On-the-fly hand detection training with application in egocentric action recognition
2015 IEEE Conference on Computer Vision and Pattern Recognition Workshops (CVPRW)(2015)
摘要
We propose a novel approach to segment hand regions in egocentric video that requires no manual labeling of training samples. The user wearing a head-mounted camera is prompted to perform a simple gesture during an initial calibration step. A combination of color and motion analysis that exploits knowledge of the expected gesture is applied on the calibration video frames to automatically label hand pixels in an unsupervised fashion. The hand pixels identified in this manner are used to train a statistical-model-based hand detector. Superpixel region growing is used to perform segmentation refinement and improve robustness to noise. Experiments show that our hand detection technique based on the proposed on-the-fly training approach significantly outperforms state-of-the-art techniques with respect to accuracy and robustness on a variety of challenging videos. This is due primarily to the fact that training samples are personalized to a specific user and environmental conditions. We also demonstrate the utility of our hand detection technique to inform an adaptive video sampling strategy that improves both computational speed and accuracy of egocentric action recognition algorithms. Finally, we offer an egocentric video dataset of an insulin self-injection procedure with action labels and hand masks that can serve towards future research on both hand detection and egocentric action recognition.
更多查看译文
关键词
on-the-fly hand detection training,egocentric action recognition algorithm,hand region segmentation,egocentric video,head-mounted camera,motion analysis,color analysis,statistical-model-based hand detector,hand detection technique,adaptive video sampling strategy,insulin self-injection procedure,unsupervised fashion,superpixel region growing
AI 理解论文
溯源树
样例
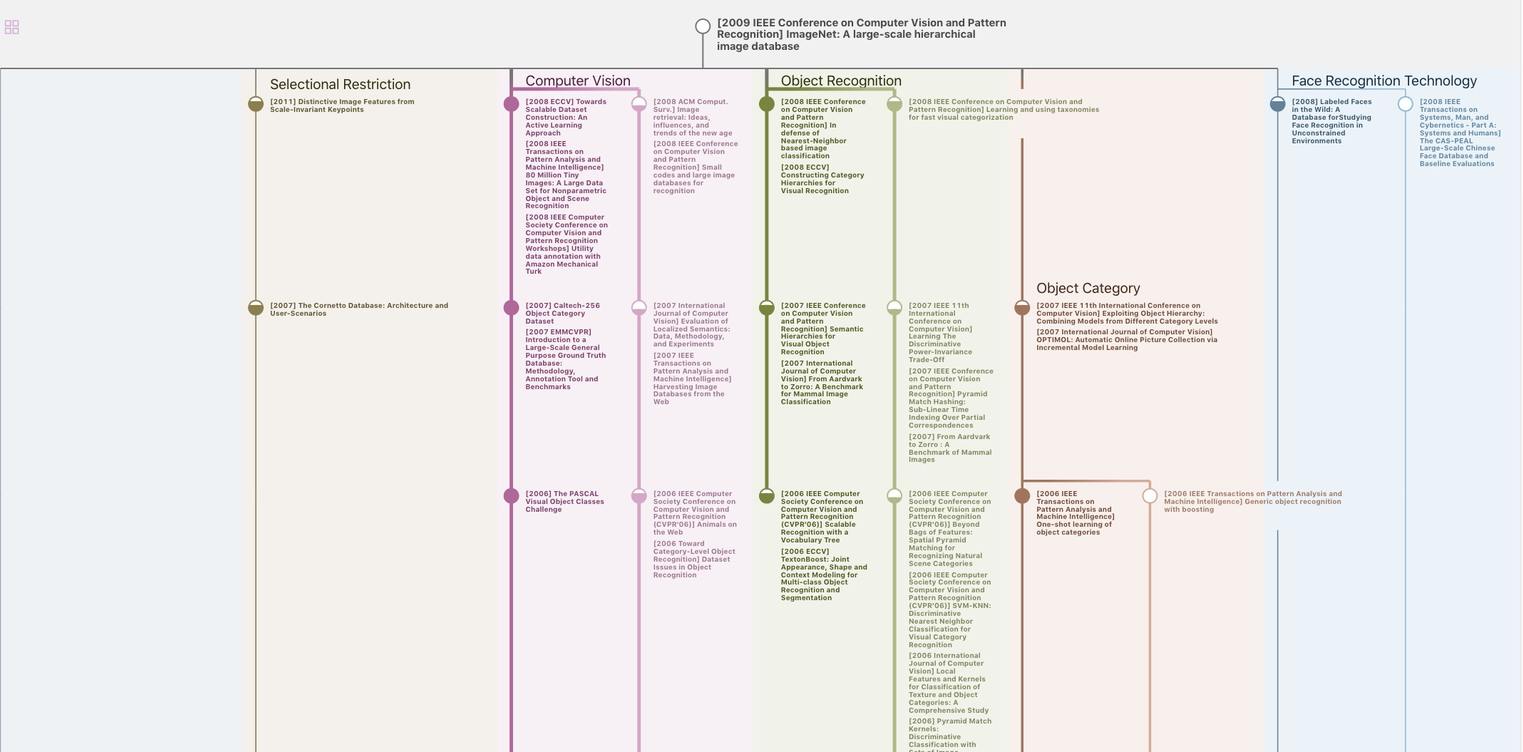
生成溯源树,研究论文发展脉络
Chat Paper
正在生成论文摘要