Weighted Filtering For Neural Activity Reconstruction Under Time Varying Constraints
ARTIFICIAL COMPUTATION IN BIOLOGY AND MEDICINE, PT I (IWINAC 2015)(2015)
摘要
A novel Weighted Unscented Kalman Filtering method is introduced for neural activity estimation from electroencephalographic signals. The introduction of a weighting stage improves the solution by extracting relevant information directly from the measured data. Besides, a discrete nonlinear state space model representing the brain neural activity is used as a physiological constraint in order to improve the estimation. Moreover, time-varying parameters are considered which allow describing adequately healthy and pathological activity even for localized epilepsy events. Performance of the new method is evaluated in terms of introduced error measurements by application to simulated EEG data over several noise conditions. As a result, a considerable improvement over linear estimation approaches is found.
更多查看译文
关键词
Inverse problem, Unscented kalman filtering, Relevance analysis
AI 理解论文
溯源树
样例
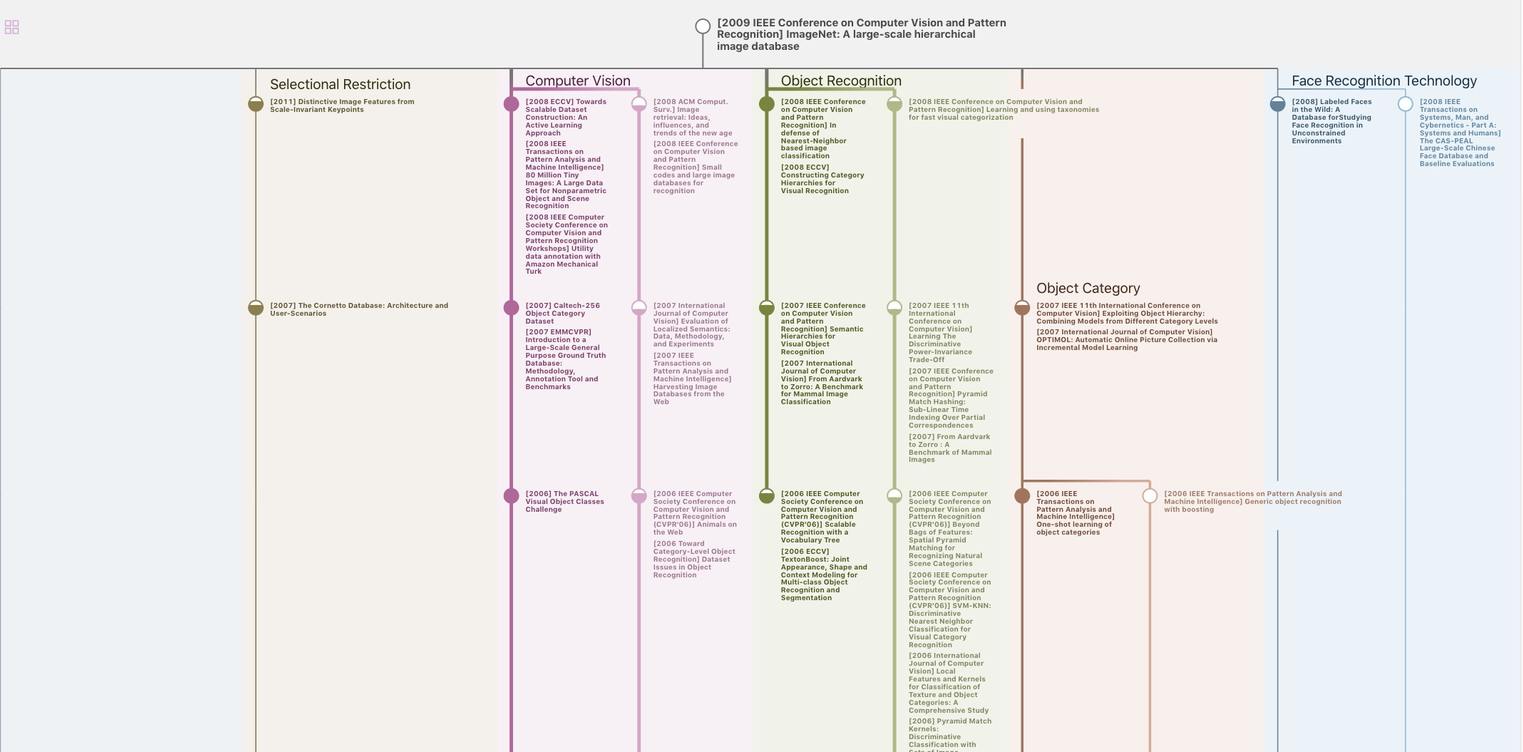
生成溯源树,研究论文发展脉络
Chat Paper
正在生成论文摘要