Ups and Downs: Modeling the Visual Evolution of Fashion Trends with One-Class Collaborative Filtering
WWW(2016)
摘要
Building a successful recommender system depends on understanding both the dimensions of people's preferences as well as their dynamics. In certain domains, such as fashion, modeling such preferences can be incredibly difficult, due to the need to simultaneously model the visual appearance of products as well as their evolution over time. The subtle semantics and non-linear dynamics of fashion evolution raise unique challenges especially considering the sparsity and large scale of the underlying datasets. In this paper we build novel models for the One-Class Collaborative Filtering setting, where our goal is to estimate users' fashion-aware personalized ranking functions based on their past feedback. To uncover the complex and evolving visual factors that people consider when evaluating products, our method combines high-level visual features extracted from a deep convolutional neural network, users' past feedback, as well as evolving trends within the community. Experimentally we evaluate our method on two large real-world datasets from Amazon.com, where we show it to outperform state-of-the-art personalized ranking measures, and also use it to visualize the high-level fashion trends across the 11-year span of our dataset.
更多查看译文
关键词
Recommender Systems,Fashion Evolution,Personalized Ranking,Visual Dimensions
AI 理解论文
溯源树
样例
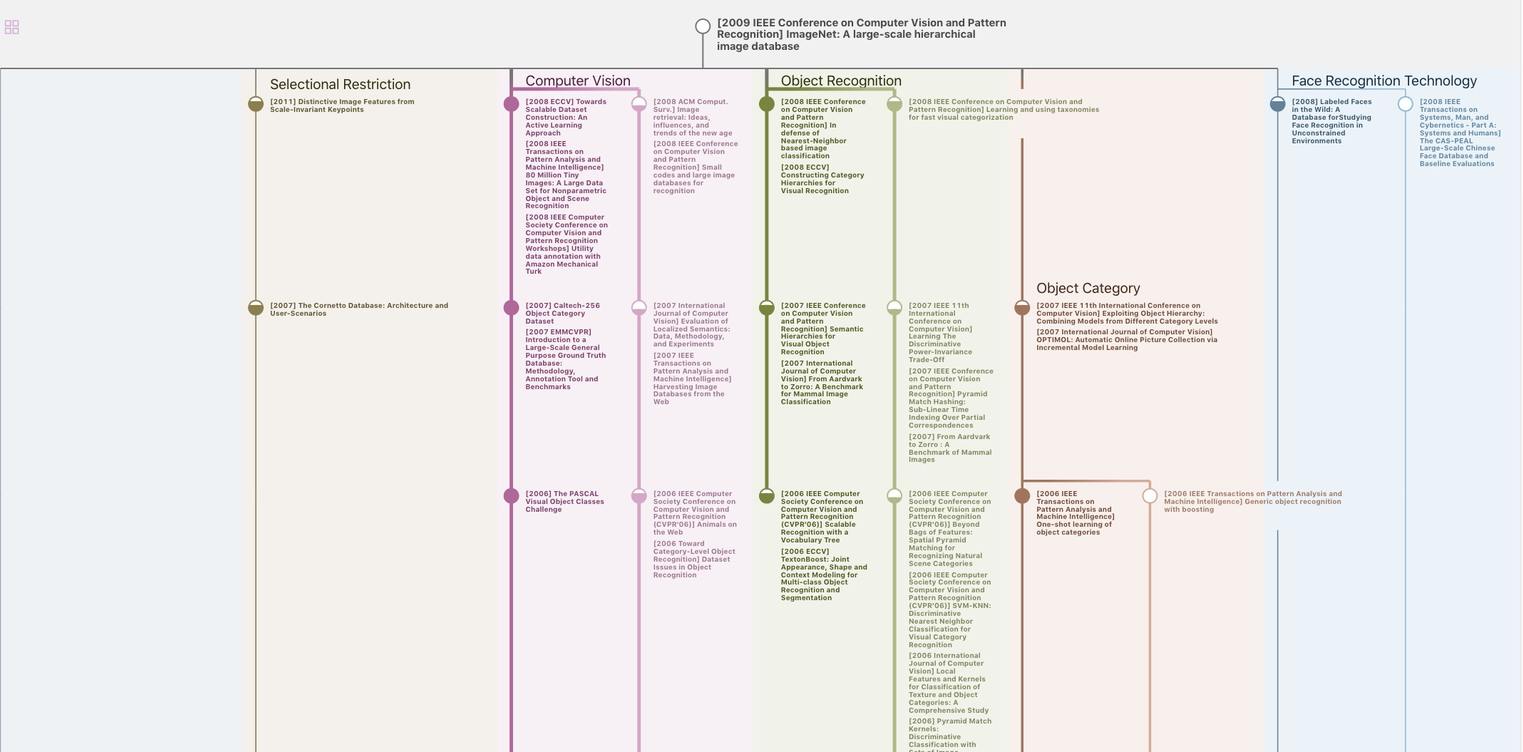
生成溯源树,研究论文发展脉络
Chat Paper
正在生成论文摘要