Zero-Shot Object Recognition By Semantic Manifold Distance
2015 IEEE Conference on Computer Vision and Pattern Recognition (CVPR)(2015)
摘要
Object recognition by zero-shot learning (ZSL) aims to recognise objects without seeing any visual examples by learning knowledge transfer between seen and unseen object classes. This is typically achieved by exploring a semantic embedding space such as attribute space or semantic word vector space. In such a space, both seen and unseen class labels, as well as image features can be embedded (projected), and the similarity between them can thus be measured directly. Existing works differ in what embedding space is used and how to project the visual data into the semantic embedding space. Yet, they all measure the similarity in the space using a conventional distance metric (e.g. cosine) that does not consider the rich intrinsic structure, i.e. semantic manifold, of the semantic categories in the embedding space. In this paper we propose to model the semantic manifold in an embedding space using a semantic class label graph. The semantic manifold structure is used to redefine the distance metric in the semantic embedding,space for more effective ZSL. The proposed semantic manifold distance is computed using a novel absorbing Markov chain process (AMP), which has a very efficient closed-form solution. The proposed new model improves upon and seamlessly unifies various existing ZSL, algorithms. Extensive experiments on both the large scale ImageNet dataset and the widely used Animal with Attribute (AwA) dataset show that our model outperforms significantly the state-of-the-arts.
更多查看译文
关键词
zero-shot object recognition,semantic manifold distance,zero-shot learning,ZSL,semantic embedding space,attribute space,semantic word vector space,semantic class label graph,absorbing Markov chain process,AMP,ImageNet dataset,animal with attribute dataset,AwA dataset
AI 理解论文
溯源树
样例
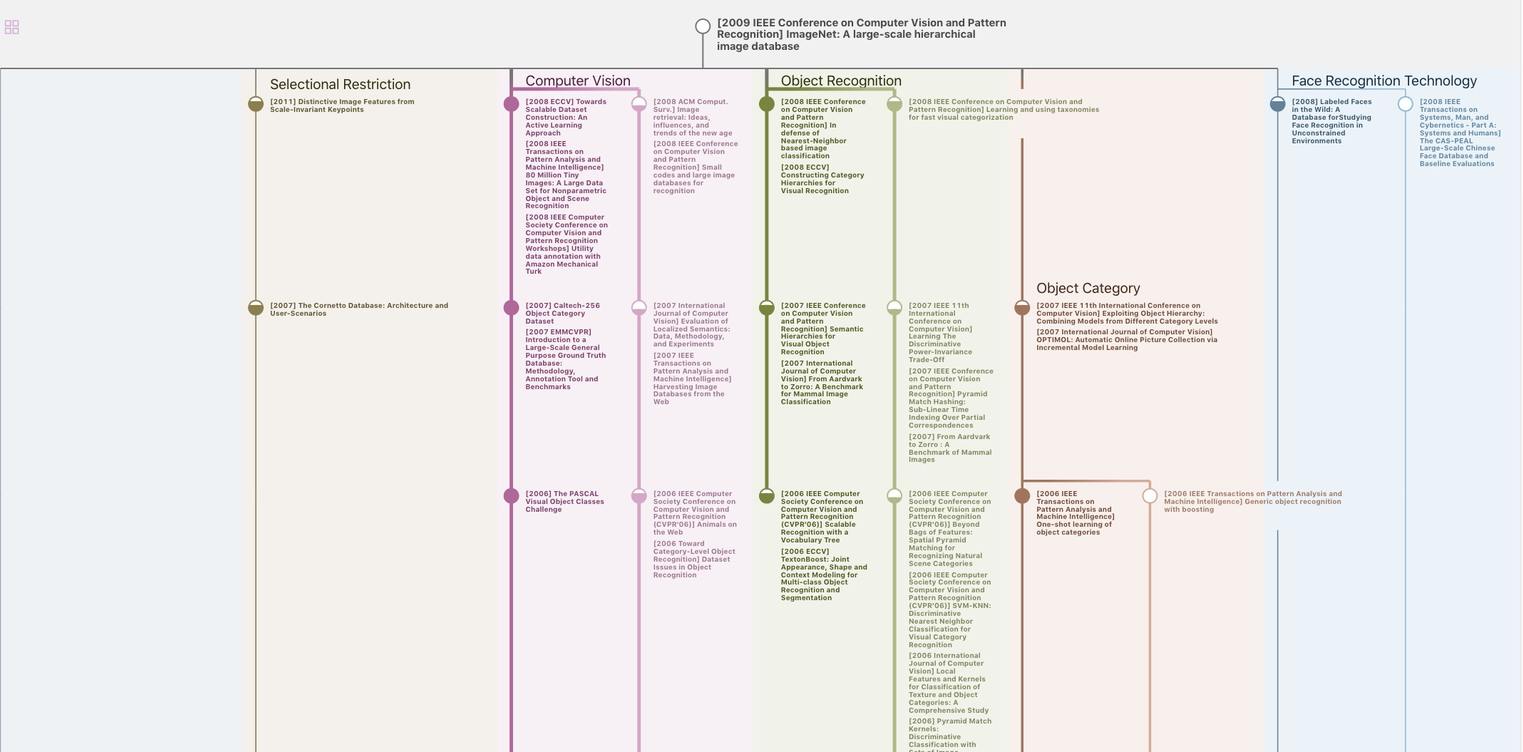
生成溯源树,研究论文发展脉络
Chat Paper
正在生成论文摘要