Efficient object localization using Convolutional Networks
2015 IEEE Conference on Computer Vision and Pattern Recognition (CVPR)(2015)
摘要
Recent state-of-the-art performance on human-body pose estimation has been achieved with Deep Convolutional Networks (ConvNets). Traditional ConvNet architectures include pooling and sub-sampling layers which reduce computational requirements, introduce invariance and prevent over-training. These benefits of pooling come at the cost of reduced localization accuracy. We introduce a novel architecture which includes an efficient ‘position refinement’ model that is trained to estimate the joint offset location within a small region of the image. This refinement model is jointly trained in cascade with a state-of-the-art ConvNet model [21] to achieve improved accuracy in human joint location estimation. We show that the variance of our detector approaches the variance of human annotations on the FLIC [20] dataset and outperforms all existing approaches on the MPII-human-pose dataset [1].
更多查看译文
关键词
object localization,human-body pose estimation,deep convolutional networks,ConvNet model,position refinement model,human joint offset location estimation,FLIC dataset,MPII-human-pose dataset
AI 理解论文
溯源树
样例
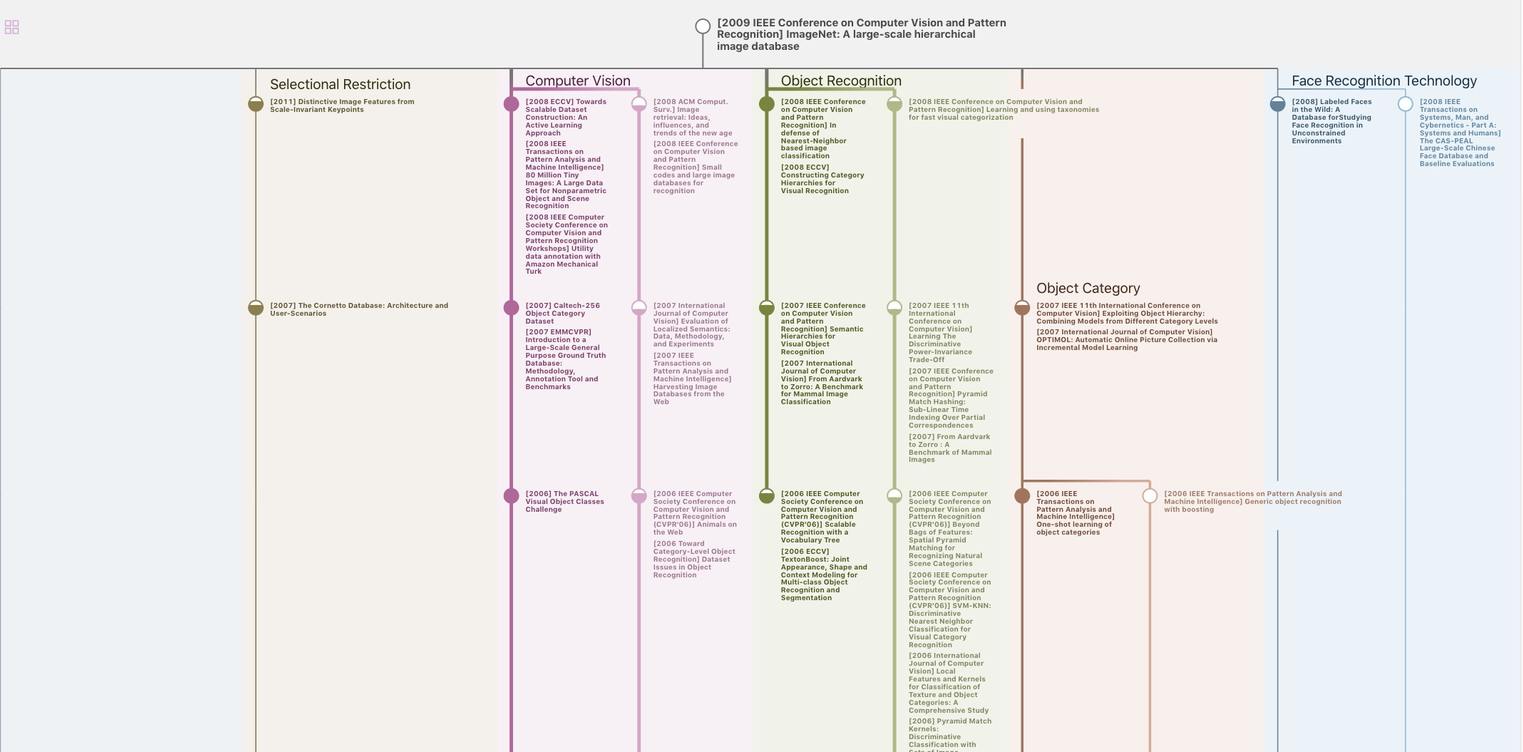
生成溯源树,研究论文发展脉络
Chat Paper
正在生成论文摘要