Modeling Spatial-Temporal Clues in a Hybrid Deep Learning Framework for Video Classification
MM(2015)
摘要
Classifying videos according to content semantics is an important problem with a wide range of applications. In this paper, we propose a hybrid deep learning framework for video classification, which is able to model static spatial information, short-term motion, as well as long-term temporal clues in the videos. Specifically, the spatial and the short-term motion features are extracted separately by two Convolutional Neural Networks (CNN). These two types of CNN-based features are then combined in a regularized feature fusion network for classification, which is able to learn and utilize feature relationships for improved performance. In addition, Long Short Term Memory (LSTM) networks are applied on top of the two features to further model longer-term temporal clues. The main contribution of this work is the hybrid learning framework that can model several important aspects of the video data. We also show that (1) combining the spatial and the short-term motion features in the regularized fusion network is better than direct classification and fusion using the CNN with a softmax layer, and (2) the sequence-based LSTM is highly complementary to the traditional classification strategy without considering the temporal frame orders. Extensive experiments are conducted on two popular and challenging benchmarks, the UCF-101 Human Actions and the Columbia Consumer Videos (CCV). On both benchmarks, our framework achieves to-date the best reported performance: $91.3\%$ on the UCF-101 and $83.5\%$ on the CCV.
更多查看译文
关键词
Video Classification,Deep Learning,CNN,LSTM,Fusion
AI 理解论文
溯源树
样例
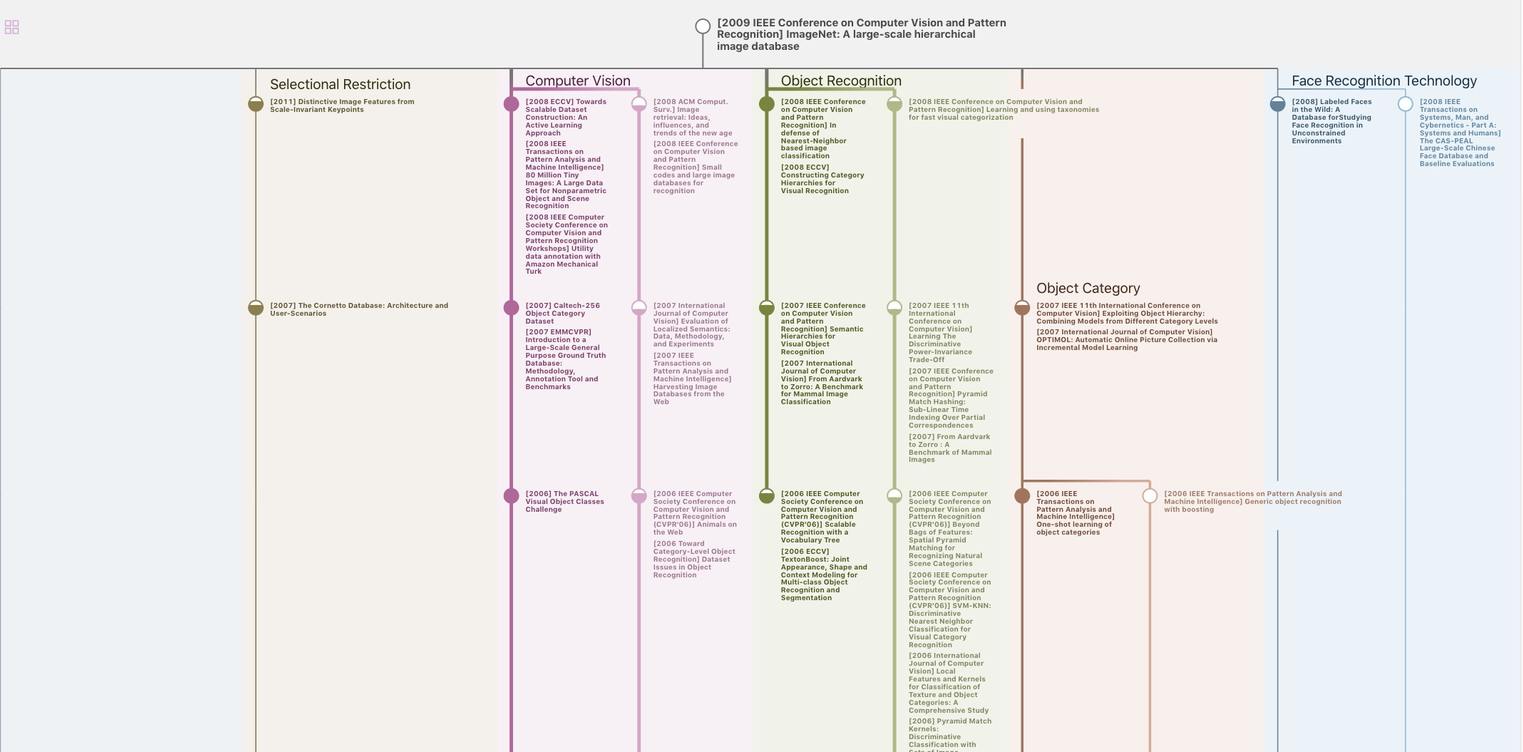
生成溯源树,研究论文发展脉络
Chat Paper
正在生成论文摘要