DRUM: A Dynamic Range Unbiased Multiplier for Approximate Applications
International Conference on Computer-Aided Design(2015)
摘要
Many applications for signal processing, computer vision and machine learning show an inherent tolerance to some computational error. This error resilience can be exploited to trade off accuracy for savings in power consumption and design area. Since multiplication is an essential arithmetic operation for these applications, in this paper we focus specifically on this operation and propose a novel approximate multiplier with a dynamic range selection scheme. We design the multiplier to have an unbiased error distribution, which leads to lower computational errors in real applications because errors cancel each other out, rather than accumulate, as the multiplier is used repeatedly for a computation. Our approximate multiplier design is also scalable, enabling designers to parameterize it depending on their accuracy and power targets. Furthermore, our multiplier benefits from a reduction in propagation delay, which enables its use on the critical path. We theoretically analyze the error of our design as a function of its parameters and evaluate its performance for a number of applications in image processing, and machine classification. We demonstrate that our design can achieve power savings of 54% -- 80%, while introducing bounded errors with a Gaussian distribution with near-zero average and standard deviations of 0.45% -- 3.61%. We also report power savings of up to 58% when using the proposed design in applications. We show that our design significantly outperforms other approximate multipliers recently proposed in the literature.
更多查看译文
关键词
DRUM,dynamic range unbiased multiplier,error resilience,power consumption savings,design area,arithmetic operation,approximate multiplier,dynamic range selection scheme,multiplier design,unbiased error distribution,computational errors,performance evaluation,parameter function,image processing,machine classification,bounded errors,Gaussian distribution,near-zero average deviations,near-zero standard deviations,power savings
AI 理解论文
溯源树
样例
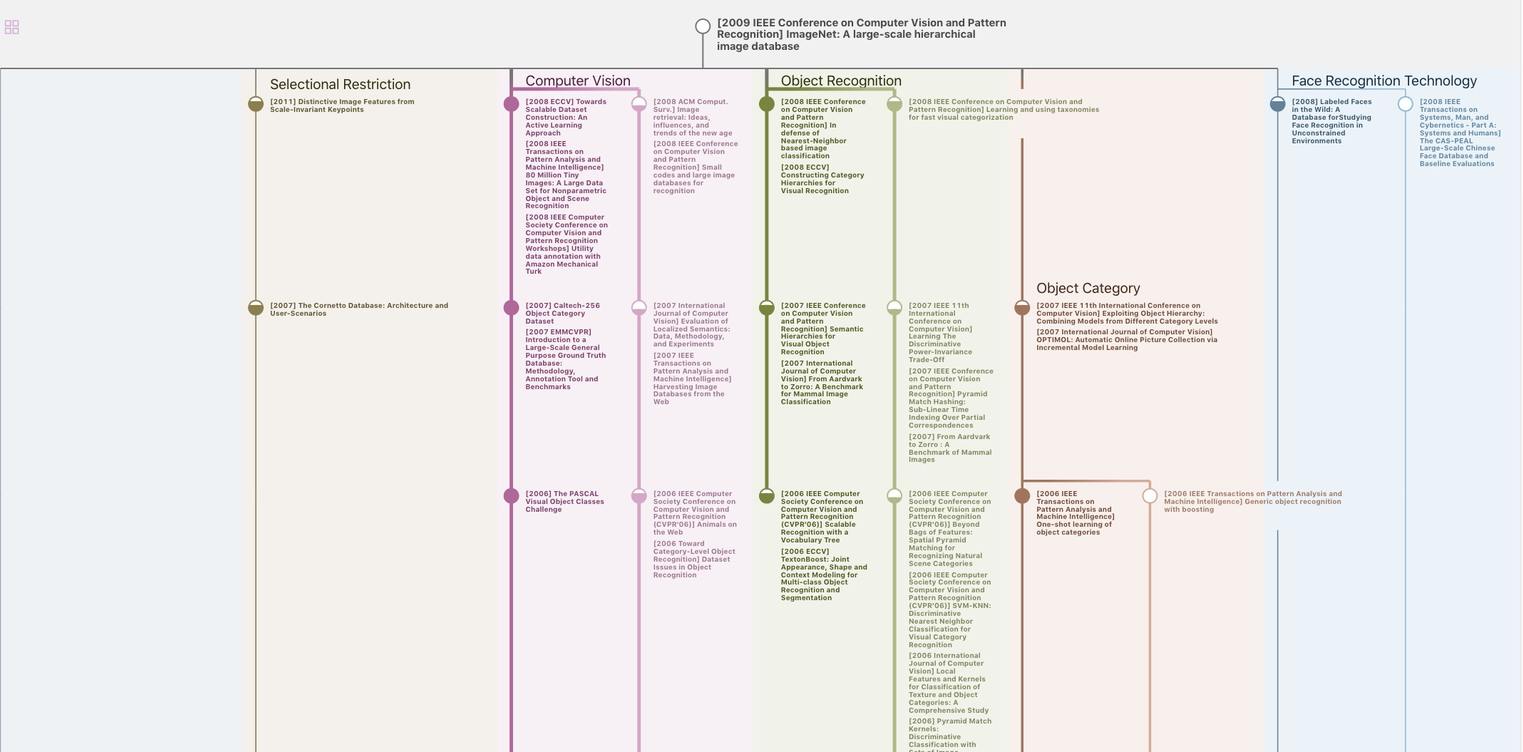
生成溯源树,研究论文发展脉络
Chat Paper
正在生成论文摘要