Towards an Efficient Computational Framework for Guiding Surgical Resection through Intra-operative Endo-microscopic Pathology.
MICCAI(2015)
摘要
Precise detection and surgical resection of the tumors during an operation greatly increases the chance of the overall procedure efficacy. Emerging experimental in-vivo imaging technologies such as Confocal Laser Endomicroscopy CLE, could potentially assist surgeons to examine brain tissues on histological scale in real-time during the operation. However, it is a challenging task for neurosurgeons to interpret these images in real-time, primarily due to the low signal to noise ratio and variability in the patterns expressed within these images by various examined tissue types. In this paper, we present a comprehensive computational framework capable of automatic brain tumor classification in real-time. Specifically, our contributions include: a an end-to-end computational pipeline where a variety of the feature extraction methods, encoding schemes, and classification algorithms can be readily deployed, b thorough evaluation of state-of-the-art low-level image features and popular encoding techniques in context of CLE imagery, and finally, c A highly optimized feature pooling method based on codeword proximity. The proposed system can effectively classify two types of commonly diagnosed brain tumors in CLE sequences captured in real-time with close to 90% accuracy. Extensive experiments on a dataset of 117 videos demonstrate the efficacy of our system.
更多查看译文
AI 理解论文
溯源树
样例
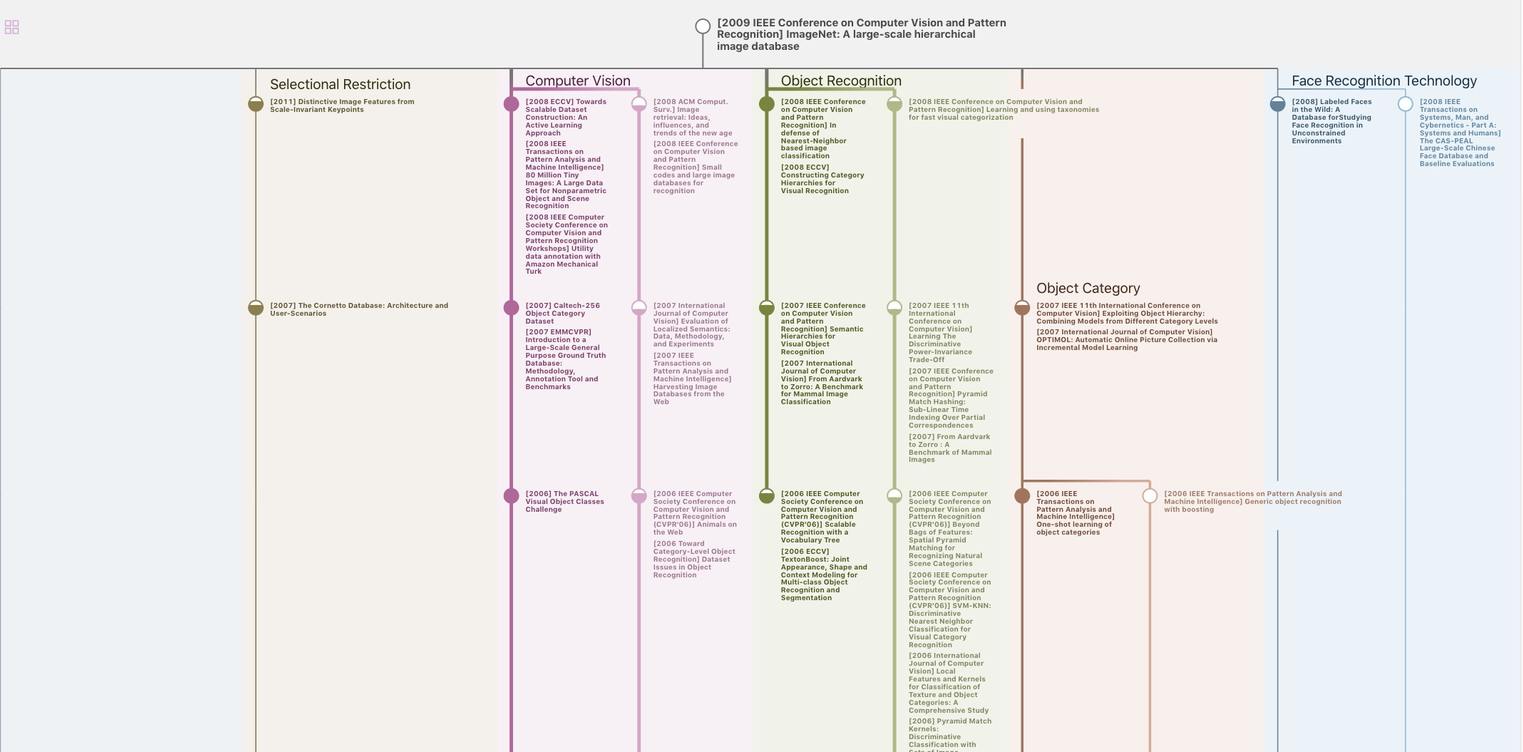
生成溯源树,研究论文发展脉络
Chat Paper
正在生成论文摘要