Effectively Creating Weakly Labeled Training Examples Via Approximate Domain Knowledge
ILP 2014: Revised Selected Papers of the 24th International Conference on Inductive Logic Programming - Volume 9046(2015)
摘要
One of the challenges to information extraction is the requirement of human annotated examples, commonly called gold-standard examples. Many successful approaches alleviate this problem by employing some form of distant supervision, i.e., look into knowledge bases such as Freebase as a source of supervision to create more examples. While this is perfectly reasonable, most distant supervision methods rely on a hand-coded background knowledge that explicitly looks for patterns in text. For example, they assume all sentences containing Person X and Person Y are positive examples of the relation married(X, Y). In this work, we take a different approach - we infer weakly supervised examples for relations from models learned by using knowledge outside the natural language task. We argue that this method creates more robust examples that are particularly useful when learning the entire information-extraction model (the structure and parameters). We demonstrate on three domains that this form of weak supervision yields superior results when learning structure compared to using distant supervision labels or a smaller set of gold-standard labels.
更多查看译文
关键词
Gold Standard Examples, Distant Supervision, Weak Supervision, Freebase, Markov Logic Networks (MLN)
AI 理解论文
溯源树
样例
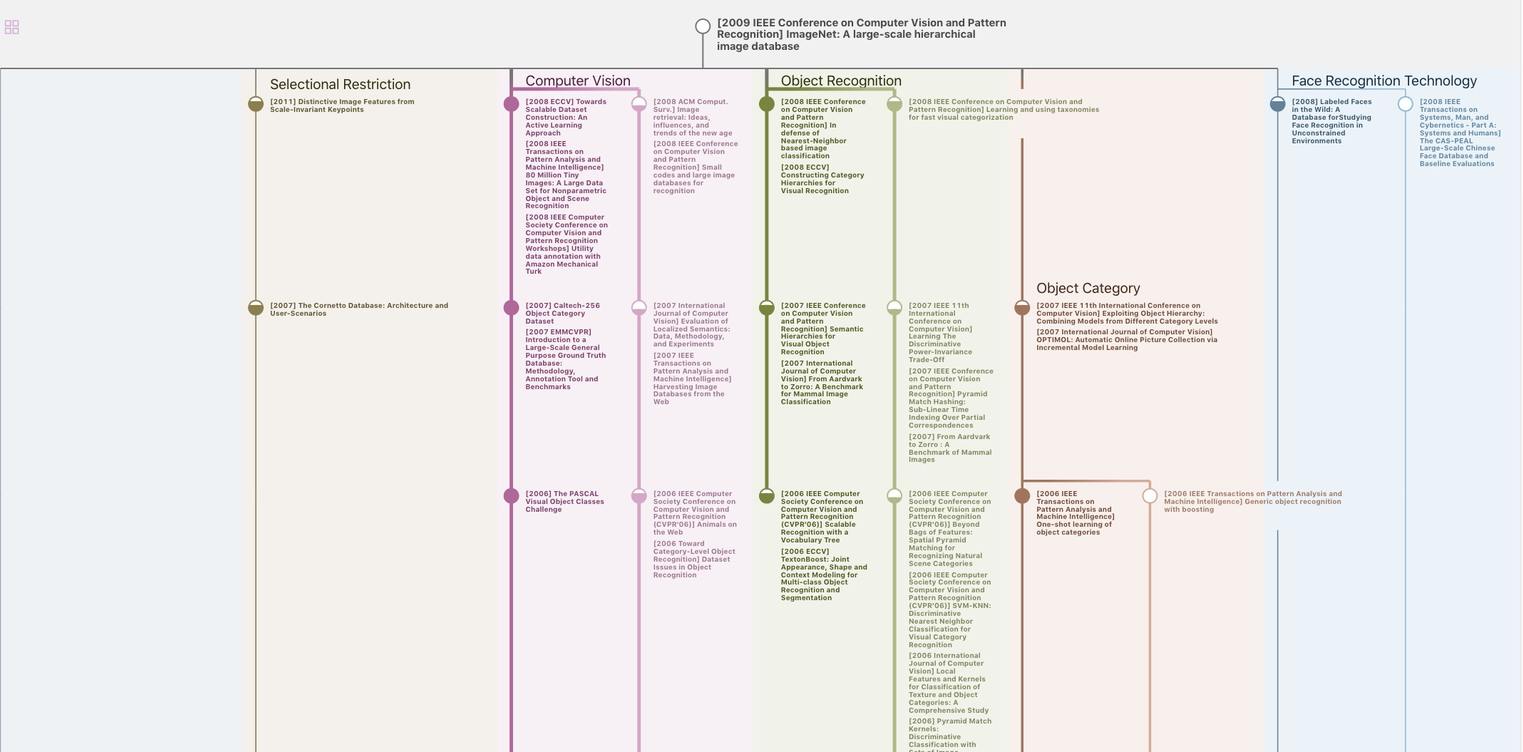
生成溯源树,研究论文发展脉络
Chat Paper
正在生成论文摘要