Leveraging Dual-Observable Inputfor Fine-Grained Thumb Interaction Using Forearm Emg
UIST(2015)
摘要
We introduce the first forearm-based EMG input system that can recognize fine-grained thumb gestures, including left swipes, right swipes, taps, long presses, and more complex thumb motions. EMG signals for thumb motions sensed from the forearm are quite weak and require significant training data to classify. We therefore also introduce a novel approach for minimally-intrusive collection of labeled training data for always-available input devices. Our dual-observable input approach is based on the insight that interaction observed by multiple devices allows recognition by a primary device (e.g., phone recognition of a left swipe gesture) to create labeled training examples for another (e.g., forearm-based EMG data labeled as a left swipe). We implement a wearable prototype with dry EMG electrodes, train with labeled demonstrations from participants using their own phones, and show that our prototype can recognize common fine-grained thumb gestures and user-defined complex gestures.
更多查看译文
关键词
Always-Available Interaction, Dual-Observable Input, EMG
AI 理解论文
溯源树
样例
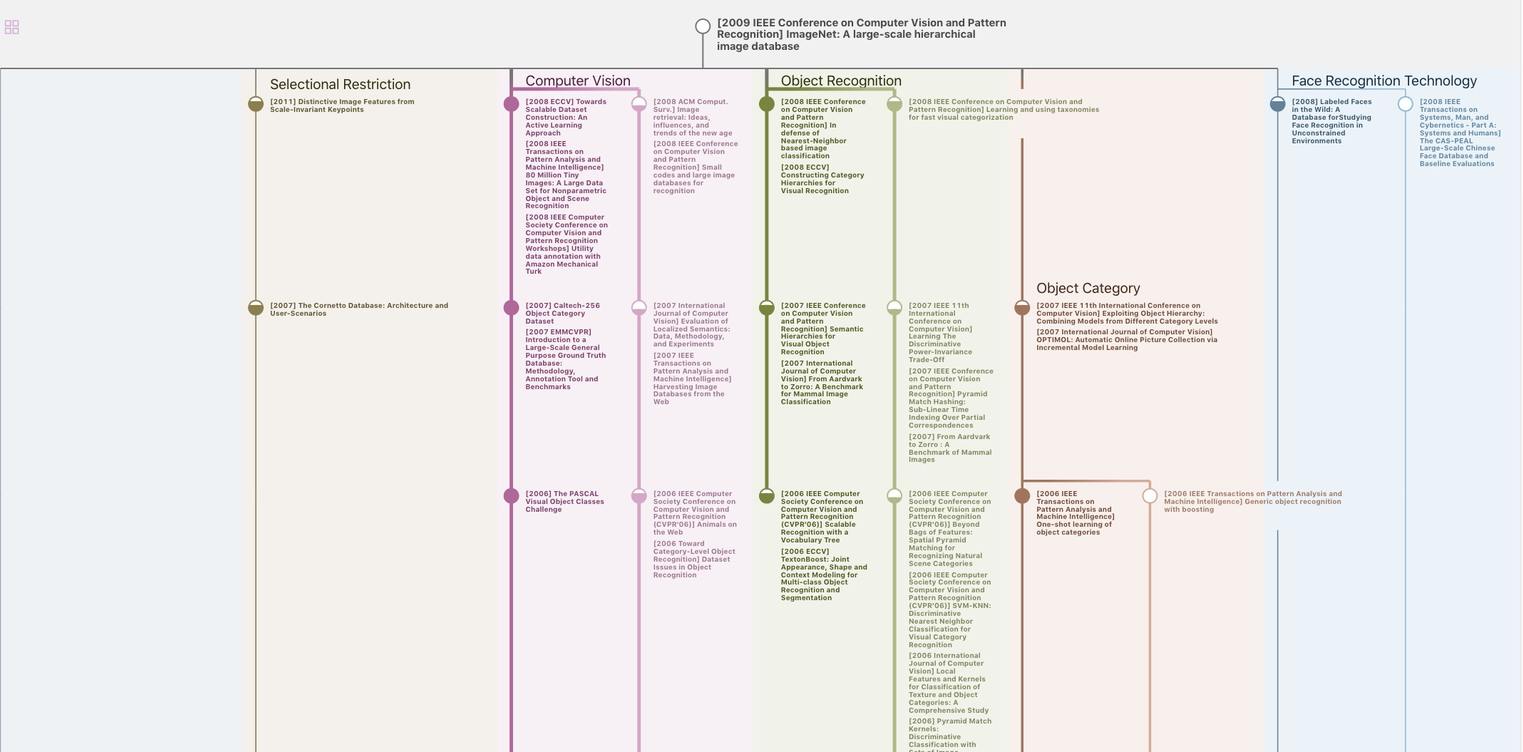
生成溯源树,研究论文发展脉络
Chat Paper
正在生成论文摘要