Inference Complexity In Continuous Time Bayesian Networks
UAI'14: Proceedings of the Thirtieth Conference on Uncertainty in Artificial Intelligence(2014)
摘要
The continuous time Bayesian network (CTBN) enables temporal reasoning by representing a system as a factored, finite-state Markov process. The CTBN uses a traditional Bayesian network (BN) to specify the initial distribution. Thus, the complexity results of Bayesian networks also apply to CTBNs through this initial distribution. However, the question remains whether propagating the probabilities through time is, by itself, also a hard problem. We show that exact and approximate inference in continuous time Bayesian networks is NP-hard even when the initial states are given.
更多查看译文
AI 理解论文
溯源树
样例
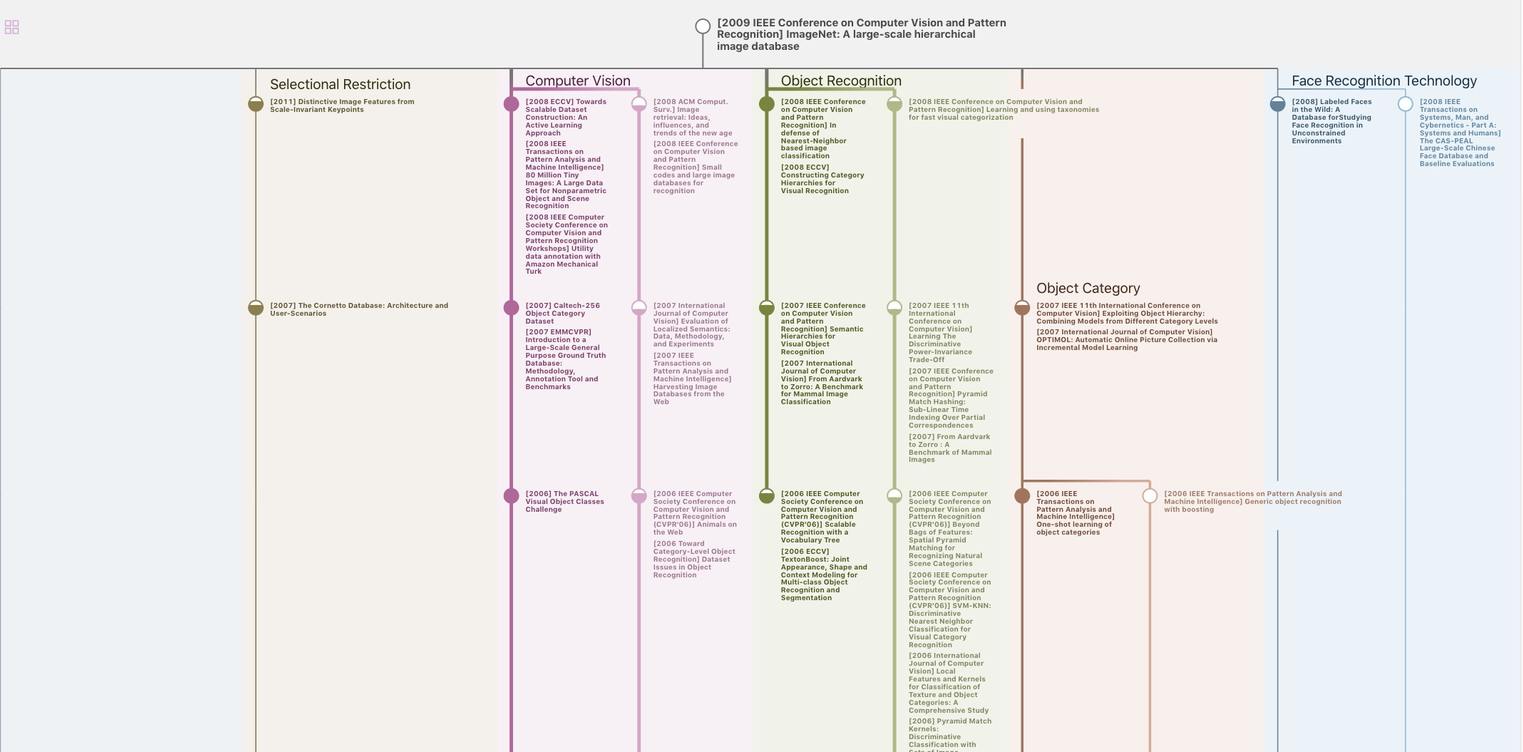
生成溯源树,研究论文发展脉络
Chat Paper
正在生成论文摘要