Nicp: Dense Normal Based Point Cloud Registration
2015 IEEE/RSJ International Conference on Intelligent Robots and Systems (IROS)(2015)
摘要
In this paper we present a novel on-line method to recursively align point clouds. By considering each point together with the local features of the surface (normal and curvature), our method takes advantage of the 3D structure around the points for the determination of the data association between two clouds. The algorithm relies on a least squares formulation of the alignment problem, that minimizes an error metric depending on these surface characteristics. We named the approach Normal Iterative Closest Point (NICP in short). Extensive experiments on publicly available benchmark data show that NICP outperforms other state-of-the-art approaches.
更多查看译文
关键词
NICP,dense normal based point cloud registration,3D structure,data association,least squares formulation,alignment problem,error metric,normal iterative closest point
AI 理解论文
溯源树
样例
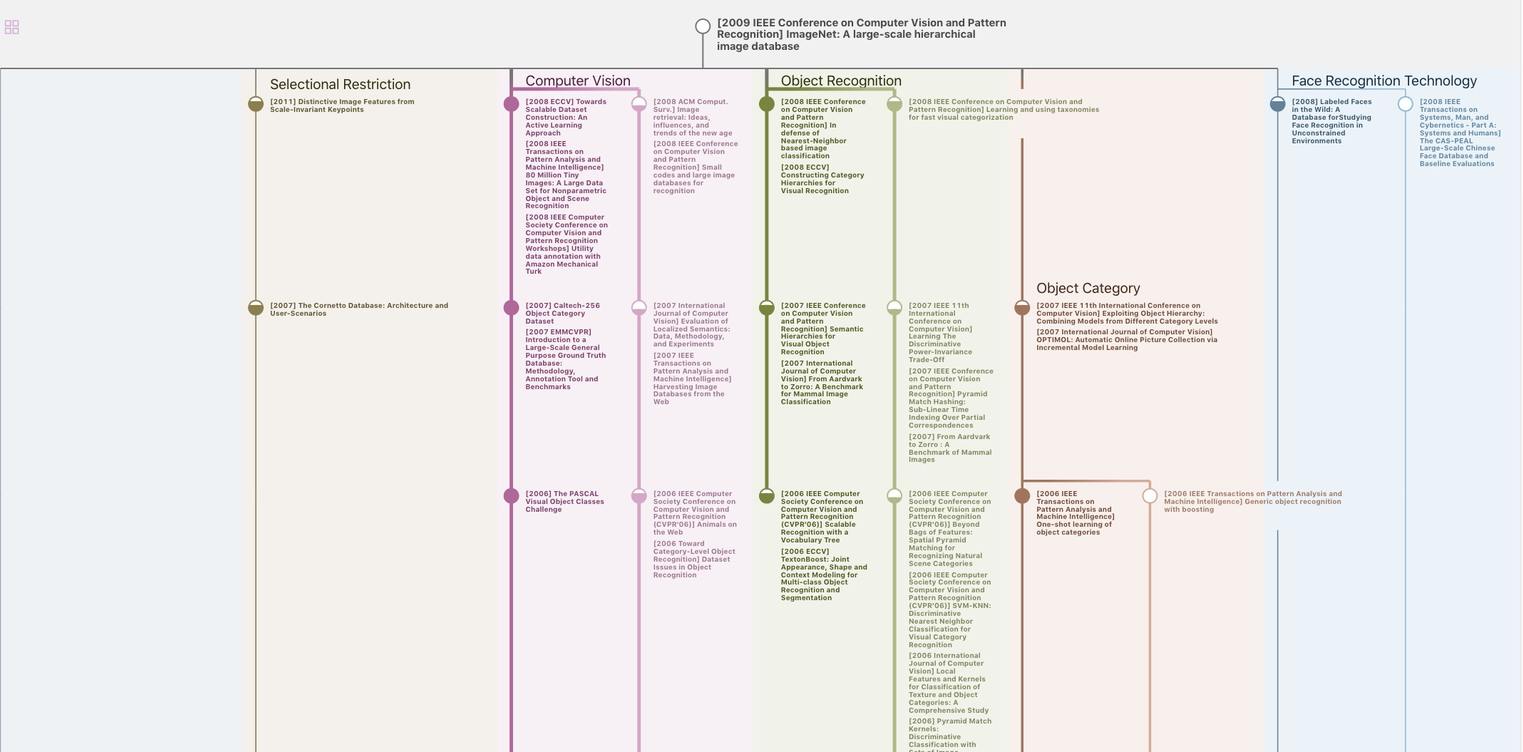
生成溯源树,研究论文发展脉络
Chat Paper
正在生成论文摘要