Counterfactual Reasoning about Intent for Interactive Navigation in Dynamic Environments
2015 IEEE/RSJ INTERNATIONAL CONFERENCE ON INTELLIGENT ROBOTS AND SYSTEMS (IROS)(2016)
摘要
Many modern robotics applications require robots to function autonomously in dynamic environments including other decision making agents, such as people or other robots. This calls for fast and scalable interactive motion planning. This requires models that take into consideration the other agent's intended actions in one's own planning. We present a real-time motion planning framework that brings together a few key components including intention inference by reasoning counterfactually about potential motion of the other agents as they work towards different goals. By using a light-weight motion model, we achieve efficient iterative planning for fluid motion when avoiding pedestrians, in parallel with goal inference for longer range movement prediction. This inference framework is coupled with a novel distributed visual tracking method that provides reliable and robust models for the current belief-state of the monitored environment. This combined approach represents a computationally efficient alternative to previously studied policy learning methods that often require significant offline training or calibration and do not yet scale to densely populated environments. We validate this framework with experiments involving multi-robot and human-robot navigation. We further validate the tracker component separately on much larger scale unconstrained pedestrian data sets.
更多查看译文
关键词
counterfactual reasoning,interactive navigation,dynamic environments,interactive motion planning,real-time motion planning framework,intention inference,light-weight motion model,iterative planning,fluid motion,pedestrian avoidance,goal inference,range movement prediction,distributed visual tracking method,multirobot,human-robot navigation,unconstrained pedestrian data sets
AI 理解论文
溯源树
样例
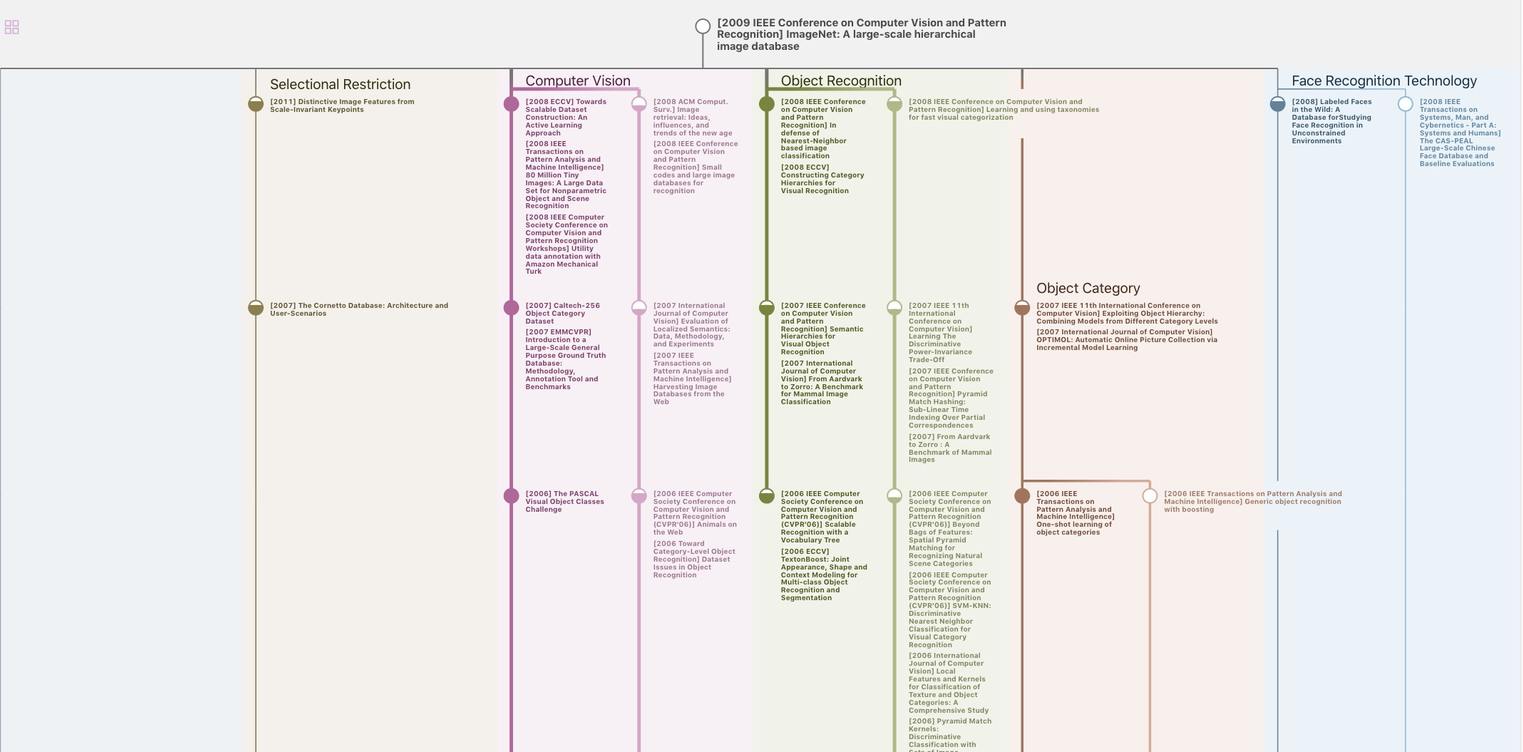
生成溯源树,研究论文发展脉络
Chat Paper
正在生成论文摘要