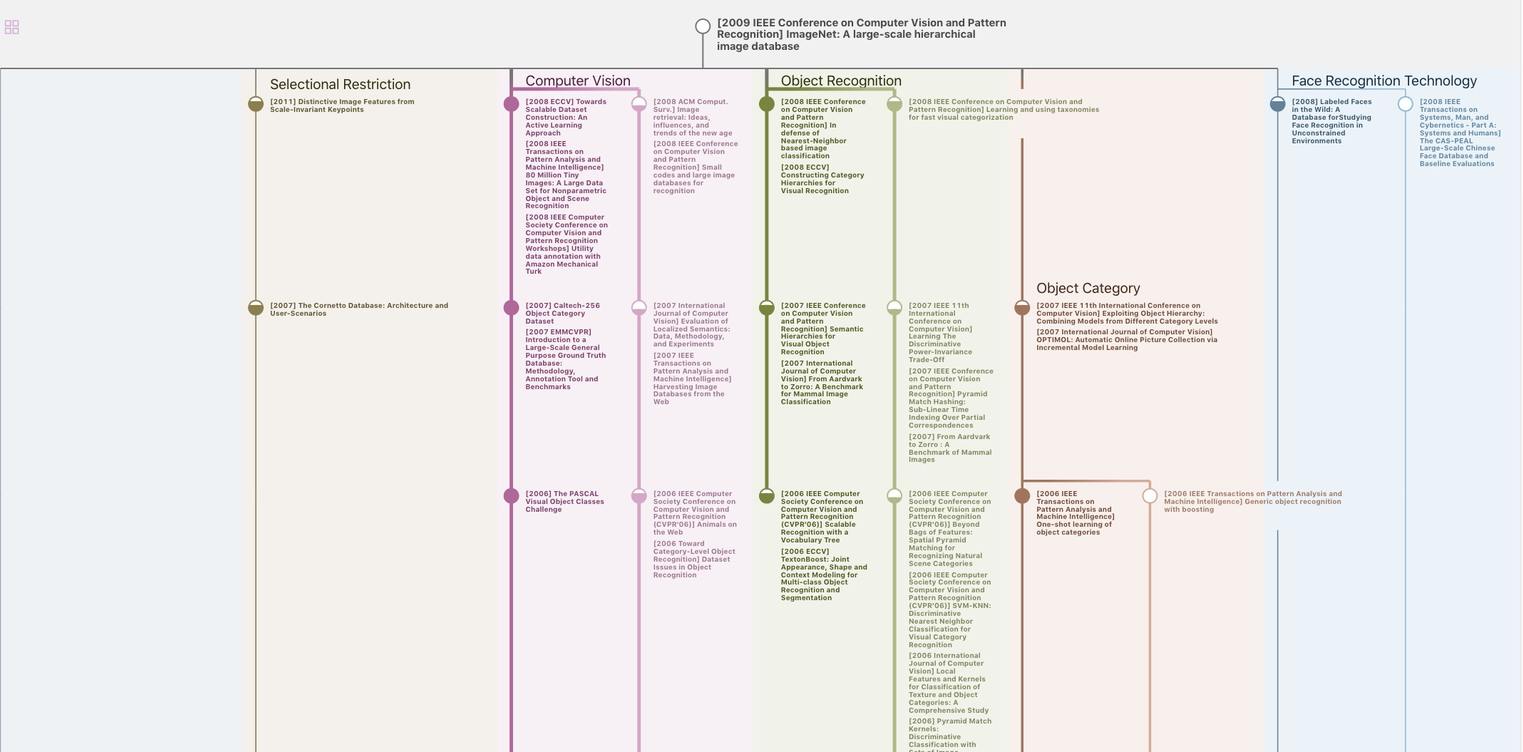
生成溯源树,研究论文发展脉络
Chat Paper
正在生成论文摘要