Multiple Label Prediction For Image Annotation With Multiple Kernel Correlation Models
2009 IEEE COMPUTER SOCIETY CONFERENCE ON COMPUTER VISION AND PATTERN RECOGNITION WORKSHOPS (CVPR WORKSHOPS 2009), VOLS 1 AND 2(2009)
摘要
Image annotation is a challenging task that allows to correlate text keywords with an image. In this paper we address the problem of image annotation using Kernel Multiple Linear Regression model. Multiple Linear Regression (MLR) model reconstructs image caption from an image by performing a linear transformation of an image into some semantic space, and then recovers the caption by performing another linear transformation from the semantic space into the label space. The model is trained so that model parameters minimize the error of reconstruction directly. This model is related to Canonical Correlation Analysis (CCA) which maps both images and caption into the semantic space to minimize the distance of mapping in the semantic space. Kernel trick is then used for the MLR resulting in Kernel Multiple Linear Regression model. The solution to KMLR is a solution to the generalized eigen-value problem, related to KCCA (Kernel Canonical Correlation Analysis). We then extend Kernel Multiple Linear Regression and Kernel Canonical Correlation analysis models to multiple kernel setting, to allow various representations of images and captions. We present results for image annotation using Multiple Kernel Learning CCA and MLR on Oliva and Torralba [21] scene recognition that show kernel selection behaviour.
更多查看译文
关键词
image reconstruction,regression analysis,text analysis,canonical correlation analysis,generalized eigen-value problem,image annotation,image caption reconstruction,kernel multiple linear regression model,linear transformation,multiple kernel correlation models,multiple kernel learning,multiple label prediction,text keywords,
AI 理解论文
溯源树
样例
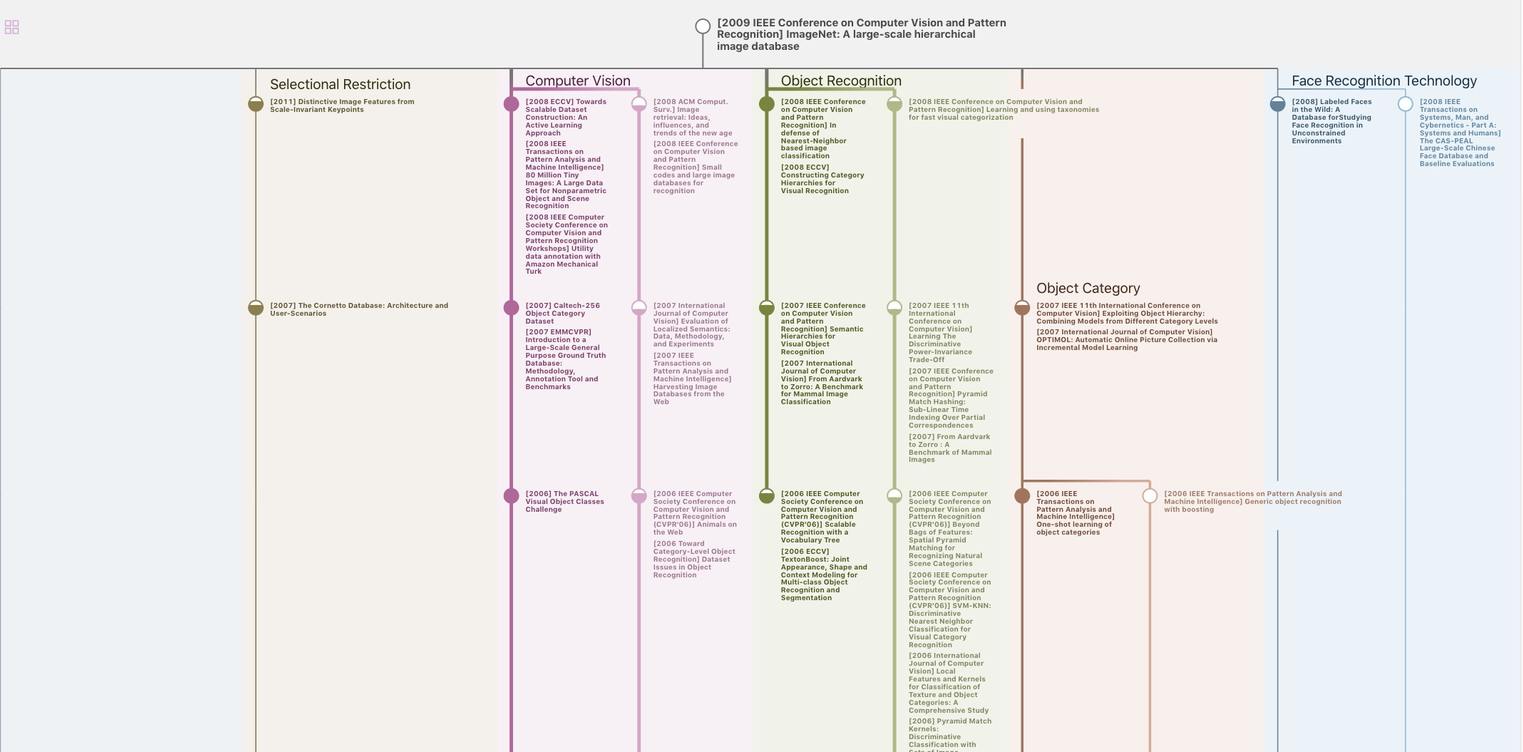
生成溯源树,研究论文发展脉络
Chat Paper
正在生成论文摘要