Brand-Related Twitter Sentiment Analysis Using Feature Engineering and the Dynamic Architecture for Artificial Neural Networks
PROCEEDINGS OF THE 49TH ANNUAL HAWAII INTERNATIONAL CONFERENCE ON SYSTEM SCIENCES (HICSS 2016)(2016)
摘要
We present an approach to brand-related Twitter sentiment analysis using feature engineering and the Dynamic Architecture for Artificial Neural Networks (DAN2). The approach addresses challenges associated with the unique characteristics of the Twitter language, and the recall of mild sentiment expressions that are of interest to brand management practitioners. We demonstrate the effectiveness of the approach on a Starbucks brand-related Twitter data set. The feature engineering produced a final tweet feature representation consisting of only seven dimensions, with greater feature density. Two sets of experiments were conducted in three-class and five-class tweet sentiment classification. We compare the proposed approach to the performances of two state-of-the-art Twitter sentiment analysis systems from the academic and commercial domains. The results indicate that the approach outperforms these state-of-the-art systems in both three-class and five-class tweet sentiment classification by wide margins, with classification accuracies above 80% and excellent recall of mild sentiment tweets.
更多查看译文
关键词
brand-related Twitter sentiment analysis,feature engineering,dynamic architecture,tweet sentiment classification,tweet feature representation,Starbucks brand-related Twitter data set,DAN2,artificial neural network
AI 理解论文
溯源树
样例
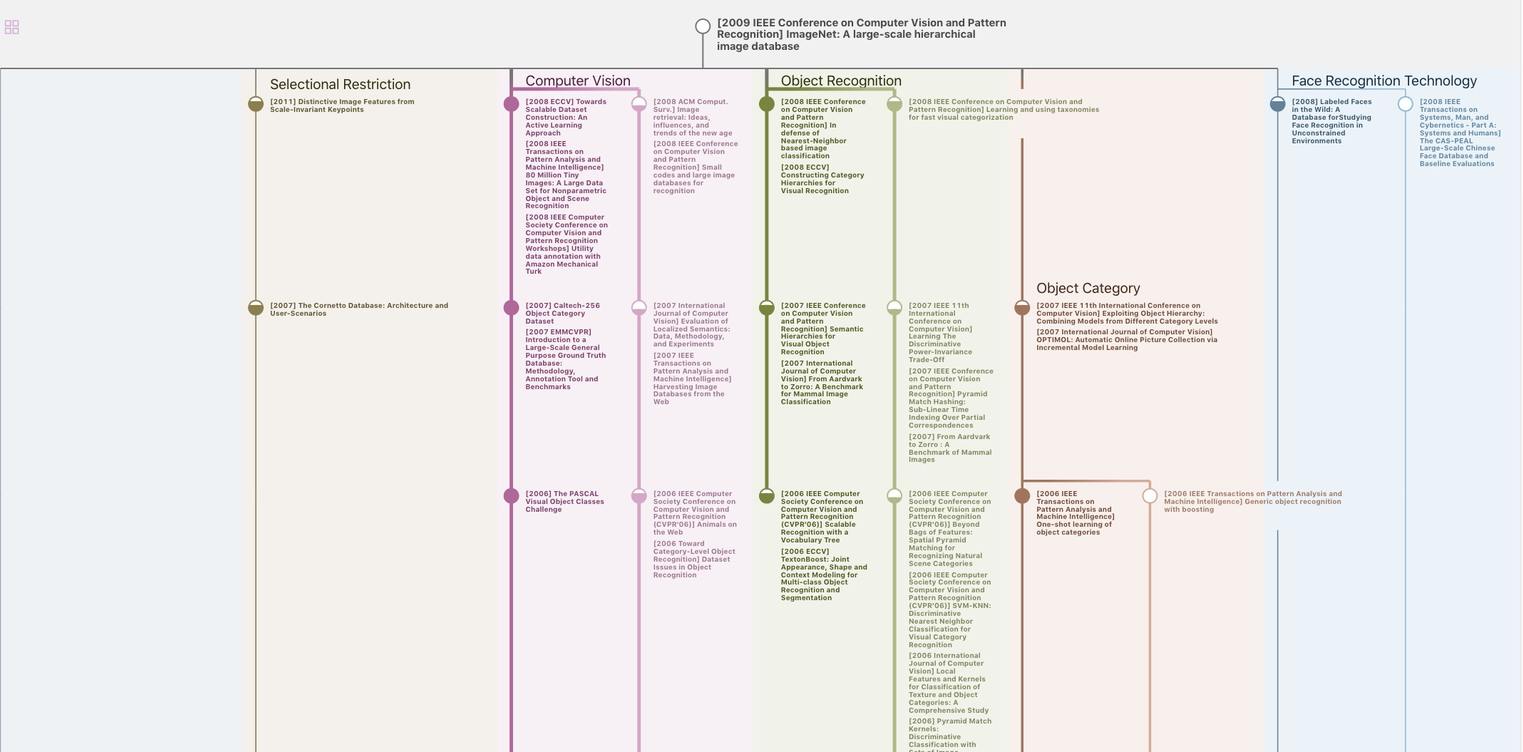
生成溯源树,研究论文发展脉络
Chat Paper
正在生成论文摘要