Proximal Alternating Direction Method Of Multipliers For Distributed Optimization On Weighted Graphs
2015 54TH IEEE CONFERENCE ON DECISION AND CONTROL (CDC)(2015)
摘要
Distributed optimization aims to optimize a global objective function formed by summation of coupled local functions over a graph via only local communication and computation. In this paper, we develop a weighted proximal Alternating Direction Method of Multipliers (ADMM) for distributed optimization using graph structure. We give a bound on the rate of convergence of the algorithm in terms of the graph parameters. This fully distributed, single-loop algorithm allows simultaneous updates and can be viewed as a generalization of existing algorithms. More importantly, we achieve faster convergence by jointly designing graph weights and algorithm parameters. Numerical examples demonstrate that designing the graph weights and proximal term can considerably improve the algorithm performance.
更多查看译文
关键词
Optimization,Algorithm design and analysis,Convergence,Laplace equations,Standards,Linear programming,Symmetric matrices
AI 理解论文
溯源树
样例
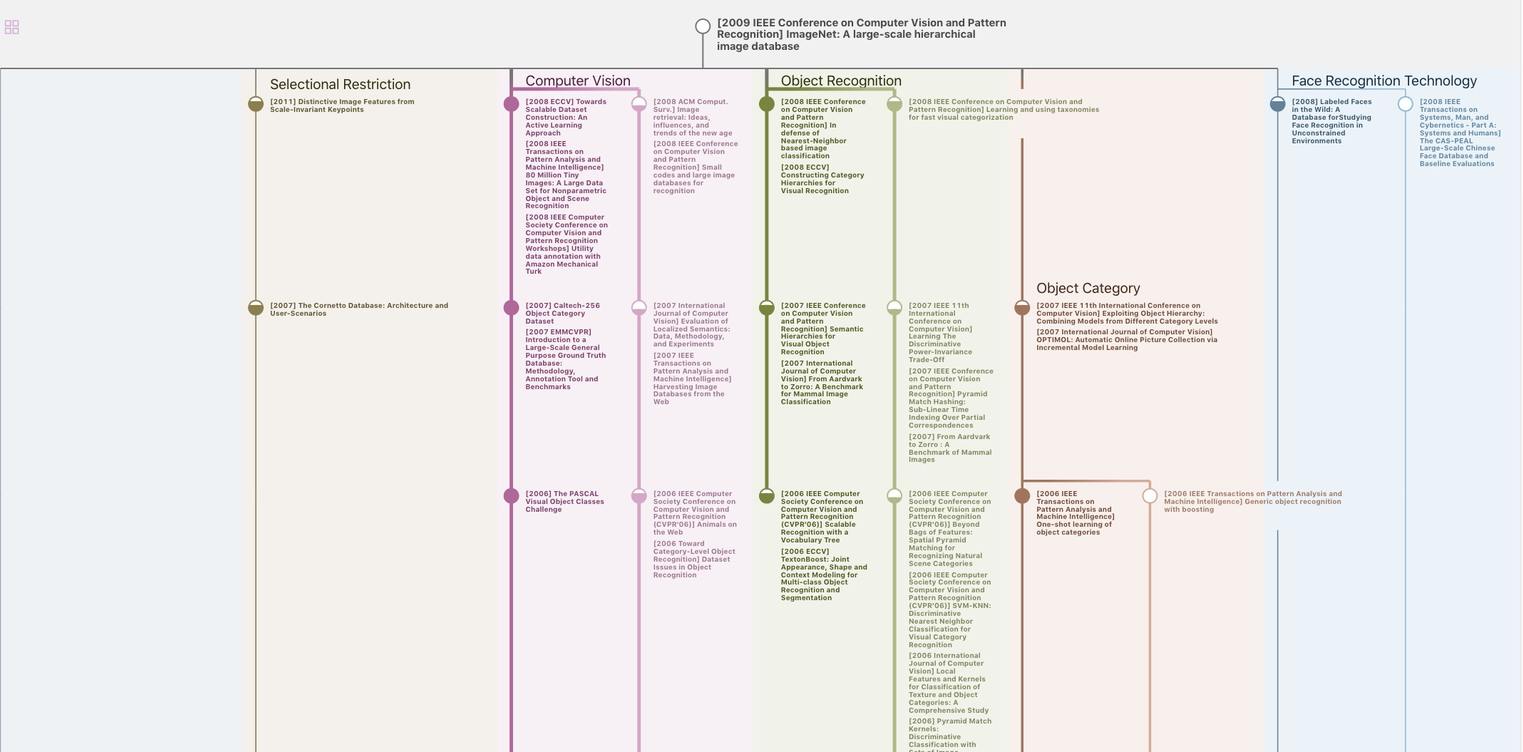
生成溯源树,研究论文发展脉络
Chat Paper
正在生成论文摘要